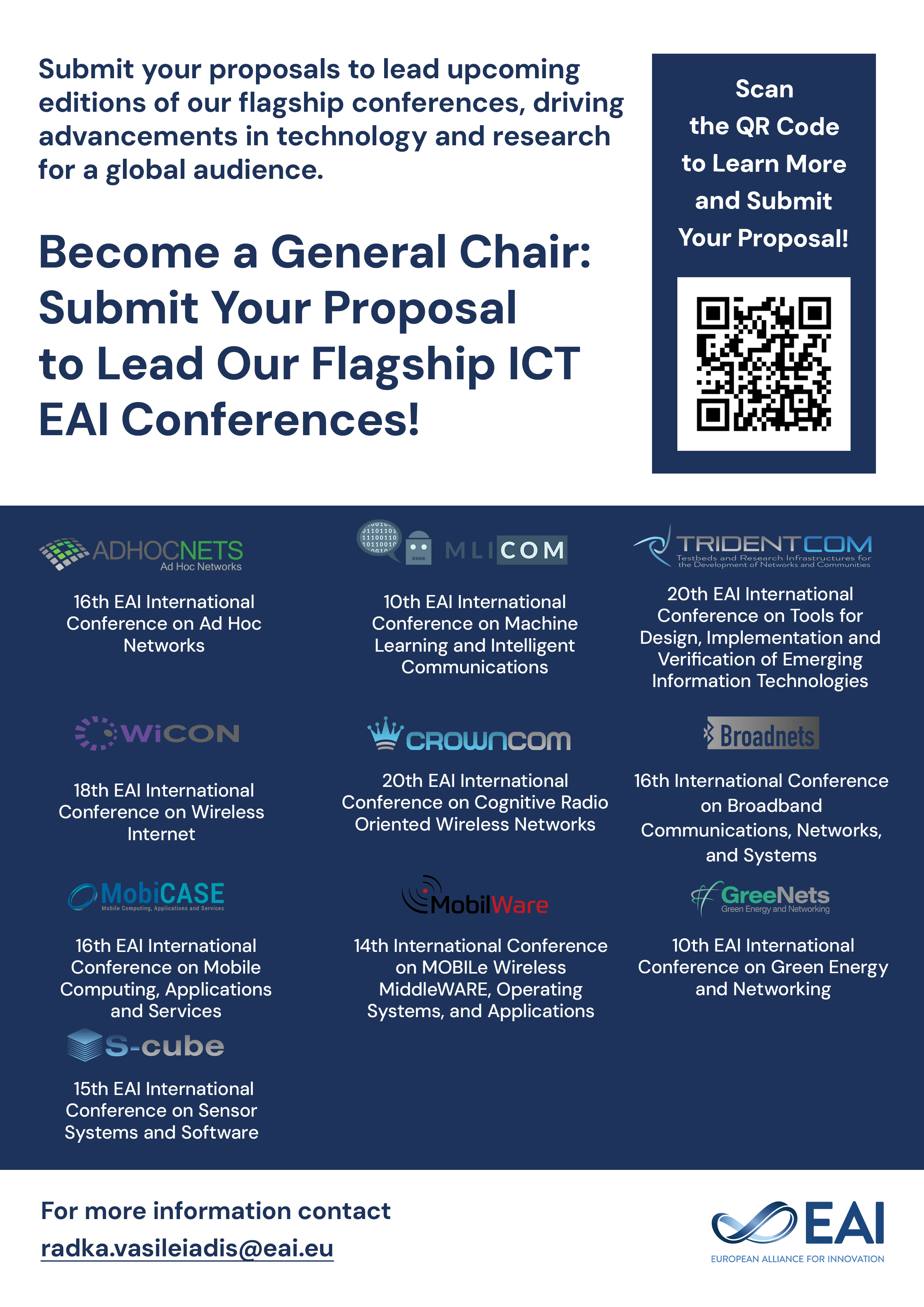
Research Article
Comparative Analysis of Machine Learning Algorithms on IVR data
@ARTICLE{10.4108/eai.12-6-2018.154814, author={Aswin. T.S and Himanshu Batra and Mathangi Ramachandran}, title={Comparative Analysis of Machine Learning Algorithms on IVR data}, journal={EAI Endorsed Transactions on Energy Web and Information Technologies}, volume={5}, number={18}, publisher={EAI}, journal_a={EW}, year={2018}, month={6}, keywords={IVR data classification, machine learning on IVR data, text classification, analysis of machine learning methods}, doi={10.4108/eai.12-6-2018.154814} }
- Aswin. T.S
Himanshu Batra
Mathangi Ramachandran
Year: 2018
Comparative Analysis of Machine Learning Algorithms on IVR data
EW
EAI
DOI: 10.4108/eai.12-6-2018.154814
Abstract
We aim to classify IVR data (Interactive Voice Response) and provide a detailed summary of the methods and techniques we employed to create a classifier model of reasonably high accuracy. This model is built to process large datasets of customer grievance lines (in IVR form converted to text), clustering and classifying these lines as accurately as possible. Here machine learning algorithms have been used to build text classifier models, in both supervised and unsupervised approaches on IVR datasets, and their labels have been checked for accuracy. Documentation of the methods applied gives insight into how best we can extract meaningful information and perform classification. By ensuring optimization in the classification of large datasets, resources like time, money and human effort are saved in the long run, and the data is more purposeful.
Copyright © 2018 Aswin.T.S et al., licensed to EAI. This is an open access article distributed under the terms of the Creative Commons Attribution licence (http://creativecommons.org/licenses/by/3.0/), which permits unlimited use, distribution and reproduction in any medium so long as the original work is properly cited.