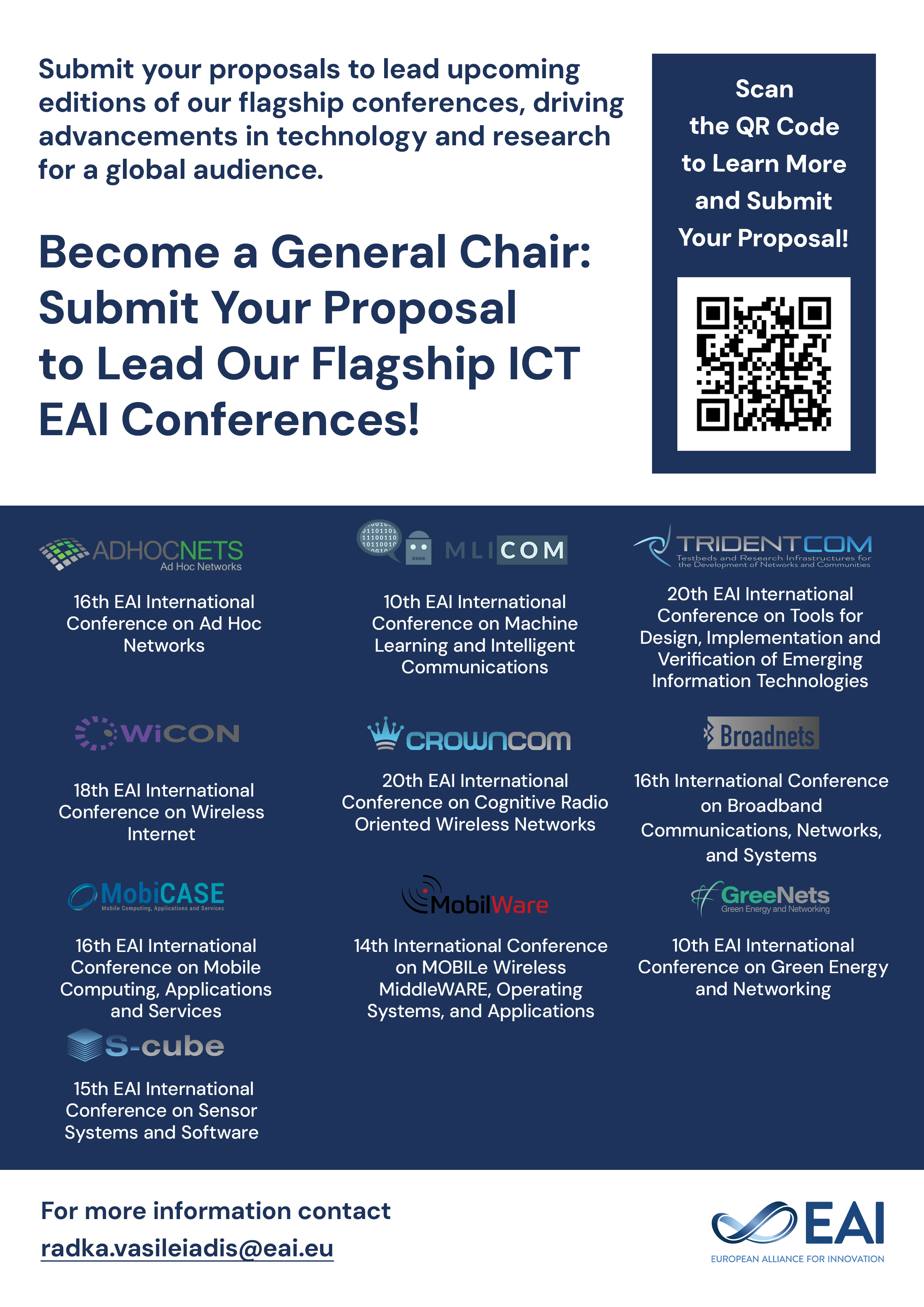
Research Article
Reverse Power Flow Detection Using Optimally Placed μPMUs in a Distribution System
@INPROCEEDINGS{10.1007/978-3-030-45694-8_8, author={Philip Eloja and Niko Jorda and Michael Pedrasa}, title={Reverse Power Flow Detection Using Optimally Placed μPMUs in a Distribution System}, proceedings={Sustainable Energy for Smart Cities. First EAI International Conference, SESC 2019, Braga, Portugal, December 4--6, 2019, Proceedings}, proceedings_a={SESC}, year={2020}, month={6}, keywords={Reverse power flow (RPF) µPMU Machine learning}, doi={10.1007/978-3-030-45694-8_8} }
- Philip Eloja
Niko Jorda
Michael Pedrasa
Year: 2020
Reverse Power Flow Detection Using Optimally Placed μPMUs in a Distribution System
SESC
Springer
DOI: 10.1007/978-3-030-45694-8_8
Abstract
The rise in the accessibility of photovoltaic (PV) generators to consumers increases the possibility of reverse power flow (RPF) in the electric distribution system. RPF occurs when power flows to the design of the system. Overvoltage, power losses and protection system coordination are among the problems that could occur due to the presence of RPF. This paper describes an algorithm to detect the presence of RPF using optimally-placed micro-phasor measurement units (µPMUs) in the IEEE 34-Bus System with 5 PV generators. A machine learning algorithm based on a feedforward artificial neural network (ANN) was developed. The algorithm was able to detect the presence of RPF using (1) voltage and current and (2) polar- and (3) rectangular-impedance methods for training. The algorithm was also able to detect RPF under scenarios that were not used during the training process. Sensitivity analyses were performed for cases such as PV outage, PV relocation, PV addition, PV expansion and load increase. The susceptibility of the algorithm to true value errors (TVEs) was tested by adding error vectors on the µPMU measurements for both the training and testing populations.