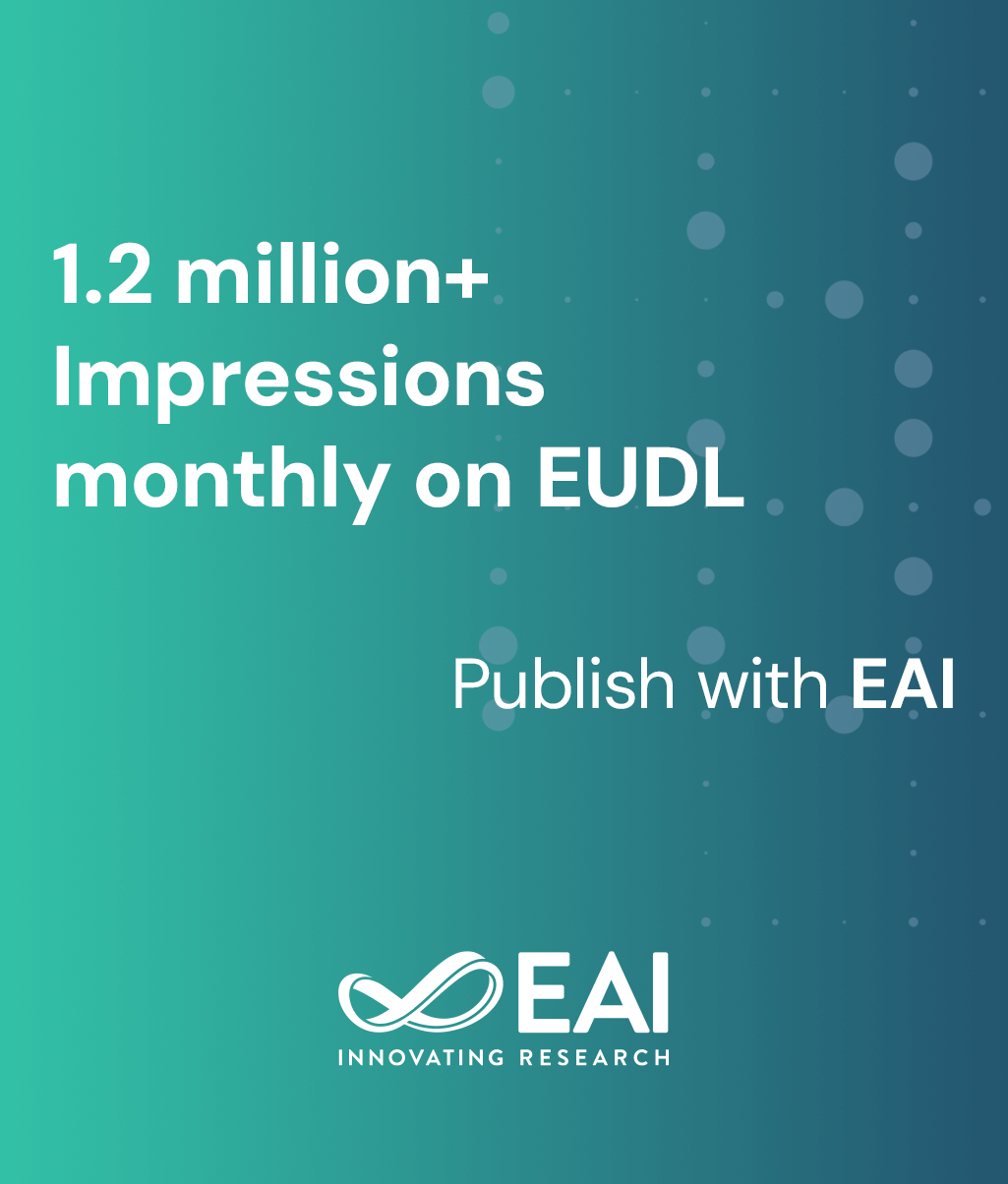
Research Article
Sentiment Analysis of the Body-Shaming Beauty Vlog Comments
@INPROCEEDINGS{10.4108/eai.12-10-2019.2296530, author={Jajam Haerul Jaman and Hannie Hannie and Martina Sari Simatupang}, title={Sentiment Analysis of the Body-Shaming Beauty Vlog Comments}, proceedings={Proceedings of the 7th Mathematics, Science, and Computer Science Education International Seminar, MSCEIS 2019, 12 October 2019, Bandung, West Java, Indonesia}, publisher={EAI}, proceedings_a={MSCEIS}, year={2020}, month={7}, keywords={body shaming na\~{n}ve bayes classifier sentiment analysis}, doi={10.4108/eai.12-10-2019.2296530} }
- Jajam Haerul Jaman
Hannie Hannie
Martina Sari Simatupang
Year: 2020
Sentiment Analysis of the Body-Shaming Beauty Vlog Comments
MSCEIS
EAI
DOI: 10.4108/eai.12-10-2019.2296530
Abstract
Sentiment analysis can be used as a solution to identify body-shaming comments with the classification method using the Naïve Bayes Classifier algorithm. Naïve Bayes Classifier uses the concept of probability of each class in its classification learning. The purpose of this study is to predict or classify comment data based on shaming and non-shaming sentiment classes. The test in this study was carried out with ten different scenarios using the R programming language with RStudio tools which were then evaluated using the confusion matrix to determine the best classifier model. The evaluation results with the confusion matrix found that the best model classifier is a scenario with a comparison of training data and testing data 90:10 and applying to stem at the preprocessing. This scenario achieves an accuracy of 98.48% with an error rate of 1.52%. Recall is 99.53%, specificity is 66.67%, precision is 98.90%, and F-measure is 99.21%.