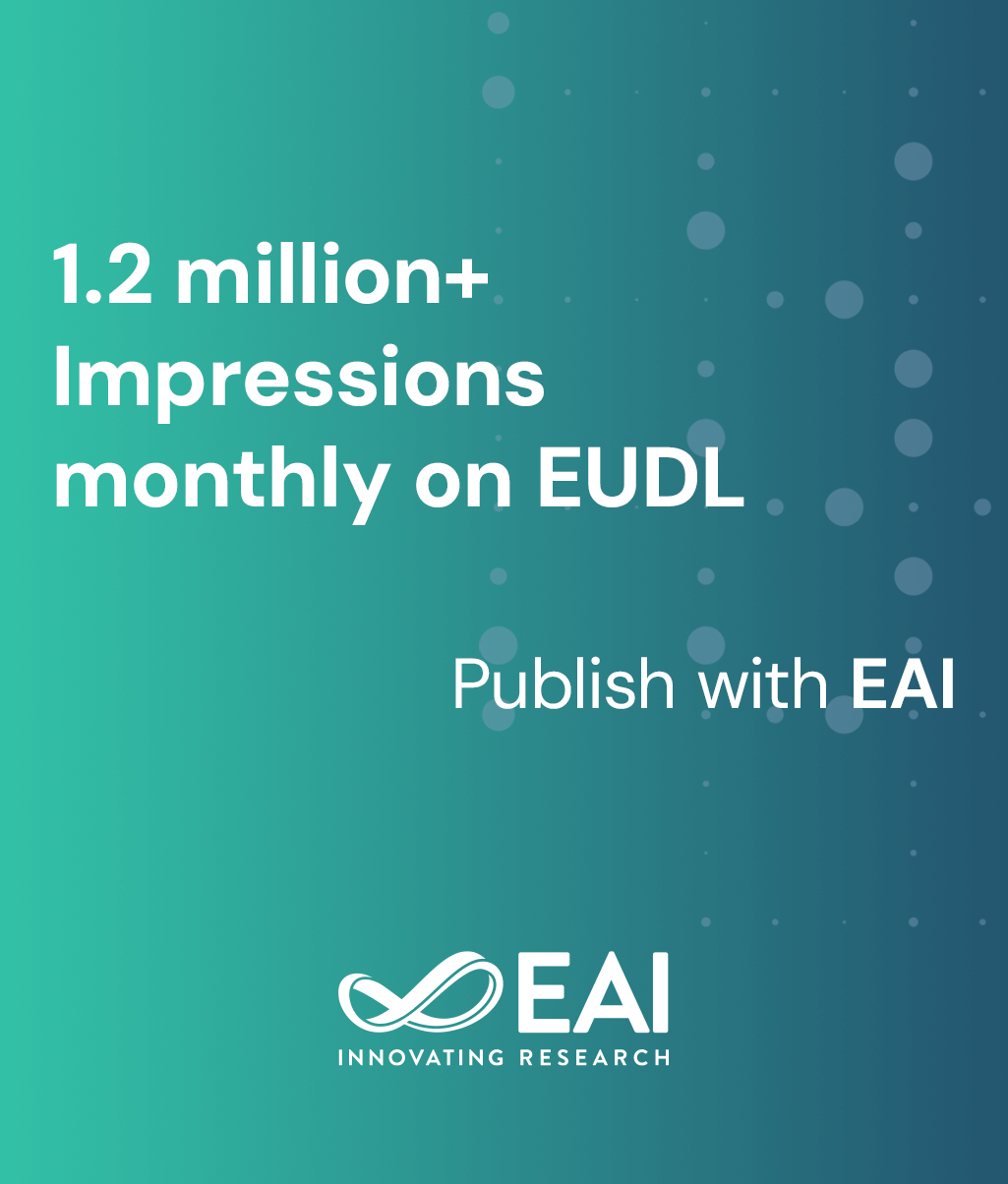
Research Article
Aspects of Mechanism Design for Industry 4.0 Multi-Robot Task Auctioning
@ARTICLE{10.4108/eai.12-8-2021.170670, author={Ajay Kattepur and Sourav Khemkha}, title={Aspects of Mechanism Design for Industry 4.0 Multi-Robot Task Auctioning}, journal={EAI Endorsed Transactions on Smart Cities}, volume={6}, number={17}, publisher={EAI}, journal_a={SC}, year={2021}, month={8}, keywords={Smart Things and Smart Objects, Software Defined Networks and Internet of Things, Smart Homes, Buildings and Malls}, doi={10.4108/eai.12-8-2021.170670} }
- Ajay Kattepur
Sourav Khemkha
Year: 2021
Aspects of Mechanism Design for Industry 4.0 Multi-Robot Task Auctioning
SC
EAI
DOI: 10.4108/eai.12-8-2021.170670
Abstract
Collaborative multi-robotic tasks are essential in complex Industry 4.0 deployments, involving autonomous robots. As such autonomous robots work with minimal centralized control, analysis of strategies for effective cooperation in task completion are needed. This is specially crucial when automation tasks are outsourced to specialized vendors without centralized control. Mechanism Design techniques have been proposed to create scenarios among multiple autonomous (possibly selfish) entities to result in desired outcomes. In this paper, we study the effect of varying bidding auction mechanism design protocols to result in multi-agent task auctioning. We demonstrate this approach over a realistic use case of task allocation involving multiple pick and place robots in Industry 4.0 warehouses. Multiple realistic auction/bidding scenarios considered including selfish agents, erroneous estimates of temporal features, heterogeneous capacities and composite bids. The results demonstrate that effective mechanisms can lead to fair outcomes despite erroneous or biased bids for tasks from agents.
Copyright ©2021 Ajay Kattepur et al., licensed to EAI. This is an open access article distributed under the terms of the Creative Commons Attribution license, which permits unlimited use, distribution and reproduction in any medium so long as the original work is properly cited.