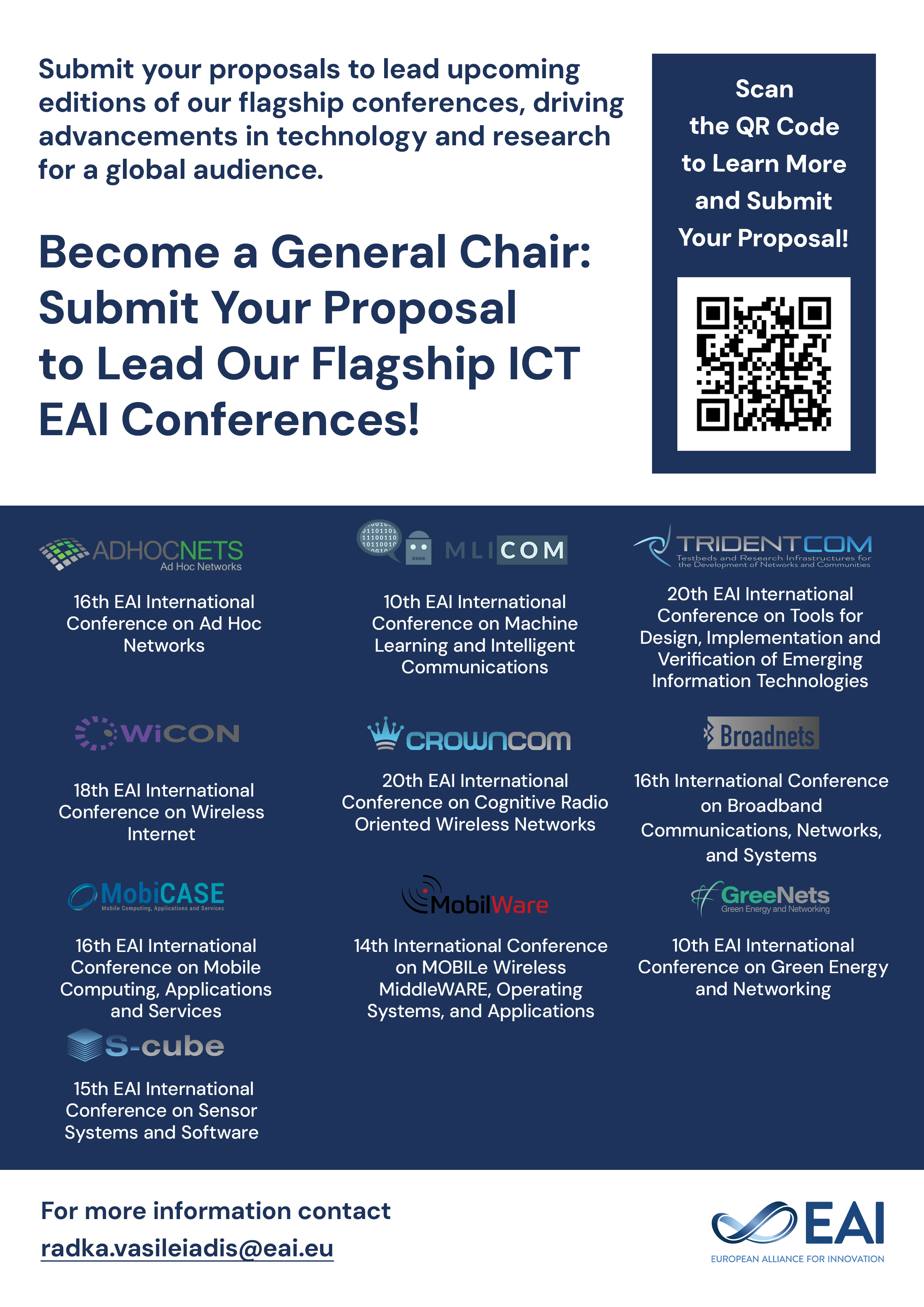
Research Article
Sentiment Analysis of Health Protocol Policy Using K-Nearest Neighbor and Cosine Similarity
@INPROCEEDINGS{10.4108/eai.11-10-2022.2326274, author={N. Ritha and N. Hayaty and T. Matulatan and A. Uperiati and M. Rathomi and M. Bettiza and F. Farasalsabila}, title={Sentiment Analysis of Health Protocol Policy Using K-Nearest Neighbor and Cosine Similarity}, proceedings={Proceedings of the 1st International Conference on Sustainable Engineering Development and Technological Innovation, ICSEDTI 2022, 11-13 October 2022, Tanjungpinang, Indonesia}, publisher={EAI}, proceedings_a={ICSEDTI}, year={2023}, month={1}, keywords={sentiment analysis health protocol k-nn cosine similarity}, doi={10.4108/eai.11-10-2022.2326274} }
- N. Ritha
N. Hayaty
T. Matulatan
A. Uperiati
M. Rathomi
M. Bettiza
F. Farasalsabila
Year: 2023
Sentiment Analysis of Health Protocol Policy Using K-Nearest Neighbor and Cosine Similarity
ICSEDTI
EAI
DOI: 10.4108/eai.11-10-2022.2326274
Abstract
As an effort to handle COVID-19 transmission, the Indonesian government introduced various regulations such as Health Protocol Policy. Meanwhile, the policy has become a public discussion on social media, such as Twitter. To classify people's opinions on the social media, sentiment analysis technique is used. This method is widely utilized in natural language processing (NLP), data mining, and information retrieval. Therefore, this study aimed to analyze Twitter users’ opinions toward health protocol policy. There were several approaches to conducting sentiment analysis, including the TF-IDF method for word weighting and the K-Nearest Neighbor algorithm for data classification. Also, the similarity between two documents or texts was measured using cosine similarity. The processed data were categorized into three, namely positive, negative, and neutral. The system classification results showed 43.94% positive sentiment, 9.06% negative, and 46.99% neutral assuming the value of K = 4.