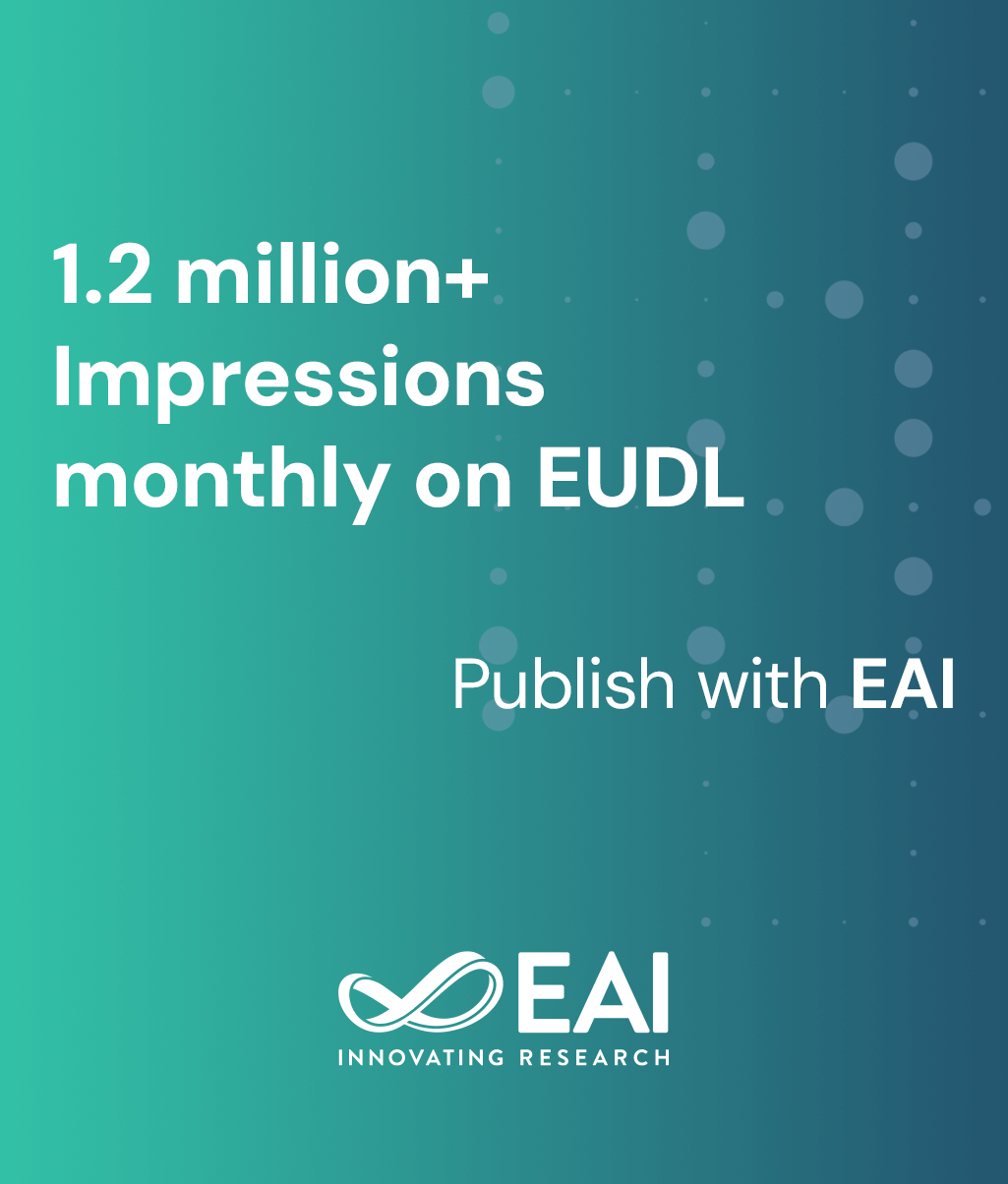
Research Article
Throughput Loss in Task Scheduling due to Server State Uncertainty
@INPROCEEDINGS{10.4108/ICST.VALUETOOLS2009.7547, author={Carri W. Chan and Nick Bambos}, title={Throughput Loss in Task Scheduling due to Server State Uncertainty}, proceedings={4th International ICST Conference on Performance Evaluation Methodologies and Tools}, publisher={ICST}, proceedings_a={VALUETOOLS}, year={2010}, month={5}, keywords={}, doi={10.4108/ICST.VALUETOOLS2009.7547} }
- Carri W. Chan
Nick Bambos
Year: 2010
Throughput Loss in Task Scheduling due to Server State Uncertainty
VALUETOOLS
ICST
DOI: 10.4108/ICST.VALUETOOLS2009.7547
Abstract
We consider a dynamic scheduling system where a single controller selects ‘tasks’ to service over U ‘servers’ of fluctuating quality/ speed. The quality/speed of each server determines the likelihood of successful service should a task be assigned to that server. The goal is to maximize the total expected number of tasks successfully served over a fixed time horizon (aggregate throughput) given only one server can be used in each time slot. However, the state of the servers are not known to the scheduler with certainty; at best, only statistical distributions (estimates) of the realized server states are available. We consider how the uncertainty of server state information compromises the expected aggregate throughput compared to a ‘clairvoyant’ scheduler which has instantaneous, perfect information about the realized server states. The issue of operating in uncertain environments arises in a number of scheduling applications of interest from wireless applications to computing networks to revenue management systems. The results presented in this paper provide a framework for gauging the loss due to uncertainty in such scheduling systems. First, it is shown that opportunistic scheduling (on the server of current expected best state) is throughput optimal, under uncertain (unknown) server states. Then, the throughput of the ‘clairvoyant’ scheduler is found to be upper-bounded (in general) by U times the throughput under uncertain server states; this bound is tight. Third, for bimodal and uniform server qualities/speeds better bounds are obtained–down to a factor of 2. Of course, actual throughput loss due to server state uncertainty depends on the server state distributions which are available as partial information to the scheduler. Finally, via numerical experiments we evaluate the throughput loss in various operational scenarios for wireless packet scheduling applications.