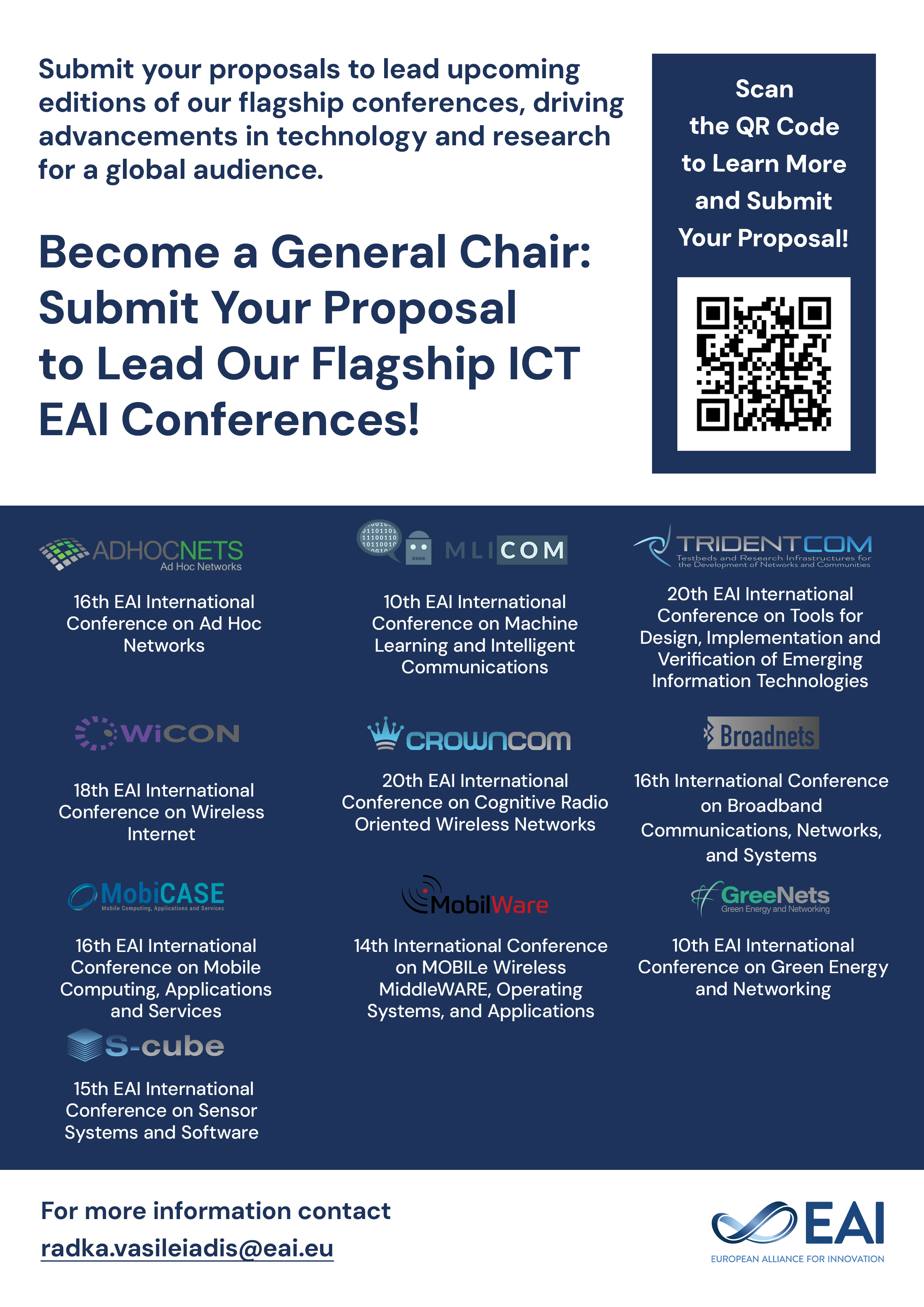
Research Article
Assisting Radiologists in X-Ray Diagnostics
@INPROCEEDINGS{10.1007/978-3-030-42029-1_8, author={Cristian Avramescu and Bercean Bogdan and Stefan Iarca and Andrei Tenescu and Sebastian Fuicu}, title={Assisting Radiologists in X-Ray Diagnostics}, proceedings={IoT Technologies for HealthCare. 6th EAI International Conference, HealthyIoT 2019, Braga, Portugal, December 4--6, 2019, Proceedings}, proceedings_a={HEALTHYIOT}, year={2020}, month={6}, keywords={Radiology Deep Learning X-ray Segmentation Bone GAN}, doi={10.1007/978-3-030-42029-1_8} }
- Cristian Avramescu
Bercean Bogdan
Stefan Iarca
Andrei Tenescu
Sebastian Fuicu
Year: 2020
Assisting Radiologists in X-Ray Diagnostics
HEALTHYIOT
Springer
DOI: 10.1007/978-3-030-42029-1_8
Abstract
Studies have shown that radiologists working together with Computer Aided Diagnostic software have increased accuracy. Automated screening software can be used to prioritize X-Rays coming in for diagnosis. We developed a suite of machine learning algorithms that aim to improve radiologist performance. It provides suggested diagnostics, a heatmap showing pathological areas and a bone subtracted version of the image which helps radiologists to identify fractures. We test different configurations for our diagnosis model, training it on both normal and enhanced images, using one or two branches. Our experiments show that adding enhanced inputs (lung segmented and bone subtracted versions of the input) increases the performance of our algorithm, which in turn increases the performance of the radiologist user. This shows that preprocessing the images before input increases model performance. More research is needed to find other preprocessing techniques, to refine existing ones, and to determine the optimal number and type of input X-Rays.