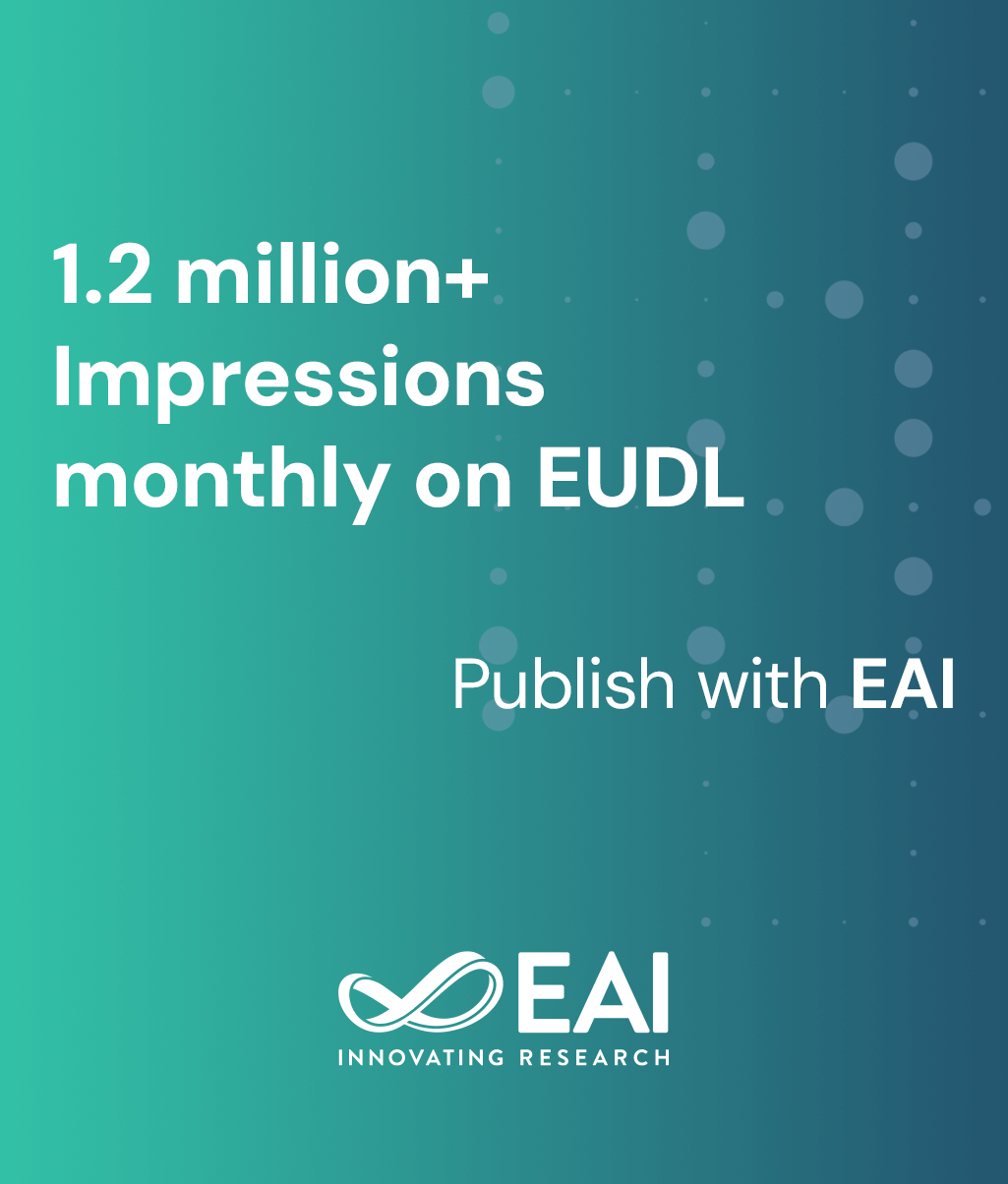
Research Article
Bayesian Inference for Statistics Recurrent Stochastic Volatility
@INPROCEEDINGS{10.4108/eai.12-1-2024.2347143, author={Weihang Qiu}, title={Bayesian Inference for Statistics Recurrent Stochastic Volatility}, proceedings={Proceedings of the 3rd International Conference on Big Data Economy and Digital Management, BDEDM 2024, January 12--14, 2024, Ningbo, China}, publisher={EAI}, proceedings_a={BDEDM}, year={2024}, month={6}, keywords={stochastic volatility cyclic neural network nonlinearity long memory}, doi={10.4108/eai.12-1-2024.2347143} }
- Weihang Qiu
Year: 2024
Bayesian Inference for Statistics Recurrent Stochastic Volatility
BDEDM
EAI
DOI: 10.4108/eai.12-1-2024.2347143
Abstract
Stochastic volatility model is an important model for studying financial time series. Due to the very complex volatility of financial markets, it is particularly important to analyze the characteristics of non-linearity and long memory. Moreover, the traditional stochastic volatility model does not explain the self-dependence in statistical sense, which will affect the model's capture of volatility effect.In this paper, a statistical recurrence volatility model is established. The cyclic neural network is used to model the time series so as to draw the long dependence at the moment, thus overcoming the limitation that the traditional random model can only explain the short dependence, and the nonlinear transformation is used to replace the logarithmic transformation of the traditional random model. We combine in-depth learning with stock volatility to simulate five international stock index data sets: Swiss stock index SWX, French market index CAC, and Singapore stock market index STI. In addition, SMC and particle MCMC are used to perform Bayesian inference on this parameter state space model.