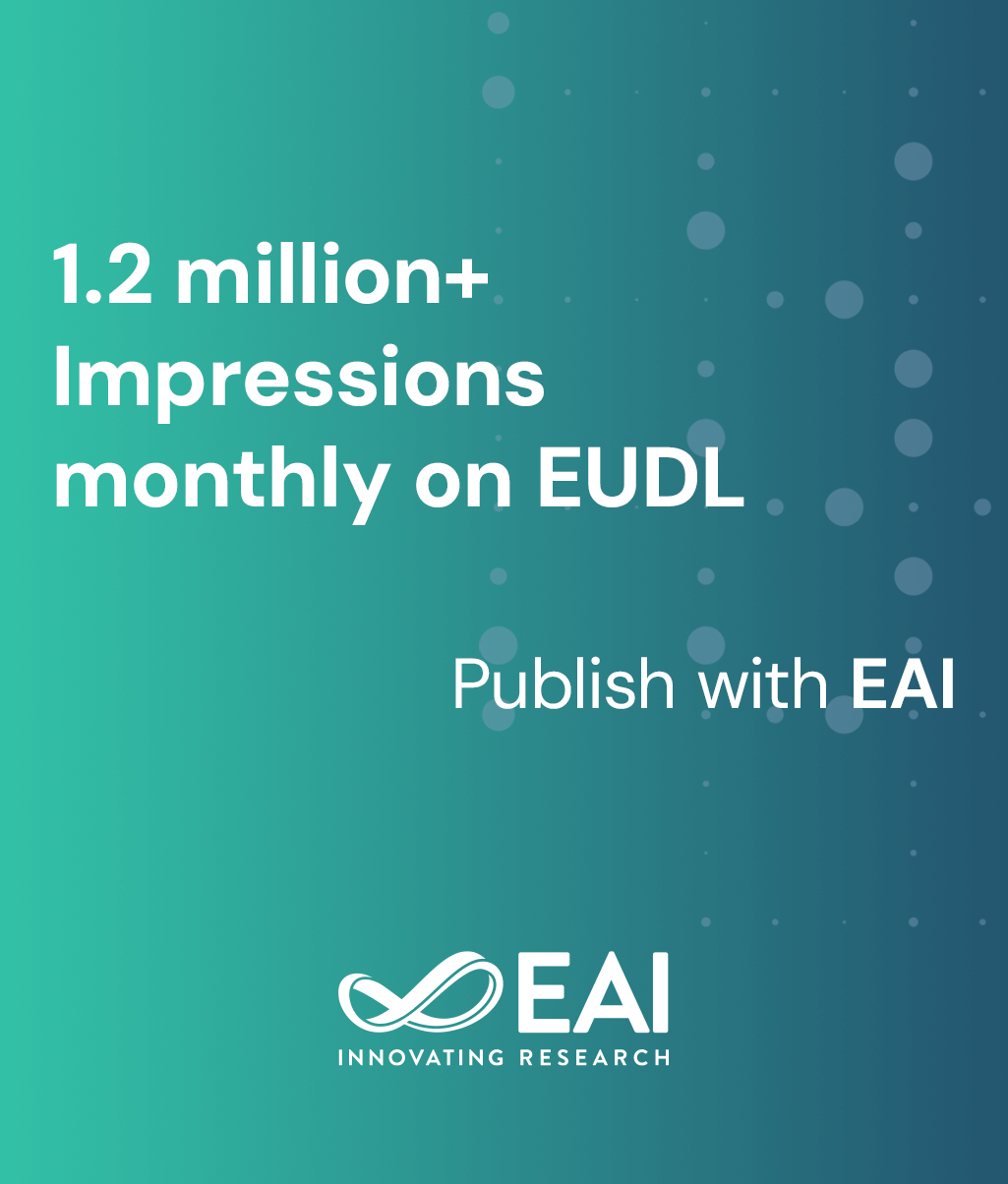
Research Article
Implementation of Fuzzy Cognitive Map and Support Vector Machine for Classification of Oral Cancers
@ARTICLE{10.4108/eai.12-9-2018.155559, author={Anuradha K and Uma K P}, title={Implementation of Fuzzy Cognitive Map and Support Vector Machine for Classification of Oral Cancers}, journal={EAI Endorsed Transactions on Energy Web and Information Technologies}, volume={5}, number={20}, publisher={EAI}, journal_a={EW}, year={2018}, month={9}, keywords={Oral cancer, Fuzzy Cognitive Map, Histological Features, Support Vector Machine}, doi={10.4108/eai.12-9-2018.155559} }
- Anuradha K
Uma K P
Year: 2018
Implementation of Fuzzy Cognitive Map and Support Vector Machine for Classification of Oral Cancers
EW
EAI
DOI: 10.4108/eai.12-9-2018.155559
Abstract
Objective: Tumors at any stage may progress into cancer. Till now, cancer classificationis a challenging task for the researchers. Research on the cancer biology starts with the changes in the tissues. Oral cancer is a malignant cell growth in the oral cavity. Method: The proposed work combines Fuzzy Cognitive map (FCM) with Support Vector Machine (SVM) for grading oral tumor. The histological features are used as concepts and the interrelationships between the concepts are identified. FCM acts as a classifier to distinguish between benign and malignant cases. Further, the extracted output from FCM is fed as an input to the Support Vector Machine (SVM) classifier. This will improve the prediction capabilities. Result: The classification accuracy obtained for the proposed model is 92.10% for malignant cases and 94.11% for benign cases. Conclusion: The experimental results show that the combination of FCM with SVM obtained a good result when compared to the hybrid model using FCM.
Copyright © Anuradha.K et al., licensed to EAI. This is an open access article distributed under the terms of the Creative Commons Attribution licence (http://creativecommons.org/licenses/by/3.0/), which permits unlimited use, distribution and reproduction in any medium so long as the original work is properly cited.