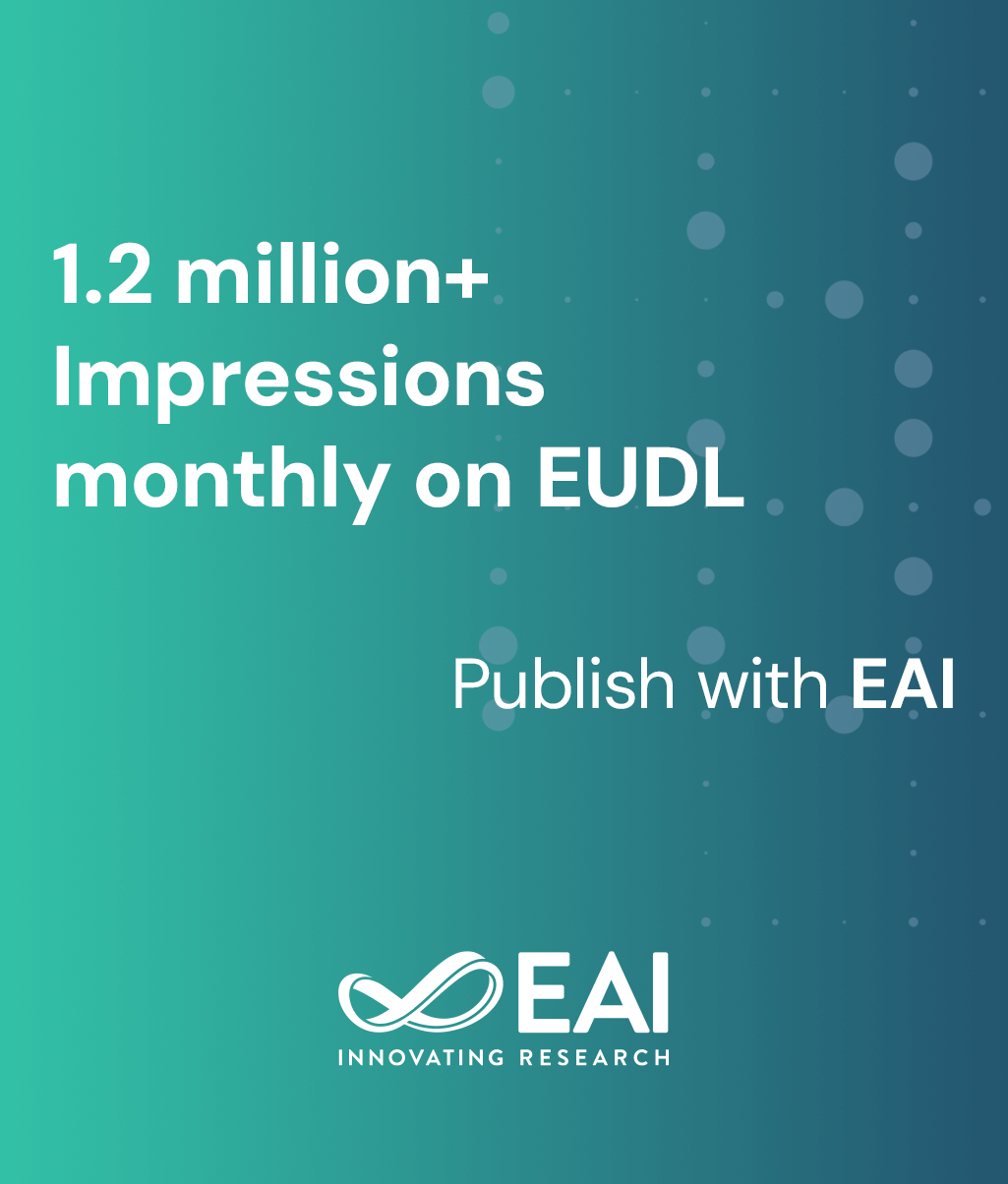
Research Article
Predicting Instructors Performance in Higher Education Systems
@ARTICLE{10.4108/eai.12-6-2018.154811, author={Dr. K. Kalaiselvi and J. Sowmiya}, title={Predicting Instructors Performance in Higher Education Systems}, journal={EAI Endorsed Transactions on Energy Web and Information Technologies}, volume={5}, number={18}, publisher={EAI}, journal_a={EW}, year={2018}, month={6}, keywords={Knowledge mining; Educational sector; knowledge discovery process; data mining algorithms, imbalance attributes and Data analysis.}, doi={10.4108/eai.12-6-2018.154811} }
- Dr. K. Kalaiselvi
J. Sowmiya
Year: 2018
Predicting Instructors Performance in Higher Education Systems
EW
EAI
DOI: 10.4108/eai.12-6-2018.154811
Abstract
In recent years, knowledge mining has become one of the effective tools for data analysis and information management systems. Educational sector is the recent research endeavors that make use of data mining algorithms. Prior works carried in data mining algorithms like J48 Decision Tree, Multilayer Perception, Naïve Bayes, and Sequential Minimal Optimization impose issues like the curse of dimensionality, cardinality and imbalance attributes. In this paper, we have proposed FA-Paired t-test method which is a novel knowledge discovery process to predict the performance of the instructors. The aim of the study is to find the factors that associated for the prediction of teaching quality. Thus, the contribution of the factor analysis method helps to find the relevant attributes from a set of attributes. Then, the selected attributes are fed as input to paired t-test model which find the associations between those linked attributes. The selected attributes are experimenting using SPSS modeler. Many attributes test for the performance evaluation. It is strongly found that content arrangement, delivery of speech, effective class hours and completion of the course helps to predict the quality of the teaching. In addition to, the proposed model is compared to prior two classifiers, named, J48DT and Naïve Bayes which shows our proposed method works better than other two classifiers in term of Attribute reduction and evaluation process.
Copyright © 2018 K.Kalaiselvi and J. Sowmiya, licensed to EAI. This is an open access article distributed under the terms of the Creative Commons Attribution licence (http://creativecommons.org/licenses/by/3.0/), which permits unlimited use, distribution and reproduction in any medium so long as the original work is properly cited.