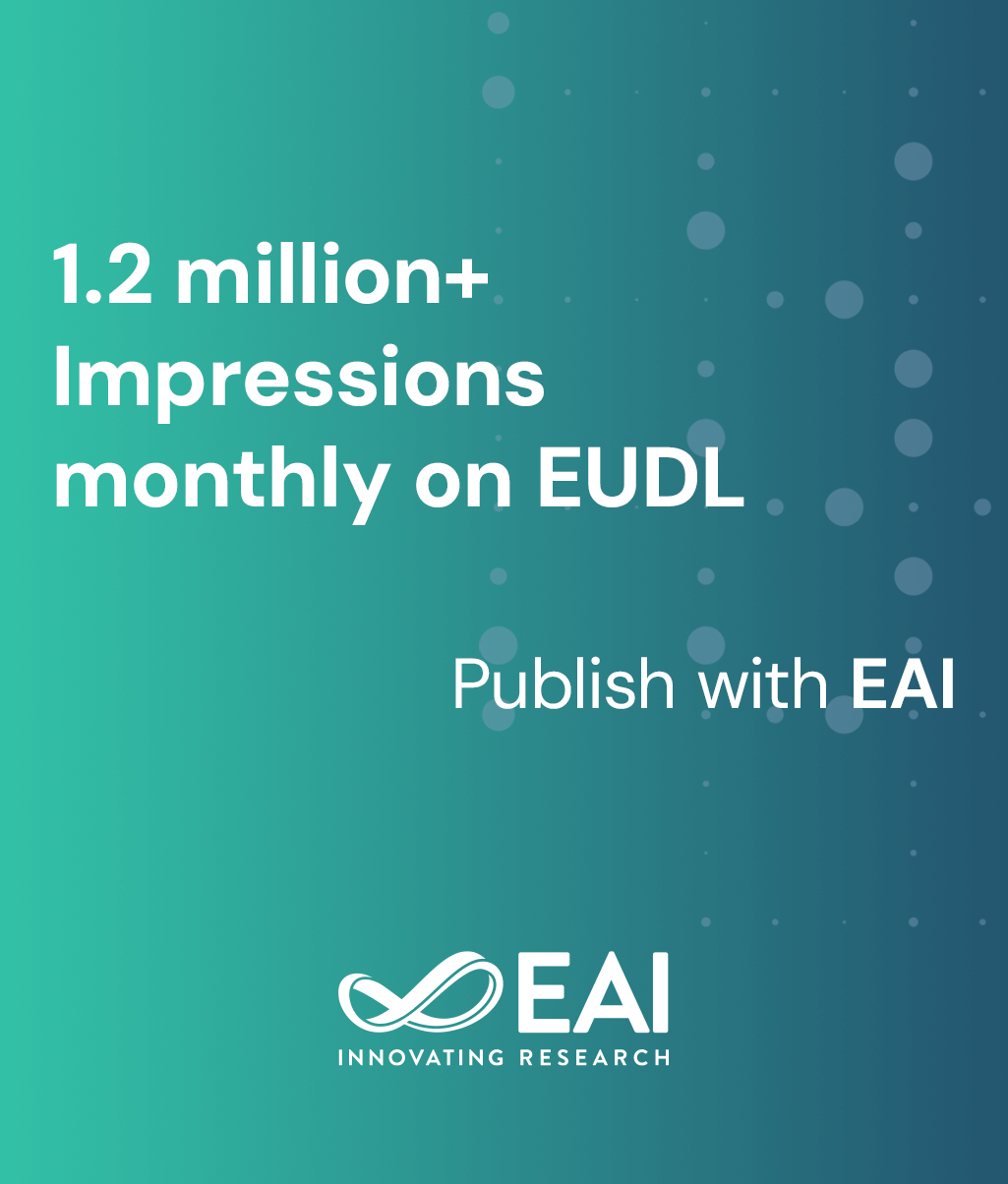
Research Article
A Content-Addressable Network for Similarity Join in Metric Spaces
@INPROCEEDINGS{10.4108/ICST.INFOSCALE2008.3526, author={Claudio Gennaro}, title={A Content-Addressable Network for Similarity Join in Metric Spaces}, proceedings={3rd International ICST Conference on Scalable Information Systems}, publisher={ICST}, proceedings_a={INFOSCALE}, year={2010}, month={5}, keywords={Similarity Join Content-Addressable Network Metric Space}, doi={10.4108/ICST.INFOSCALE2008.3526} }
- Claudio Gennaro
Year: 2010
A Content-Addressable Network for Similarity Join in Metric Spaces
INFOSCALE
ICST
DOI: 10.4108/ICST.INFOSCALE2008.3526
Abstract
Similarity join is an interesting complement of the well-established similarity range and nearest neighbors search primitives in metric spaces.
However, the quadratic computational complexity of similarity join prevents from applications on large data collections. We present MCAN+, an extension of MCAN (a Content-Addressable Network for metric objects) to support similarity self join queries. The challenge of the proposed approach is to address the problem of the intrinsic quadratic complexity of similarity joins, with the aim of limiting the elaboration time, by involving an increasing number of computational nodes as the dataset size grows. To test the scalability of MCAN+, we used a real-life dataset of color features extracted from one million images of the Flickr photo sharing website.