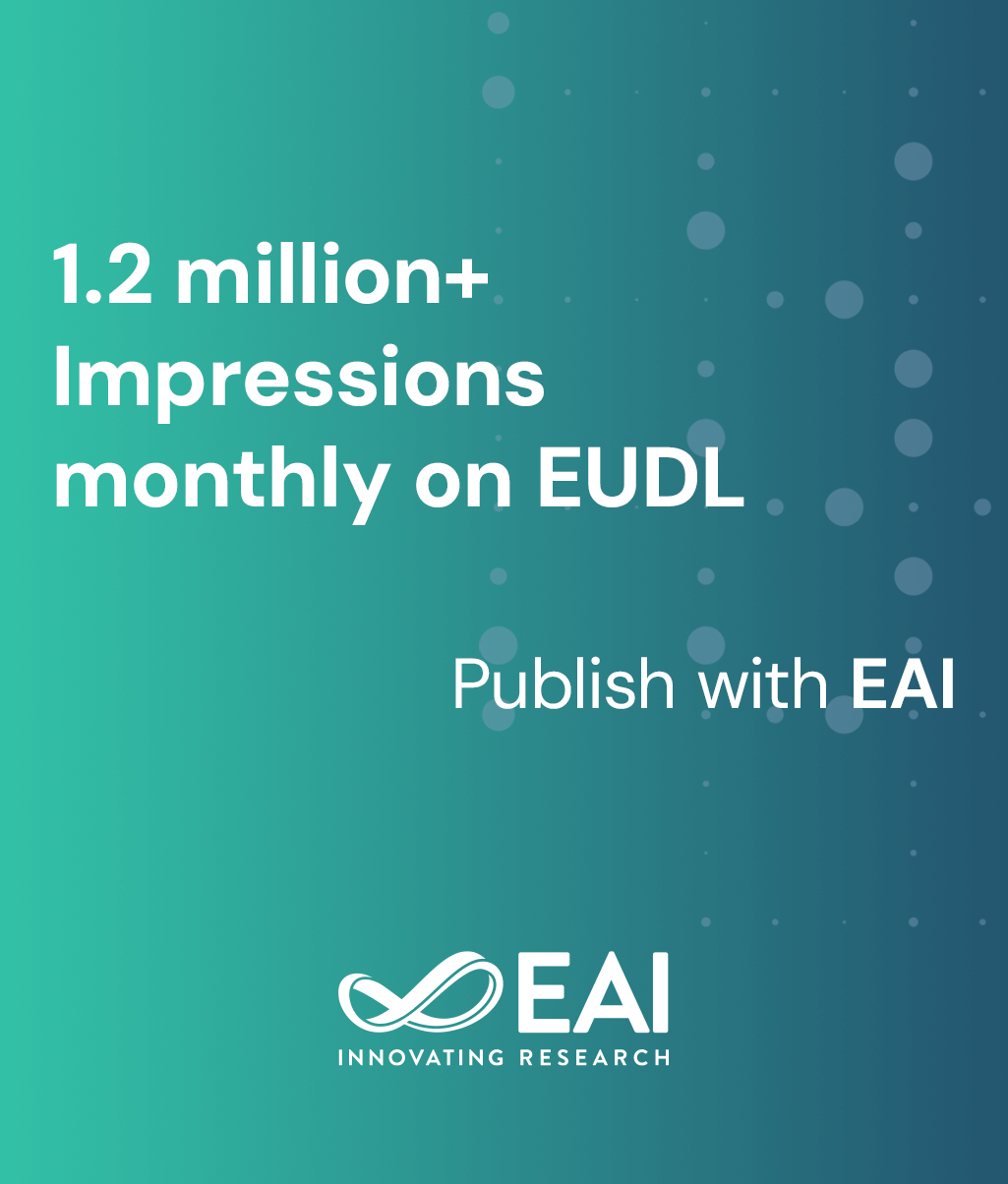
Research Article
Sensor Data Synchronization in a IoT Environment for Infants Motricity Measurement
@INPROCEEDINGS{10.1007/978-3-030-42029-1_1, author={Simone Sguazza and Alessandro Puiatti and Sandra Bernaschina and Francesca Faraci and Gianpaolo Ramelli and Vincenzo D’Apuzzo and Emmanuelle Rossini and Michela Papandrea}, title={Sensor Data Synchronization in a IoT Environment for Infants Motricity Measurement}, proceedings={IoT Technologies for HealthCare. 6th EAI International Conference, HealthyIoT 2019, Braga, Portugal, December 4--6, 2019, Proceedings}, proceedings_a={HEALTHYIOT}, year={2020}, month={6}, keywords={IoT system Sensor data synchronization Activity inference NDD early detection Healthy youth Infants Play}, doi={10.1007/978-3-030-42029-1_1} }
- Simone Sguazza
Alessandro Puiatti
Sandra Bernaschina
Francesca Faraci
Gianpaolo Ramelli
Vincenzo D’Apuzzo
Emmanuelle Rossini
Michela Papandrea
Year: 2020
Sensor Data Synchronization in a IoT Environment for Infants Motricity Measurement
HEALTHYIOT
Springer
DOI: 10.1007/978-3-030-42029-1_1
Abstract
Sensor data synchronization is a critical issue in the Internet of Things environments. In general, when a measurement environment includes different independent devices, it is paramount to ensure a global data consistency to a reference timestamp. Additionally, sensor nodes clocks are typically affected by environmental effects and by energy constraints which generate clock drifts. In this work, we present a specific Internet of Things architecture composed by seven Inertial Measurement Unit nodes, three Raspberry Pi 3, three video cameras and a laptop. In specific, we present an off-line data-driven synchronization solution which handles data of different nature and sampled at different frequencies. The solution solves both the data synchronization issue and the data-time alignment due to clock drift problems. The proposed methodology has been implemented and deployed within a measurement context involving infants (from 8 to 15 months old), within the scope of the AutoPlay project, whose goal is the analysis of infants ludic motricity data in order to possibly anticipate the identification of neurodevelopmental disorders.