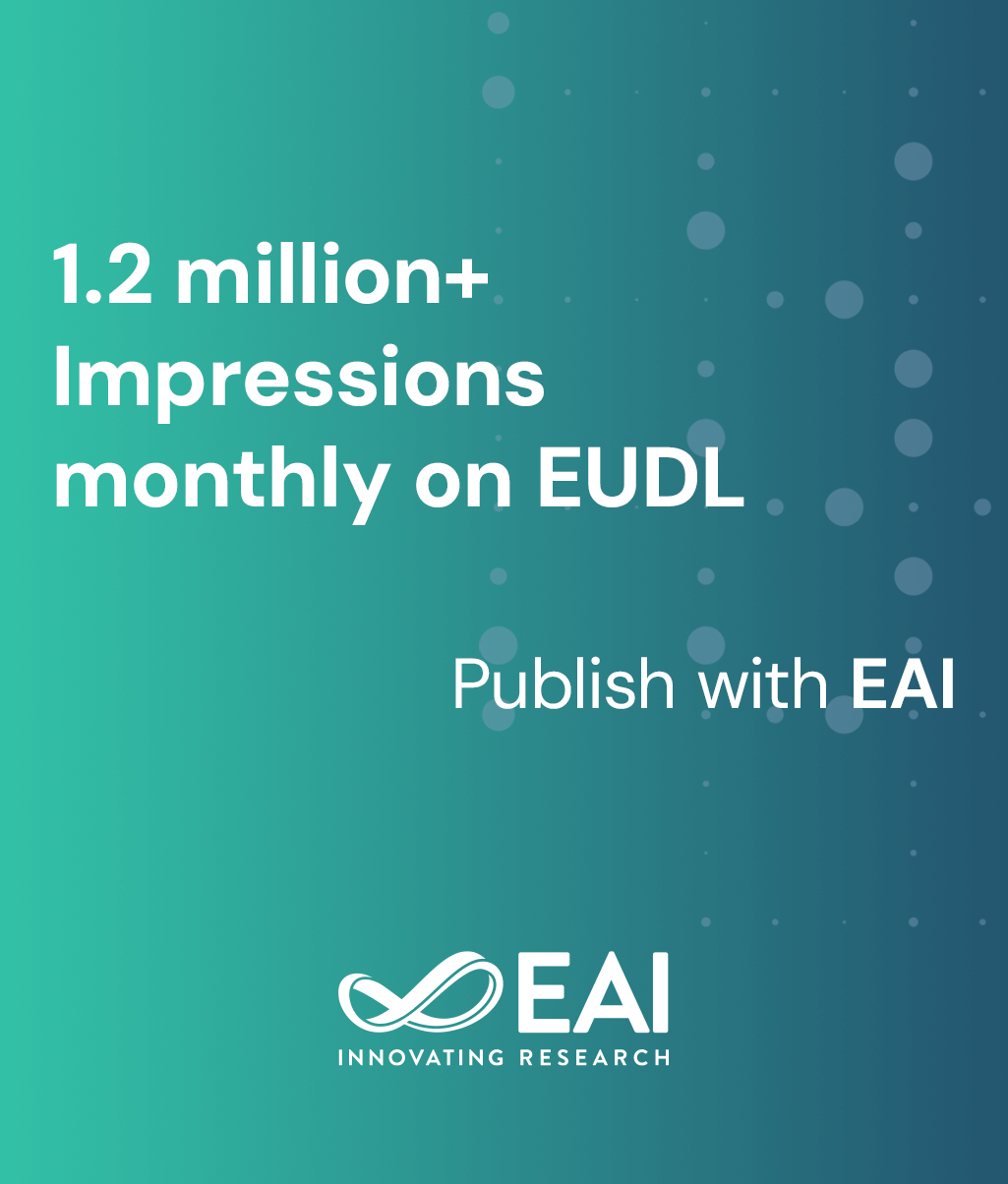
Research Article
Masked Loss Residual Convolutional Neural Network For Facial Keypoint Detection
@INPROCEEDINGS{10.4108/eai.13-7-2017.2270651, author={Junhong Xu and Shaoen Wu and Shangyue Zhu and Hangqing Guo and Honggang Wang and QIng Yang}, title={Masked Loss Residual Convolutional Neural Network For Facial Keypoint Detection}, proceedings={10th EAI International Conference on Mobile Multimedia Communications}, publisher={EAI}, proceedings_a={MOBIMEDIA}, year={2017}, month={12}, keywords={facial keypoint detection cnn deep learning}, doi={10.4108/eai.13-7-2017.2270651} }
- Junhong Xu
Shaoen Wu
Shangyue Zhu
Hangqing Guo
Honggang Wang
QIng Yang
Year: 2017
Masked Loss Residual Convolutional Neural Network For Facial Keypoint Detection
MOBIMEDIA
EAI
DOI: 10.4108/eai.13-7-2017.2270651
Abstract
This paper presents an e ective and novel CNN-based deep learning solution, named Masked Loss Residual Convolutional Neural Net- work (ML-ResNet), to facial keypoint detection on the datasets that have missing target labels. The core of the ML-ResNet solution is a masked loss objective function that ignores the error in predicting the missing target keypoints in the output layer of a CNN. To com- pensate for the loss induced by the masked loss objective function to prevent over tting, we design a data augmentation strategy in ML-ResNet to increase the number of training data. The perfor- mance of ML-ResNet has been evaluated on the image dataset from Kaggle Facial Keypoints Detection competition, which consists of 7,049 training images, but with only 2,140 images that have full tar- get keypoints labeled. In the experiments, ML-ResNet is compared to a pioneer literature CNN facial keypoint detection work. The experiment results clearly show that the proposed ML-ResNet is robust and advantageous in training CNNs on datasets that have missing target values. ML-ResNet can improve the learning time by 30% during the training and the detection accuracy by eight times in facial keypoint detection.