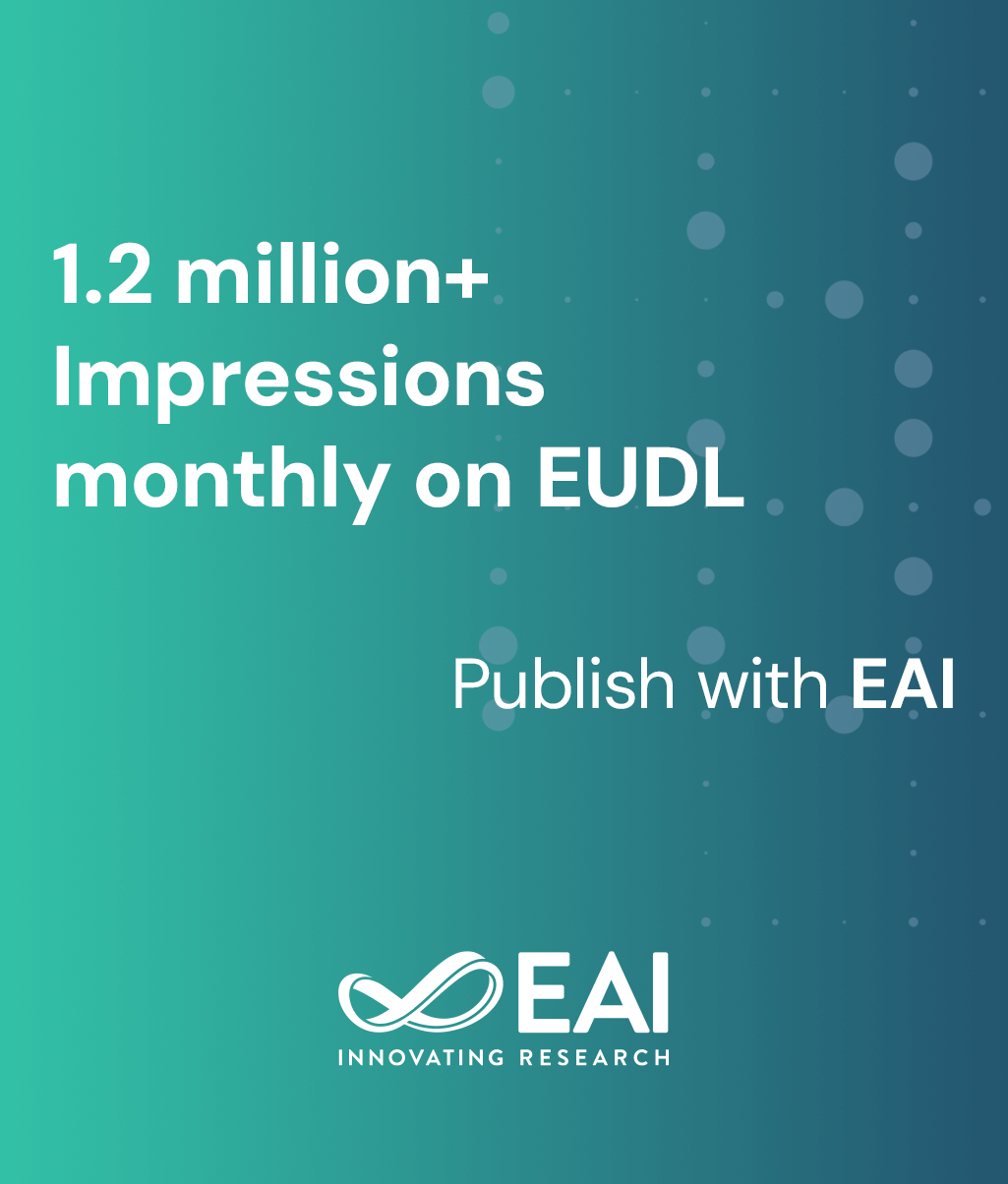
Research Article
Formal Approach to Detect and Resolve Anomalies while Clustering ABAC Policies
@ARTICLE{10.4108/eai.13-7-2018.156003, author={Maryem Ait El Hadj and Ahmed Khoumsi and Yahya Benkaouz and Mohammed Erradi}, title={Formal Approach to Detect and Resolve Anomalies while Clustering ABAC Policies}, journal={EAI Endorsed Transactions on Security and Safety}, volume={5}, number={16}, publisher={EAI}, journal_a={SESA}, year={2018}, month={12}, keywords={ABAC Policies, Clustering, Access Domain, Conflict, Redundancy, Detection and Resolution, Permissive Resolution, Restrictive Resolution}, doi={10.4108/eai.13-7-2018.156003} }
- Maryem Ait El Hadj
Ahmed Khoumsi
Yahya Benkaouz
Mohammed Erradi
Year: 2018
Formal Approach to Detect and Resolve Anomalies while Clustering ABAC Policies
SESA
EAI
DOI: 10.4108/eai.13-7-2018.156003
Abstract
In big data environments with big number of users and high volume of data, we need to manage the corresponding huge number of security policies. Using Attribute-Based Access Control (ABAC) model to ensure access control might become complex and hard to manage. Moreover, ABAC policies may be aggregated from multiple parties. Therefore, they may contain several anomalies such as conflicts and redundancies, resulting in safety and availability problems. Several policy analysis and design methods have been proposed. However, most of these methods do not preserve the original policy semantics. In this paper, we present an ABAC anomaly detection and resolution method based on the access domain concept, while preserving the policy semantics. To make the suggested method scalable for large policies, we decompose the policy into clusters of rules, then the method is applied to each cluster. We prove correctness of the method and evaluate its computational complexity. Experimental results are given and discussed.
Copyright © 2018 Maryem Ait El Hadj et al., licensed to EAI. This is an open access article distributed under the terms of the Creative Commons Attribution license (http://creativecommons.org/licenses/by/3.0/), which permits unlimited use, distribution and reproduction in any medium so long as the original work is properly cited.