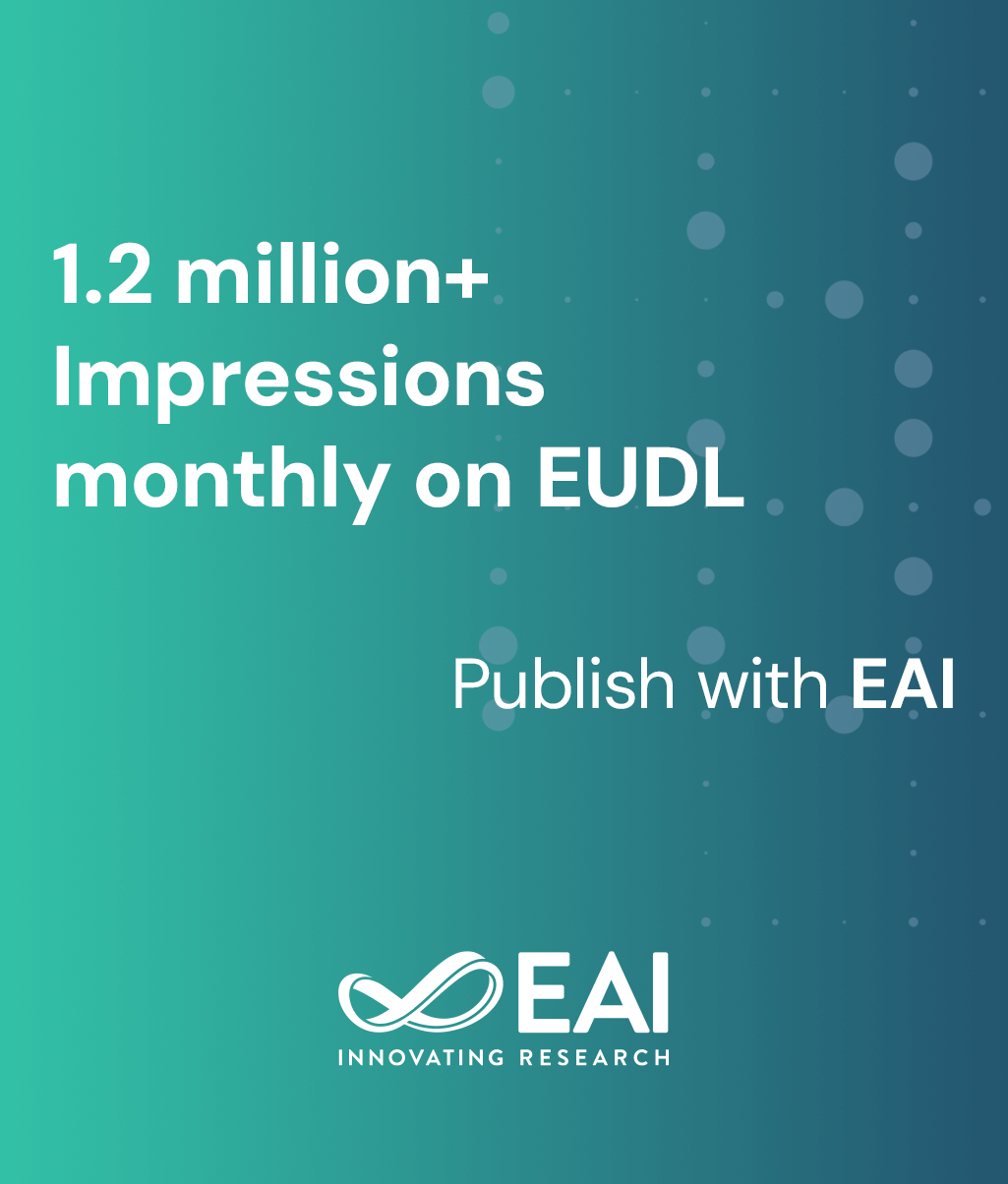
Research Article
Automated administration of the Wolf Motor Function Test for post-stroke assessment
@INPROCEEDINGS{10.4108/ICST.PERVASIVEHEALTH2010.8903, author={Eric Wade and Avinash Rao Parnandi and Maja J. Mataric}, title={Automated administration of the Wolf Motor Function Test for post-stroke assessment}, proceedings={4th International ICST Conference on Pervasive Computing Technologies for Healthcare}, proceedings_a={PERVASIVEHEALTH}, year={2010}, month={6}, keywords={Application software Automatic testing Cameras Computer science Instruments Medical treatment Neuroscience Standardization Timing Wearable sensors}, doi={10.4108/ICST.PERVASIVEHEALTH2010.8903} }
- Eric Wade
Avinash Rao Parnandi
Maja J. Mataric
Year: 2010
Automated administration of the Wolf Motor Function Test for post-stroke assessment
PERVASIVEHEALTH
ICST
DOI: 10.4108/ICST.PERVASIVEHEALTH2010.8903
Abstract
The advent of new health sensing technologies has presented us with the opportunity to gain richer data from patients undergoing clinical interventions. Such technologies are particularly suited for applications requiring temporal accuracy. The Wolf Motor Function Test (WMFT) is one such application. This assessment is an instrument used to determine functional ability of the paretic and non-paretic limbs in individuals post-stroke. It consists of 17 tasks, 15 of which are scored according to both time and a functional ability scale. We propose a technique that uses wearable sensors and performance sensors to estimate the timing of seven of these tasks. We have developed a sensing framework and an algorithm to automatically detect total movement time. We have validated the system's accuracy on the seven selected WMFT tasks. We also suggest how this framework can be adapted to the remaining tasks.