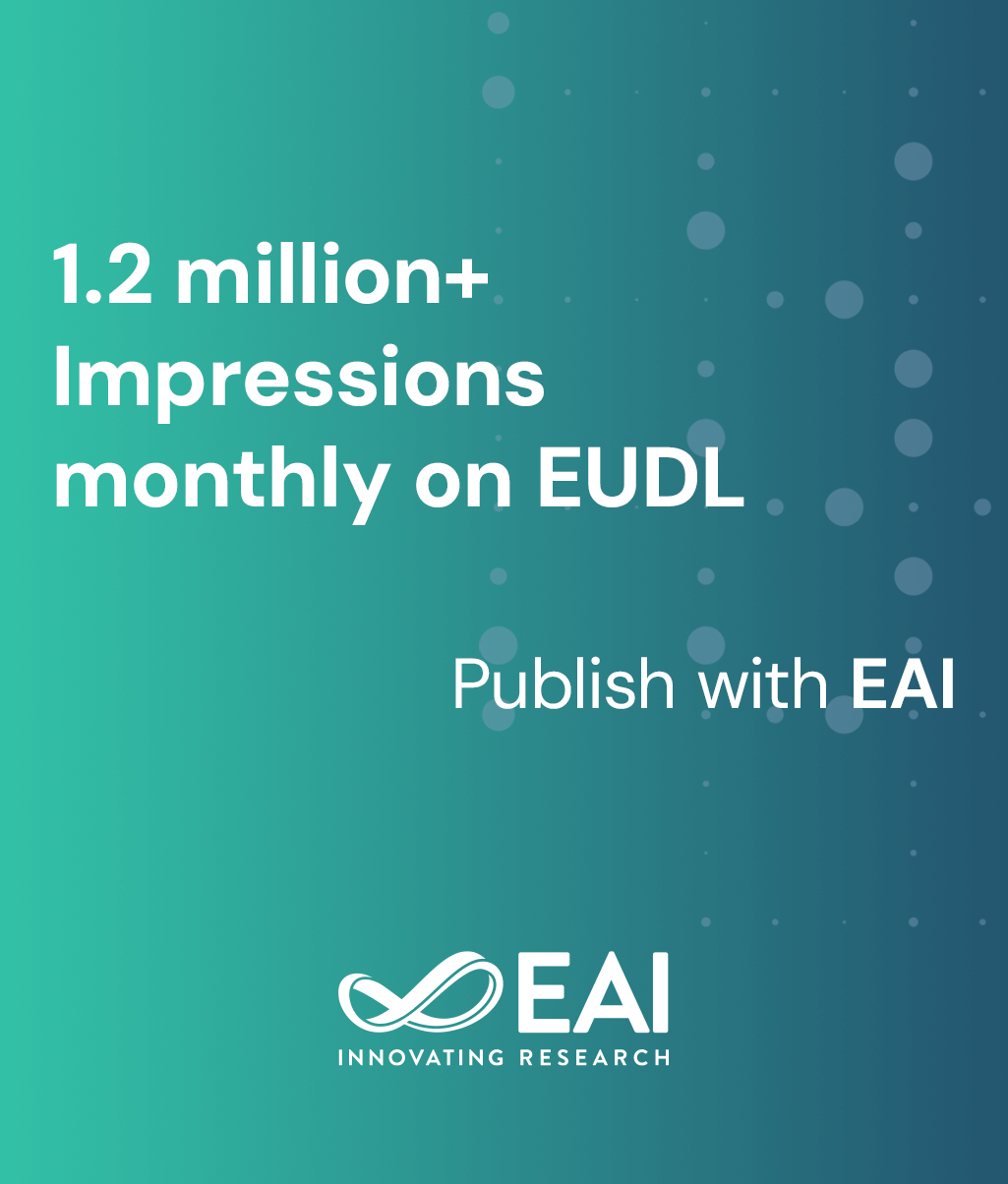
Research Article
Stock Price Prediction Based on Shareholding Network Topology and LSTM Model
@INPROCEEDINGS{10.4108/eai.1-9-2023.2338695, author={Yu Xu and Dan Wang and Jianshu Hao}, title={Stock Price Prediction Based on Shareholding Network Topology and LSTM Model}, proceedings={Proceedings of the 2nd International Conference on Public Management, Digital Economy and Internet Technology, ICPDI 2023, September 1--3, 2023, Chongqing, China}, publisher={EAI}, proceedings_a={ICPDI}, year={2023}, month={11}, keywords={network topology line long short-term memory (lstm) model stock price pre-diction risk transfer}, doi={10.4108/eai.1-9-2023.2338695} }
- Yu Xu
Dan Wang
Jianshu Hao
Year: 2023
Stock Price Prediction Based on Shareholding Network Topology and LSTM Model
ICPDI
EAI
DOI: 10.4108/eai.1-9-2023.2338695
Abstract
In the stock market, stock price forecasting is important for investors' decisions. Due to the nonlinearity, high noise, and strong temporal variability of stock price data, tradi-tional methods have shortcomings in forecasting tasks. Since based on the structure and function of recurrent neural networks, the Long Short Term Memory (LSTM) model has stronger analytical capabilities for this type of time series data. In stock prices predic-tion, it is important to consider external factors as well as network structures within shareholdings. In this paper,the directed holding network will be vectorized using the LINE algorithm, and four A-share listed companies will be analyzed and compared using three distinct stock price datasets. The study indicates that companies' stock prices are significantly impacted by the relationships between shareholdings and the fluctua-tions in related company stock prices from the perspective of network topology, leading to risk spreads within the shareholding network. Additionally, category characteristics can serve as effective assessment features.