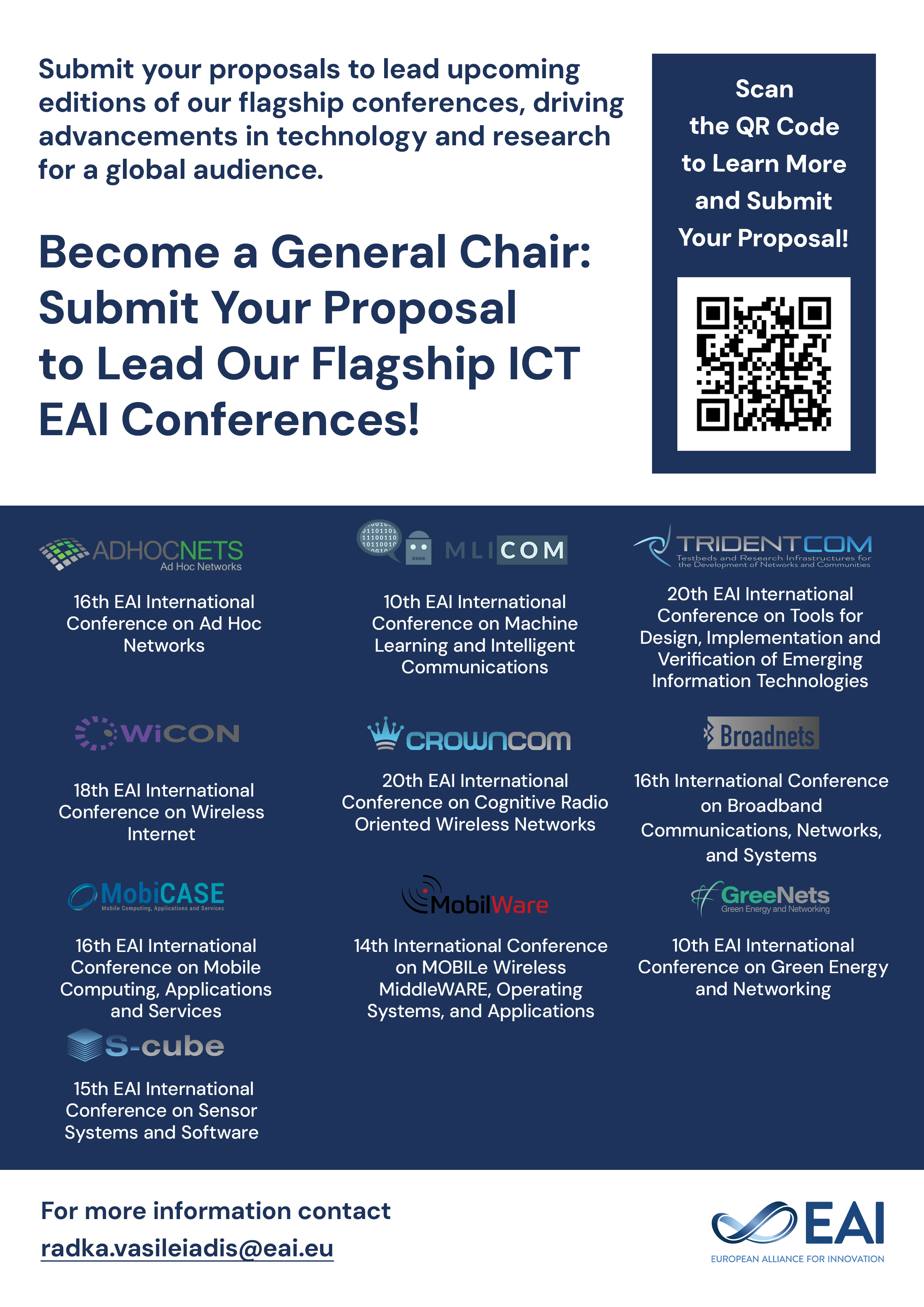
Research Article
Exploration vs Exploitation with Partially Observable Gaussian Autoregressive Arms
@ARTICLE{10.4108/icst.valuetools.2014.258207, author={Julia Kuhn and Michel Mandjes and Yoni Nazarathy}, title={Exploration vs Exploitation with Partially Observable Gaussian Autoregressive Arms}, journal={EAI Endorsed Transactions on Self-Adaptive Systems}, volume={1}, number={4}, publisher={EAI}, journal_a={SAS}, year={2015}, month={2}, keywords={restless bandits, partially observable, whittle index, performance evaluation, asymptotic dynamics}, doi={10.4108/icst.valuetools.2014.258207} }
- Julia Kuhn
Michel Mandjes
Yoni Nazarathy
Year: 2015
Exploration vs Exploitation with Partially Observable Gaussian Autoregressive Arms
SAS
EAI
DOI: 10.4108/icst.valuetools.2014.258207
Abstract
We consider a restless bandit problem with Gaussian autoregressive arms, where the state of an arm is only observed when it is played and the state-dependent reward is collected. Since arms are only partially observable, a good decision policy needs to account for the fact that information about the state of an arm becomes more and more obsolete while the arm is not being played. Thus, the decision maker faces a tradeoff between exploiting those arms that are believed to be currently the most rewarding (i.e. those with the largest conditional mean), and exploring arms with a high conditional variance. Moreover, one would like the decision policy to remain tractable despite the infinite state space and also in systems with many arms. A policy that gives some priority to exploration is the Whittle index policy, for which we establish structural properties. These motivate a parametric index policy that is computationally much simpler than the Whittle index but can still outperform the myopic policy. Furthermore, we examine the many-arm behavior of the system under the parametric policy, identifying equations describing its asymptotic dynamics. Based on these insights we provide a simple heuristic algorithm to evaluate the performance of index policies; the latter is used to optimize the parametric index.
Copyright © 2015 J. Kuhn et al., licensed to EAI. This is an open access article distributed under the terms of the Creative Commons Attribution licence (http://creativecommons.org/licenses/by/3.0/), which permits unlimited use, distribution and reproduction in any medium so long as the original work is properly cited.