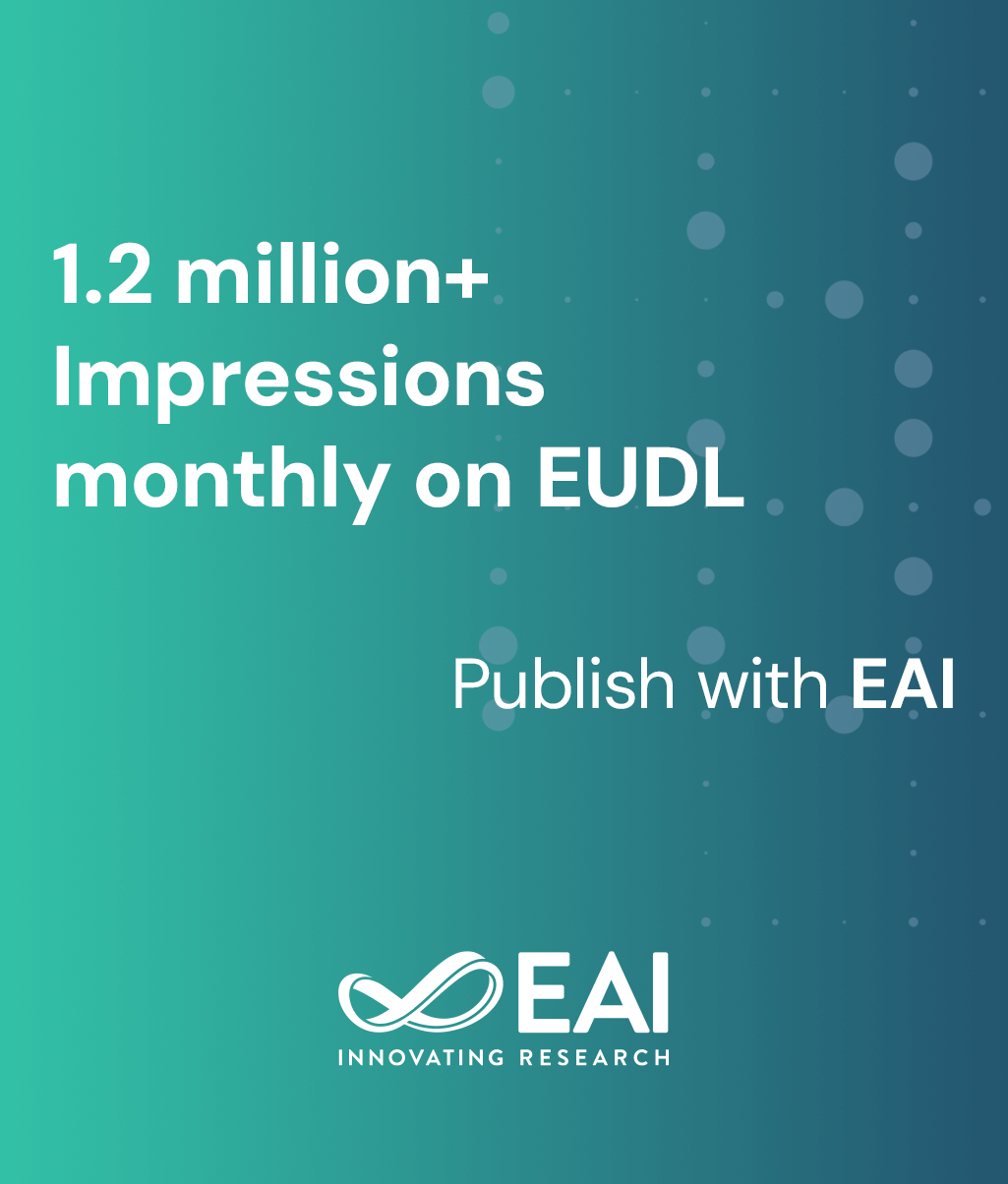
Research Article
GLAID: Designing a Game Learning Analytics Model to Analyze the Learning Process in Users with Intellectual Disabilities
@INPROCEEDINGS{10.1007/978-3-319-51055-2_7, author={Ana Cano and Baltasar Fern\^{a}ndez-Manj\^{o}n and \^{A}lvaro Garc\^{\i}a-Tejedor}, title={GLAID: Designing a Game Learning Analytics Model to Analyze the Learning Process in Users with Intellectual Disabilities}, proceedings={Serious Games, Interaction and Simulation. 6th International Conference, SGAMES 2016, Porto, Portugal, June 16-17, 2016, Revised Selected Papers}, proceedings_a={SGAMES}, year={2017}, month={1}, keywords={Serious games Intellectual Disabilities Game Learning Analytics Analytics maturity framework Educational games Down syndrome Autism spectrum disorders}, doi={10.1007/978-3-319-51055-2_7} }
- Ana Cano
Baltasar Fernández-Manjón
Álvaro García-Tejedor
Year: 2017
GLAID: Designing a Game Learning Analytics Model to Analyze the Learning Process in Users with Intellectual Disabilities
SGAMES
Springer
DOI: 10.1007/978-3-319-51055-2_7
Abstract
Educational Games are increasingly popular in teaching as they have proven to be effective learning tools. Educational videogames are beneficial for all kind of students but we think they are especially suited for users with intellectual disabilities due to the opportunity of tailoring the content to their in-game performance. Adapting the game experience to the cognitive and learning abilities to this type of students also make videogames a powerful source of learning data. In this paper we introduce the GLAID (Game Learning Analytics for Intellectual Disabilities) Model, a theoretical adaptation of a more general analytics framework. It describes how to collect, process and analyze videogame interaction data in order to provide an overview of the user learning experience, from an individualized assessment to a collective perspective. But to obtain these goals it is necessary to take into account the restrictions and special needs of users with intellectual disabilities both in the learning design and in translating them into game mechanics and the corresponding observables that will be collected for the subsequent data analysis. We conclude with a discussion and considerations about the model and future steps to follow in our investigation.