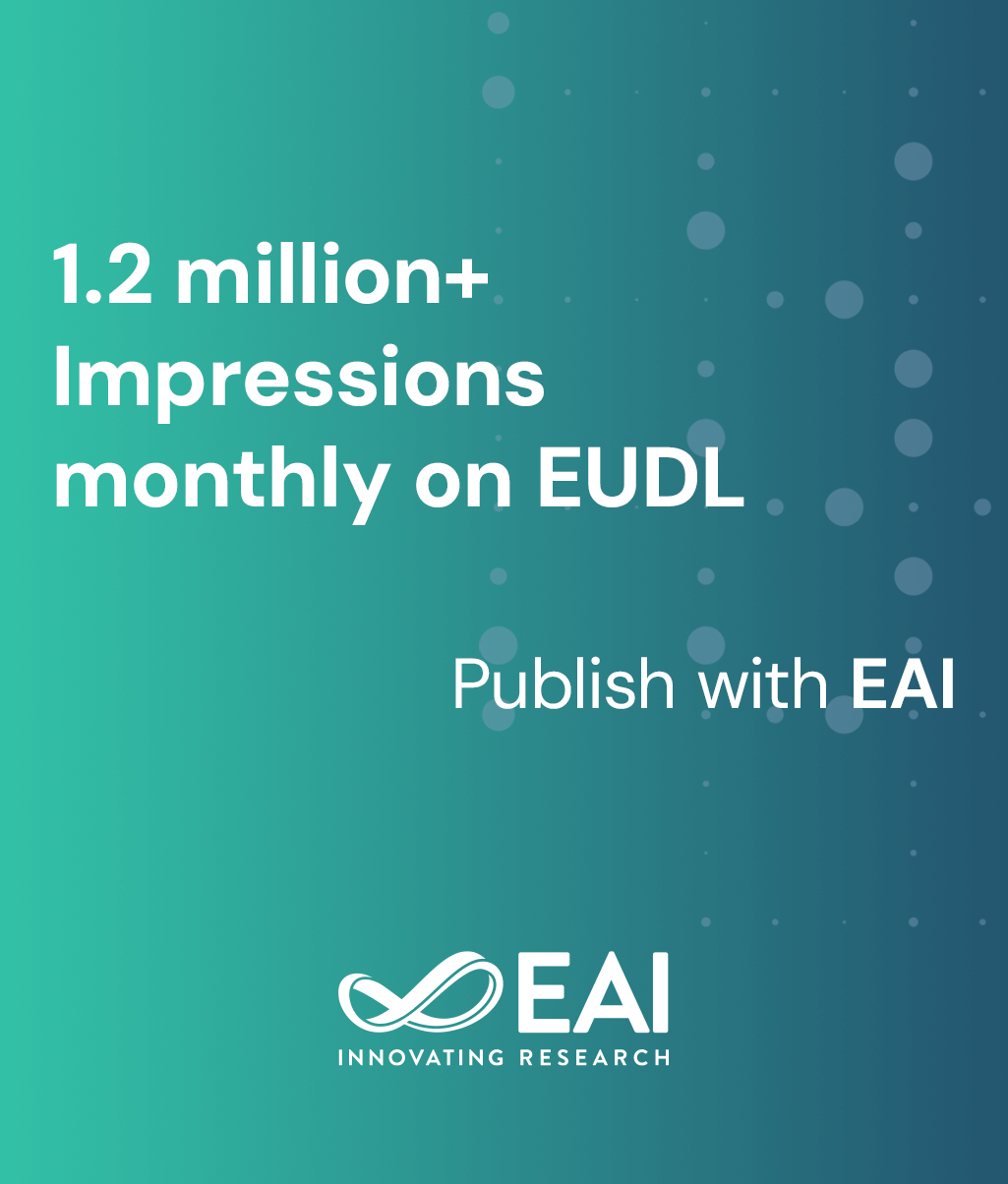
Research Article
Design and testing of lightweight inexpensive motion-capture devices with application to clinical gait analysis
@INPROCEEDINGS{10.4108/ICST.PERVASIVEHEALTH2009.6026, author={Eric Wade and Maja J Mataric}, title={Design and testing of lightweight inexpensive motion-capture devices with application to clinical gait analysis}, proceedings={3d International ICST Conference on Pervasive Computing Technologies for Healthcare}, proceedings_a={PERVASIVEHEALTH}, year={2009}, month={8}, keywords={Application software Biomedical monitoring Computer science Hardware Human robot interaction Motion analysis ehabilitation robotics Robot sensing systems Testing Wearable sensors}, doi={10.4108/ICST.PERVASIVEHEALTH2009.6026} }
- Eric Wade
Maja J Mataric
Year: 2009
Design and testing of lightweight inexpensive motion-capture devices with application to clinical gait analysis
PERVASIVEHEALTH
ICST
DOI: 10.4108/ICST.PERVASIVEHEALTH2009.6026
Abstract
The advent of more portable and affordable sensing devices has facilitated the study of rehabilitation robotics. Critical to the further development of therapies and interventions are low-cost, easy-to-use devices that can be applied in clinical and home care settings. In this paper, we present a low-cost motion capture system that relies on the opensource Player/Stage software development environment, and can be used in conjunction with a socially assistive robotic agent (or a computer interface) for various types of motor task rehabilitation training. We describe the hardware and software development for the device, and the activity recognition algorithm we developed to capture the relevant motion data. We present the overall framework in which this system can be adapted to other motor task-based rehabilitation regimens. Finally, we present initial experimental data in the domain of gait rehabilitation, in which we use the system to estimate cadence, walking speed, and stride length.