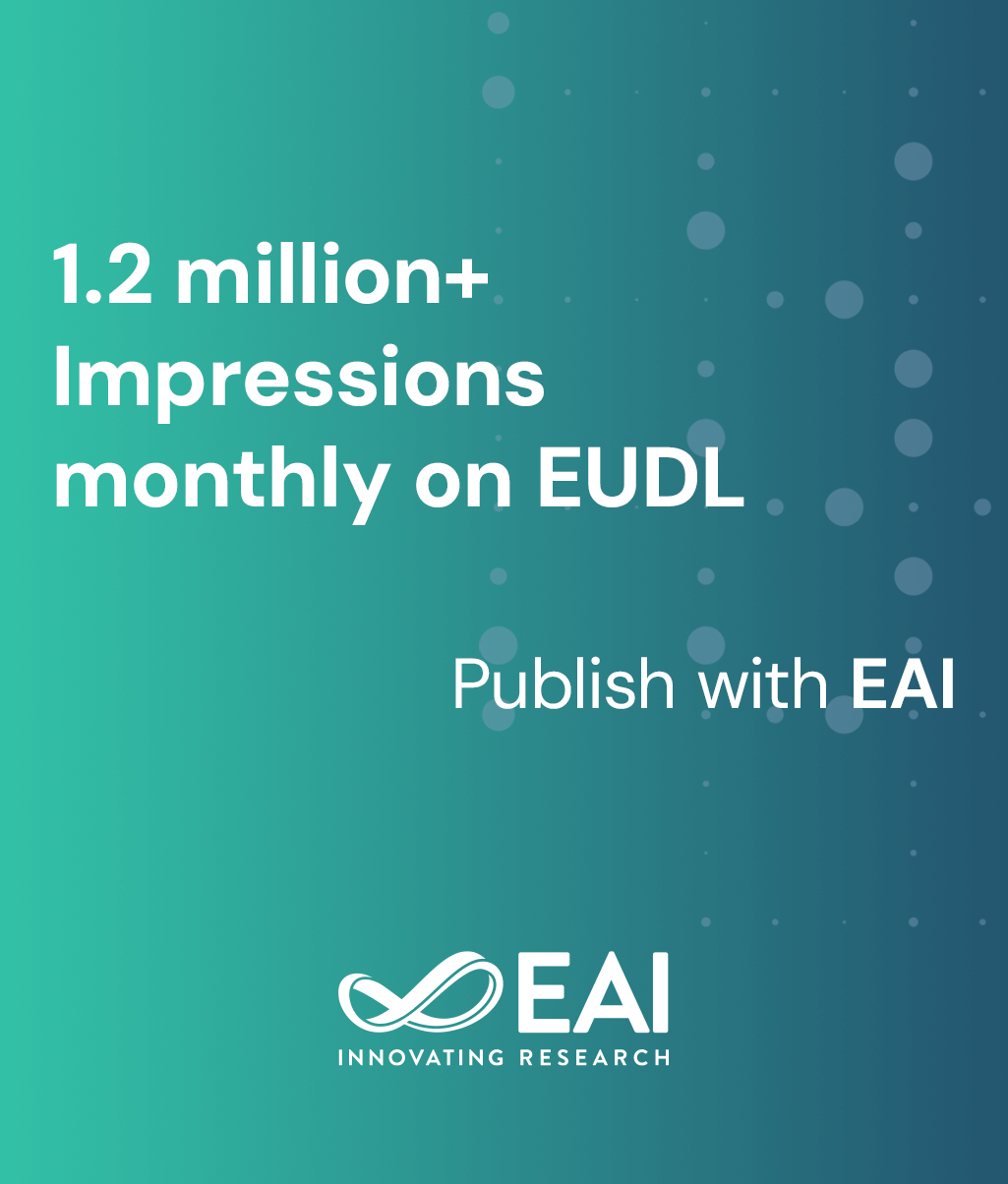
Research Article
Centrality-Based Paper Citation Recommender System
@ARTICLE{10.4108/eai.13-6-2019.159121, author={Abdul Samad and Muhammad Arshad Islam and Muhammad Azhar Iqbal and Muhammad Aleem}, title={Centrality-Based Paper Citation Recommender System}, journal={EAI Endorsed Transactions on Industrial Networks and Intelligent Systems}, volume={6}, number={19}, publisher={EAI}, journal_a={INIS}, year={2019}, month={6}, keywords={Citation Recommendation, Textual Similarity, Topological Similarity}, doi={10.4108/eai.13-6-2019.159121} }
- Abdul Samad
Muhammad Arshad Islam
Muhammad Azhar Iqbal
Muhammad Aleem
Year: 2019
Centrality-Based Paper Citation Recommender System
INIS
EAI
DOI: 10.4108/eai.13-6-2019.159121
Abstract
Researchers cite papers in order to connect the new research ideas with previous research. For the purpose of finding suitable papers to cite, researchers spend a considerable amount of time and effort. To help researchers in finding relevant/important papers, we evaluated textual and topological similarity measures for citation recommendations. This work analyzes textual and topological similarity measures (i.e., Jaccard and Cosine) to evaluate which one performs well in finding similar papers? To find the importance of papers, we compute centrality measures (i.e., Betweeness, Closeness, Degree and PageRank). After evaluation, it is found that topological-based similarity via Cosine achieved 85.2% and using Jaccard obtained 61.9% whereas textualbased similarity via Cosine on abstract obtained 68.9% and using Cosine on title achieved 37.4% citation links. Likewise, textual-based similarity via Jaccard on abstract obtained 35.4% and using Jaccard on title achieved 28.3% citation links.
Copyright © 2019 Abdul Samad et al., licensed to EAI. This is an open access article distributed under the terms of the Creative Commons Attribution license (http://creativecommons.org/licenses/by/3.0/), which permits unlimited use, distribution and reproduction in any medium so long as the original work is properly cited.