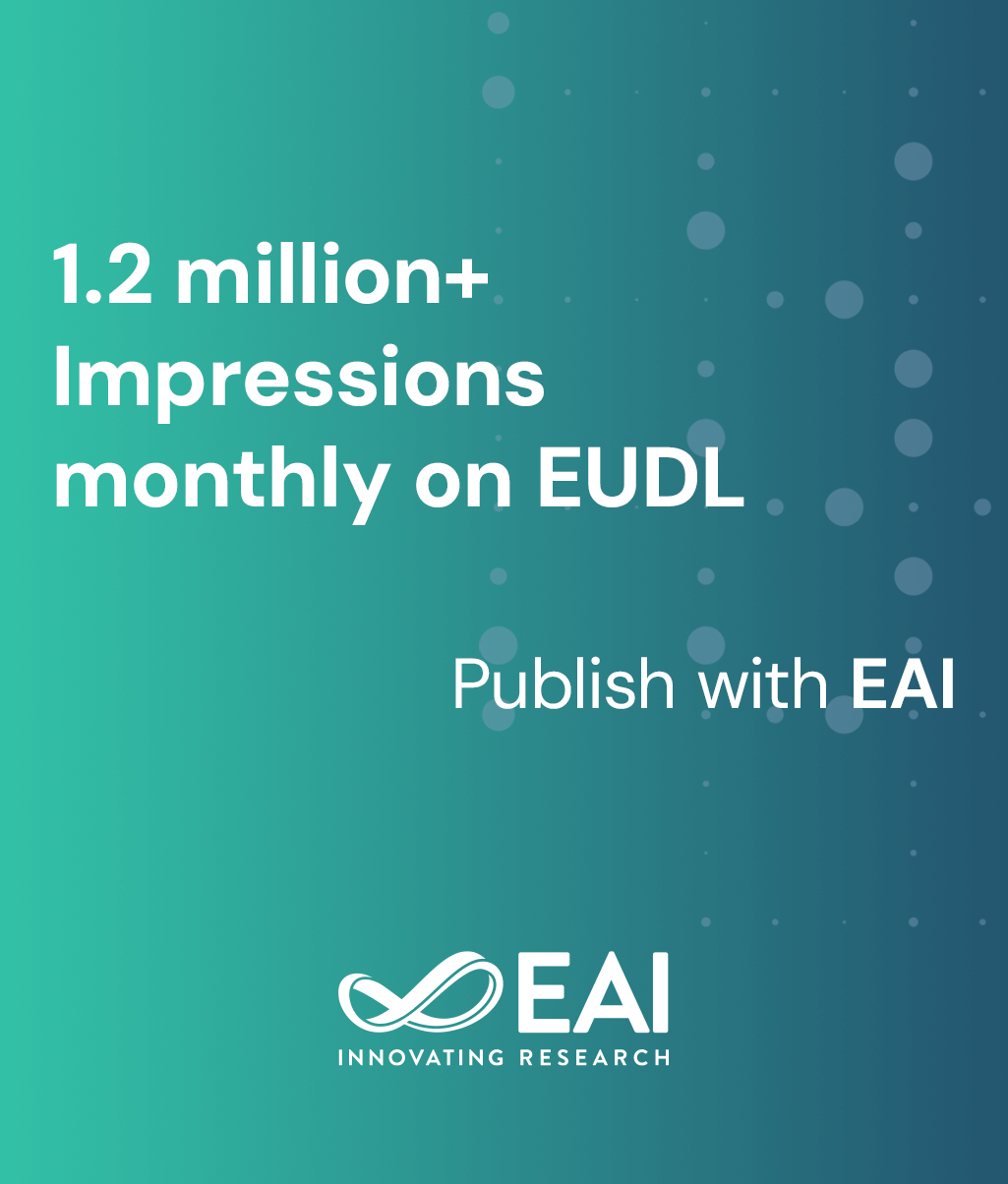
Research Article
Comparison of Thermal Load Models for MILP-Based Demand Response Planning
@INPROCEEDINGS{10.1007/978-3-030-45694-8_9, author={Pedro Magalh\"{a}es and Carlos Antunes}, title={Comparison of Thermal Load Models for MILP-Based Demand Response Planning}, proceedings={Sustainable Energy for Smart Cities. First EAI International Conference, SESC 2019, Braga, Portugal, December 4--6, 2019, Proceedings}, proceedings_a={SESC}, year={2020}, month={6}, keywords={Demand response Thermal load model Mixed-integer linear programming}, doi={10.1007/978-3-030-45694-8_9} }
- Pedro Magalhães
Carlos Antunes
Year: 2020
Comparison of Thermal Load Models for MILP-Based Demand Response Planning
SESC
Springer
DOI: 10.1007/978-3-030-45694-8_9
Abstract
Demand response has the potential to reduce end-users electricity costs by promoting judicious use of existing power system infrastructure. This is most often assumed to require the adoption of time-varying electricity prices which can make load scheduling and energy resource management difficult to carry out in a time-effective and comfortable way without computational assistance and automated control. Automated home energy management systems can facilitate this process including by providing users with optimised plans. Creating these plans requires optimisation tools operating on mathematical models of the underlying problem. Mixed-integer linear programming (MILP) has been used extensively for this purpose though increasing complexity and time resolution can render this approach impractical. In this paper, we describe and compare MILP formulations of the same demand response problems using alternative thermal load models. The results, obtained using a state-of-the-art solver, can be summarised as follows: (1) the elimination of continuous temperature variables in one thermal load submodel increased the computation time in 99% of cases and by 981% on average; (2) two new discrete control formulations leading to a 40% reduction in the number of binary variables relative to the standard formulation were found to decrease the computation time in approximately 63% of cases and by 38–40% on average. Efforts are ongoing to evaluate these techniques under more diverse scenarios.