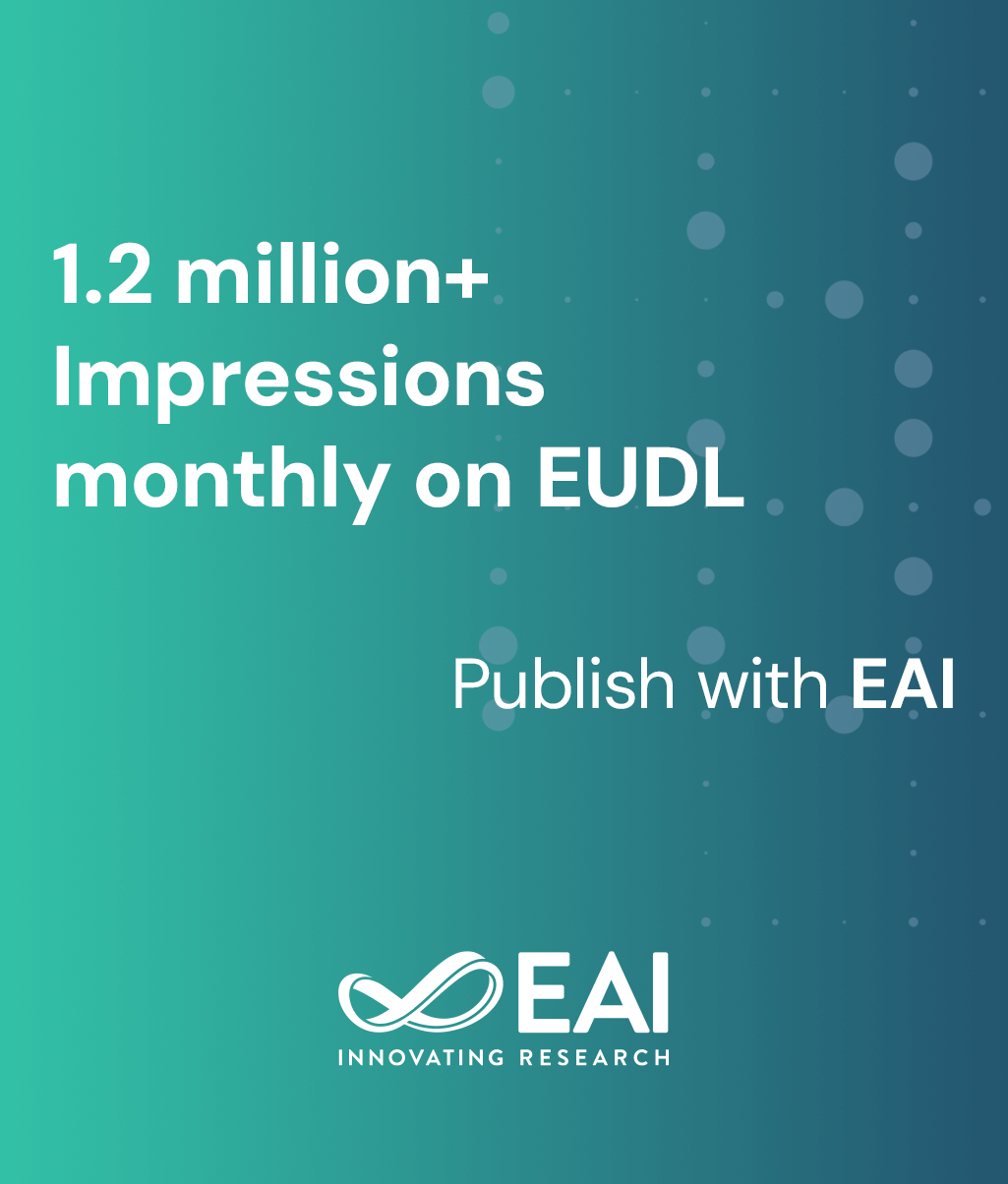
Research Article
A Competent and Novel Approach of Designing an Intelligent Image Retrieval System
@ARTICLE{10.4108/eai.13-7-2018.160538, author={Shefali Dhingra and Poonam Bansal}, title={A Competent and Novel Approach of Designing an Intelligent Image Retrieval System}, journal={EAI Endorsed Transactions on Scalable Information Systems}, volume={7}, number={24}, publisher={EAI}, journal_a={SIS}, year={2019}, month={9}, keywords={Content based Image retrieval (CBIR), Local Binary Pattern (LBP), Precision, Deep Learning Algorithm, Extreme learning Machine, Neural Networks}, doi={10.4108/eai.13-7-2018.160538} }
- Shefali Dhingra
Poonam Bansal
Year: 2019
A Competent and Novel Approach of Designing an Intelligent Image Retrieval System
SIS
EAI
DOI: 10.4108/eai.13-7-2018.160538
Abstract
A prominent technique used to search imperceptible images from any vast repository is denoted by Content based image retrieval (CBIR) system. The most eminent features of CBIR system are Texture, Color and Shape and in this paper these features are extracted using Local binary pattern, Color moment and Automatic segmentation process respectively. The features of these descriptors are combined for the formation of a hybrid feature vector by utilizing the process of normalization. Then, for enhancing the retrieval accuracy of the proposed system, Extreme learning machine (ELM) has been utilized as a classifier. The proposed hybrid CBIR system with ELM leads to an evolution of an intelligent system. Various evaluation metrics like Precision, Recall, Accuracy, etc. have been calculated on the proposed system on standard datasets. Average precision of 90%, 78%, 72% and 88.70% has been obtained respectively on Corel-1K, Corel-5K, Corel-10K and GHIM datasets which is significantly higher than the related techniques.
Copyright © 2019 Shefali Dhingra et al., licensed to EAI. This is an open access article distributed under the terms of the Creative Commons Attribution licence (http://creativecommons.org/licenses/by/3.0/), which permits unlimited use, distribution and reproduction in any medium so long as the original work is properly cited.