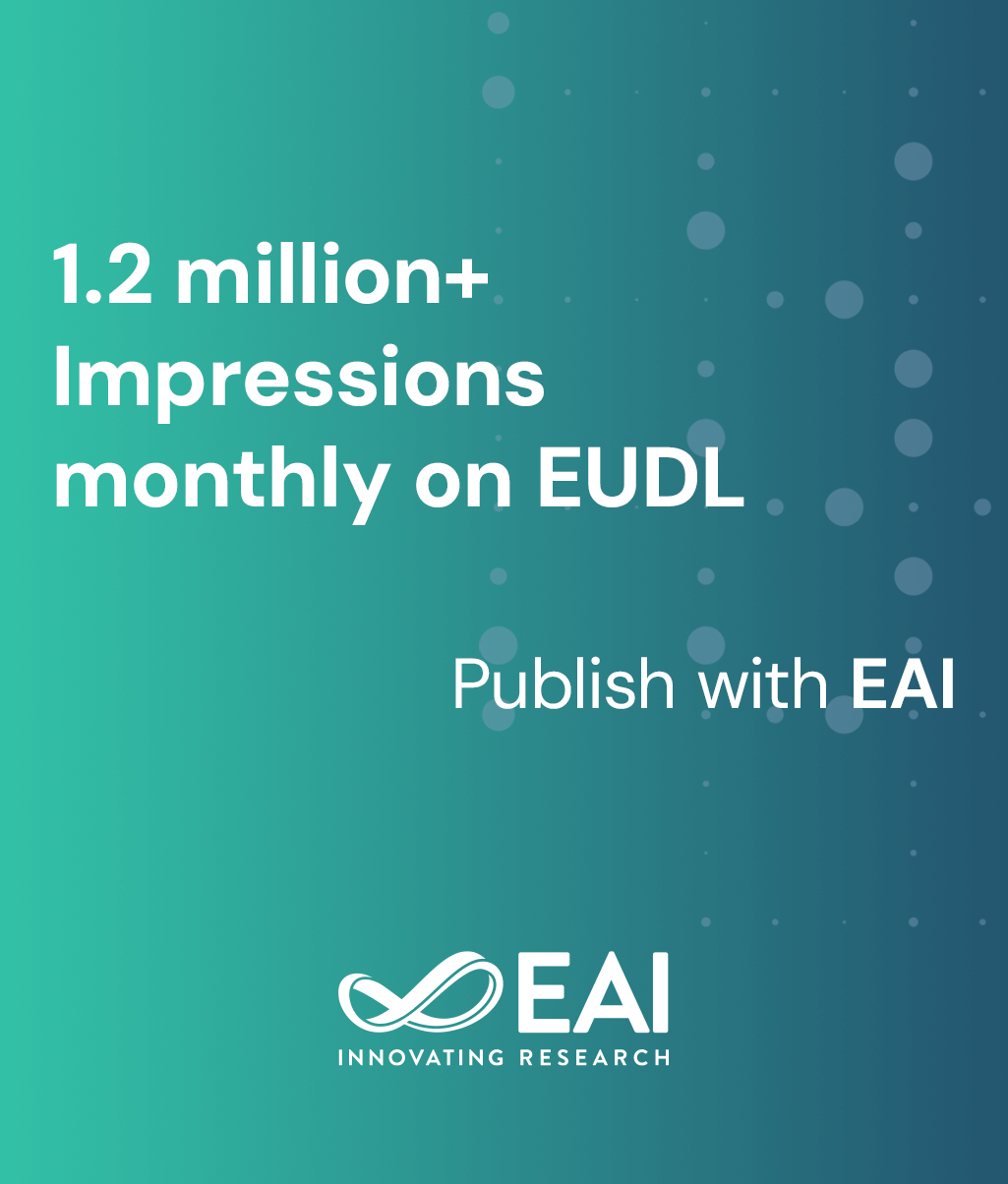
Research Article
A fireworks algorithm based Pi-Sigma neural network (FWA-PSNN) for modelling and forecasting chaotic crude oil price time series
@ARTICLE{10.4108/eai.13-7-2018.162803, author={Sarat Chandra Nayak}, title={A fireworks algorithm based Pi-Sigma neural network (FWA-PSNN) for modelling and forecasting chaotic crude oil price time series}, journal={EAI Endorsed Transactions on Energy Web}, volume={7}, number={28}, publisher={EAI}, journal_a={EW}, year={2020}, month={1}, keywords={crude oil price forecasting, time series forecasting, higher order neural network, pi-sigma neural network, fireworks algorithm, hybrid model, genetic algorithm}, doi={10.4108/eai.13-7-2018.162803} }
- Sarat Chandra Nayak
Year: 2020
A fireworks algorithm based Pi-Sigma neural network (FWA-PSNN) for modelling and forecasting chaotic crude oil price time series
EW
EAI
DOI: 10.4108/eai.13-7-2018.162803
Abstract
Capturing the complex correlations among the data on the crude oil price time series is challenging, hence accurate prediction of it is difficult. Contrast to multilayer artificial neural network, Pi-Sigma neural network (PSNN) is characterized with stronger approximation ability, fast learning and higher fault tolerance. Fireworks algorithm (FWA) is a new metaheuristic motivated from the occurrence of fireworks explosion, characterized with fast convergence capability, parallelism and finds global optima. This article intends to achieve the synergetic effect of both on hybridizing them. It uses FWA for optimization of weight and bias of PSNN (FWA-PSNN) and overcomes the limitations of gradient based training. It has single hidden layer structure and trainable weight, hence fast and robust. The FWA-PSNN is evaluated on prediction of crude oil price time series. Extensive simulation results, performance analysis, and statistical significance test suggested the suitability of FWA-PSNN.
Copyright © 2020 Sarat Chandra Nayak et al., licensed to EAI. This is an open access article distributed under the terms of the Creative Commons Attribution licence (http://creativecommons.org/licenses/by/3.0/), which permits unlimited use, distribution and reproduction in any medium so long as the original work is properly cited.