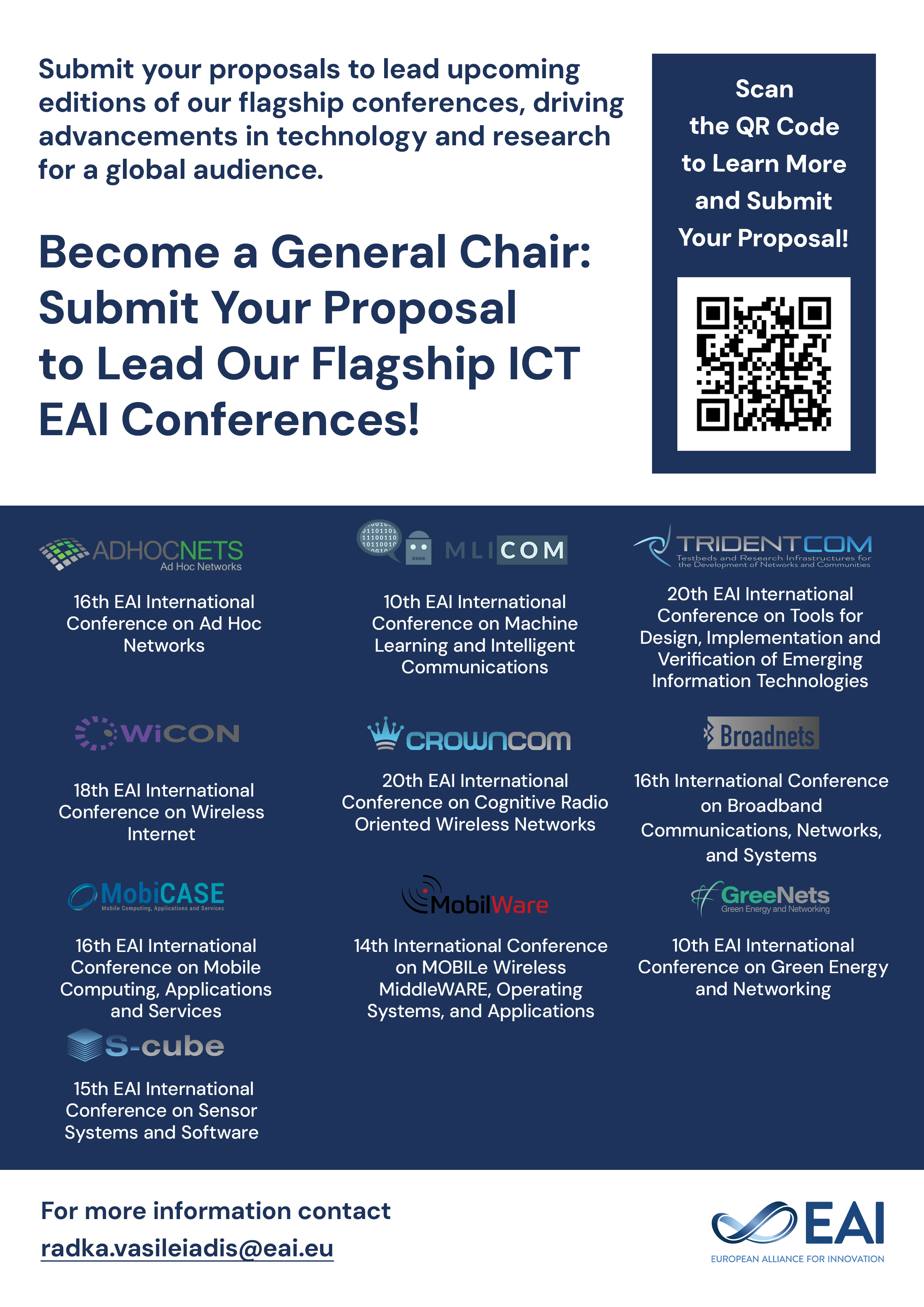
Research Article
Email Phishing: An Enhanced Classification Model to Detect Malicious URLs
@ARTICLE{10.4108/eai.13-7-2018.158529, author={Shweta Sankhwar and Dhirendra Pandey and R.A Khan}, title={Email Phishing: An Enhanced Classification Model to Detect Malicious URLs}, journal={EAI Endorsed Transactions on Scalable Information Systems}, volume={6}, number={21}, publisher={EAI}, journal_a={SIS}, year={2019}, month={5}, keywords={Email, Phishing, Machine Learning Techniques, Information security, Cybercrime}, doi={10.4108/eai.13-7-2018.158529} }
- Shweta Sankhwar
Dhirendra Pandey
R.A Khan
Year: 2019
Email Phishing: An Enhanced Classification Model to Detect Malicious URLs
SIS
EAI
DOI: 10.4108/eai.13-7-2018.158529
Abstract
Phishing is the process of enticing people into visiting fraudulent websites and persuading them to enter their personal information. Number in phishing email are spread with the aim of making web users believe that they are communicating with a trusted entity or organization. Phishing is deployed by the use of advanced and harmful tactics like malicious or phishing URLs. So, it becomes necessary to detect malicious or phishing URLs in the present scenario. Numerous anti- phishing techniques are in vogue to discriminate fake and the authentic website but are not effective. This research, focuses on the relevant URLs features that discriminate between legitimate and malicious/phishing URLs. The impact of email phishing can be largely reduced by adopting an appropriate combination of all these features with classification techniques. Therefore, an Enhanced Malicious URLs Detection (EMUD) model is developed with machine learning techniques for better classification and accurate results.
Copyright © 2019 Shweta Sankhwar et al., licensed to EAI. This is an open access article distributed under the terms of the Creative Commons Attribution licence (http://creativecommons.org/licenses/by/3.0/), which permits unlimited use, distribution and reproduction in any medium so long as the original work is properly cited.