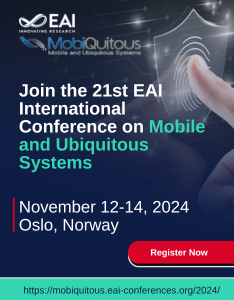
Research Article
Going Multi-viral: Synthedemic Modelling of Internet-based Spreading Phenomena
@ARTICLE{10.4108/icst.valuetools.2014.258221, author={Marily Nika and Thomas Wilding and Dieter Fiems and Koen De Turck and William Knottenbelt}, title={Going Multi-viral: Synthedemic Modelling of Internet-based Spreading Phenomena}, journal={EAI Endorsed Transactions on Ambient Systems}, volume={2}, number={7}, publisher={EAI}, journal_a={AMSYS}, year={2015}, month={2}, keywords={epidemiology, synthedemic modelling, spreading phenomena}, doi={10.4108/icst.valuetools.2014.258221} }
- Marily Nika
Thomas Wilding
Dieter Fiems
Koen De Turck
William Knottenbelt
Year: 2015
Going Multi-viral: Synthedemic Modelling of Internet-based Spreading Phenomena
AMSYS
EAI
DOI: 10.4108/icst.valuetools.2014.258221
Abstract
Epidemics of a biological and technological nature pervade modern life. For centuries, scientific research focused on biological epidemics, with simple compartmental epidemiological models emerging as the dominant explanatory paradigm. Yet there has been limited translation of this effort to explain internet-based spreading phenomena. Indeed, single-epidemic models are inadequate to explain the multimodal nature of complex phenomena. In this paper we propose a novel paradigm for modelling internet-based spreading phenomena based on the composition of multiple compartmental epidemiological models. Our approach is inspired by Fourier analysis, but rather than trigonometric wave forms, our components are compartmental epidemiological models. We show results on simulated multiple epidemic data, swine flu data and BitTorrent downloads of a popular music artist. Our technique can characterise these multimodal data sets utilising a parsimonous number of subepidemic models.
Copyright © 2015 M. Nika et al., licensed to EAI. This is an open access article distributed under the terms of the Creative Commons Attribution licence (http://creativecommons.org/licenses/by/3.0/), which permits unlimited use, distribution and reproduction in any medium so long as the original work is properly cited.