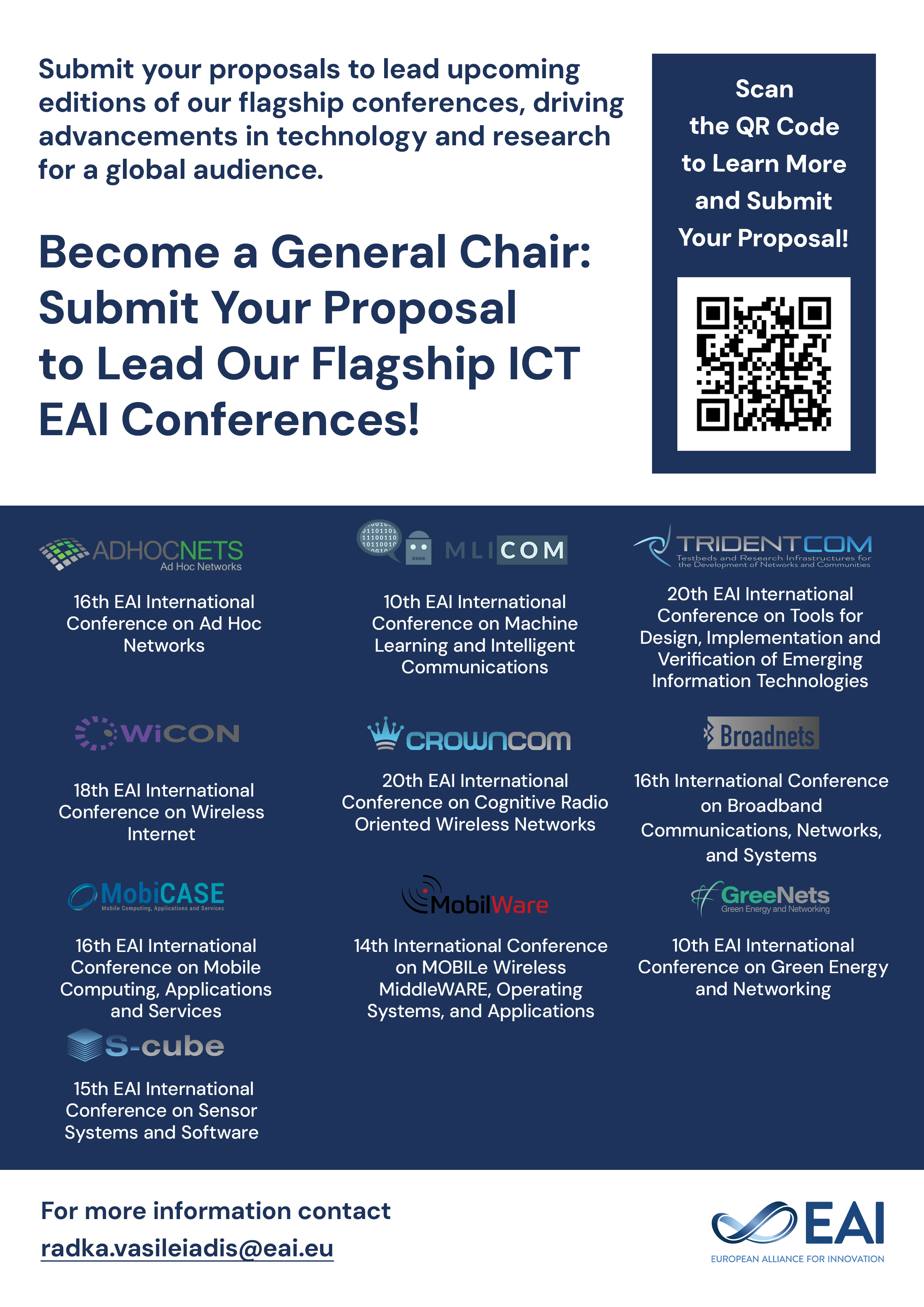
Research Article
Prediction of Cross Project Defects using Ensemble based Multinomial Classifier
@ARTICLE{10.4108/eai.13-7-2018.159974, author={Lipika Goel and Mayank Sharma and Sunil Kumar Khatri and D. Damodaran}, title={Prediction of Cross Project Defects using Ensemble based Multinomial Classifier}, journal={EAI Endorsed Transactions on Scalable Information Systems}, volume={7}, number={25}, publisher={EAI}, journal_a={SIS}, year={2019}, month={9}, keywords={Defect Prediction, CPDP (Cross Project defect prediction), WPDP (Within Project defect prediction), Ensemble learning, Multinomial classification, Homogeneous metrics}, doi={10.4108/eai.13-7-2018.159974} }
- Lipika Goel
Mayank Sharma
Sunil Kumar Khatri
D. Damodaran
Year: 2019
Prediction of Cross Project Defects using Ensemble based Multinomial Classifier
SIS
EAI
DOI: 10.4108/eai.13-7-2018.159974
Abstract
BACKGROUND: The availability of defect related data of different projects leads to cross project defect prediction an open issue. Many studies have focused on analyzing and improving the performance of Cross project defect prediction.
OBJECTIVE: The multinomial classification has not been much explored. This paper instanced on multiclass/multinomial classification of defect prediction of cross projects.
METHOD: The ensemble based statistical models – Gradient Boosting and Random Forest are used for classification. An empirical study is carried out to determine the performance of multinomial classification for cross project defect prediction. Depending on the number of defects, class level information is classified into one of three defined multiclass class 0, class 1, and class 2.
RESULTS & CONCLUSION: Major outcome of the paper concludes that multinomial/multiclass classification is applicable on cross project data and has comparable results to within project defect data.
Copyright © 2019 Lipika Goel, licensed to EAI. This is an open access article distributed under the terms of the Creative Commons Attribution licence (http://creativecommons.org/licenses/by/3.0/), which permits unlimited use, distribution and reproduction in any medium so long as the original work is properly cited.