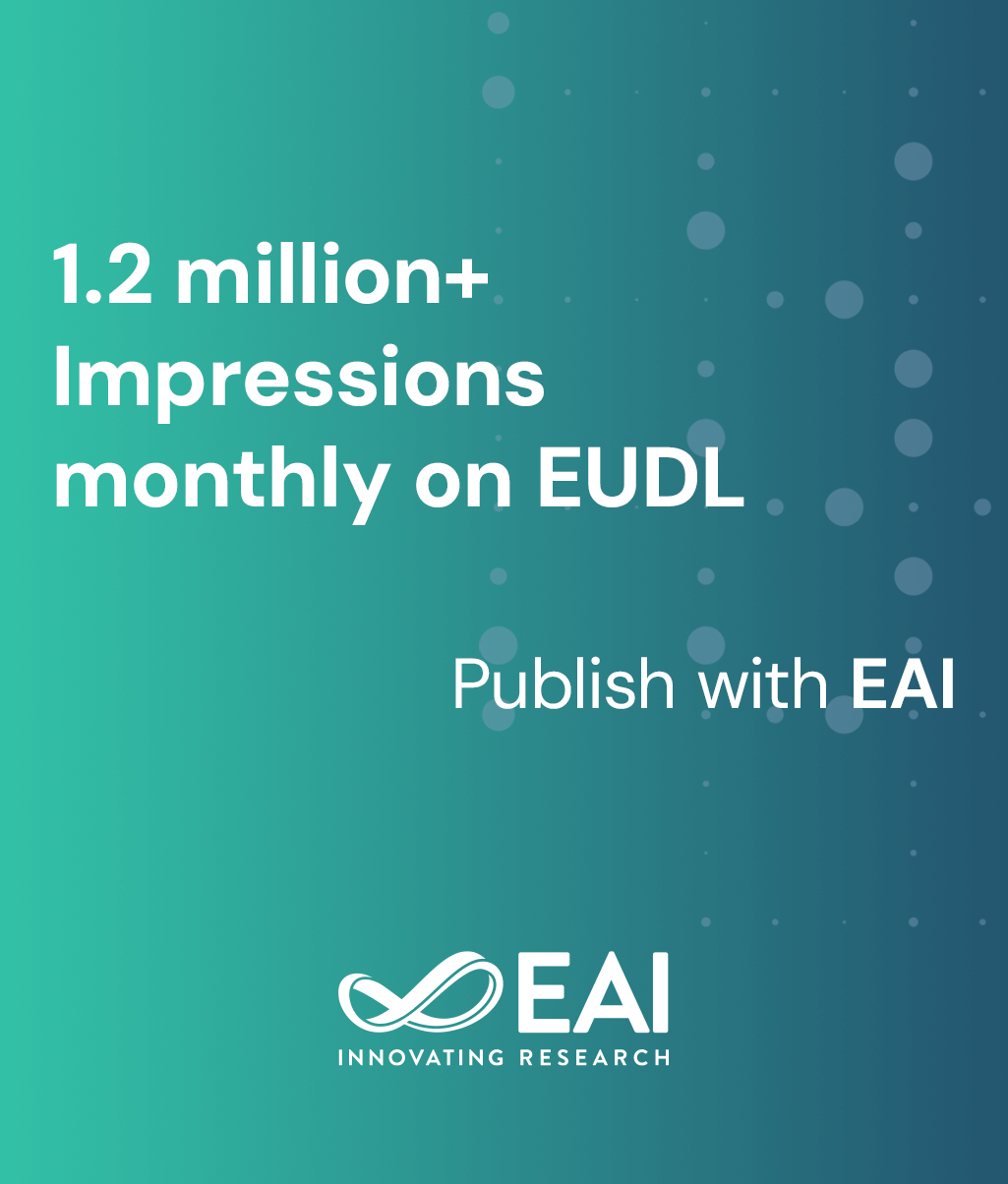
Research Article
Chinese fingerspelling sign language recognition using a nine-layer convolutional neural network
@ARTICLE{10.4108/eai.12-10-2020.166555, author={Ya Gao and Chengchong Jia and Hongli Chen and Xianwei Jiang}, title={Chinese fingerspelling sign language recognition using a nine-layer convolutional neural network}, journal={EAI Endorsed Transactions on e-Learning}, volume={7}, number={20}, publisher={EAI}, journal_a={EL}, year={2020}, month={10}, keywords={deaf-mute, sign language recognition, Chinese fingerspelling recognition, convolutional neural network, stochastic pooling, batch normalization technology, dropout method}, doi={10.4108/eai.12-10-2020.166555} }
- Ya Gao
Chengchong Jia
Hongli Chen
Xianwei Jiang
Year: 2020
Chinese fingerspelling sign language recognition using a nine-layer convolutional neural network
EL
EAI
DOI: 10.4108/eai.12-10-2020.166555
Abstract
INTRODUCTION: Sign language is a form of communication and exchange of ideas by people who are hearing-impaired or unable to speak. Chinese fingerspelling is an important component of Chinese sign language, which is suitable for denoting terminology and using as the basis of gesture sign language learning. OBJECTIVES: We propose a nine-layer convolutional neural network (CNN) for the classification of Chinese sign language. METHODS: With self-learning and self-organization abilities, CNN is committed to processing data with similar network structure. CNN has a good application prospect in the aspect of image classification andplays a very important role in the classification of Chinese sign language. RESULTS: Through experiments on 1320 data samples of 30 categories, the results show that the classification accuracy based on the nine-layer convolutional neural network can reach up to 89.69± 2.10 %, it can be seen that this method can effectively classify Chinese gestures. CONCLUSION: We proposed a nine-layer convolutional neural network (CNN) that can classify Chinese sign language.
Copyright © 2020 Ya Gao et al., licensed to EAI. This is an open access article distributed under the terms of the Creative Commons Attribution license, which permits unlimited use, distribution and reproduction in any medium so long as the original work is properly cited.