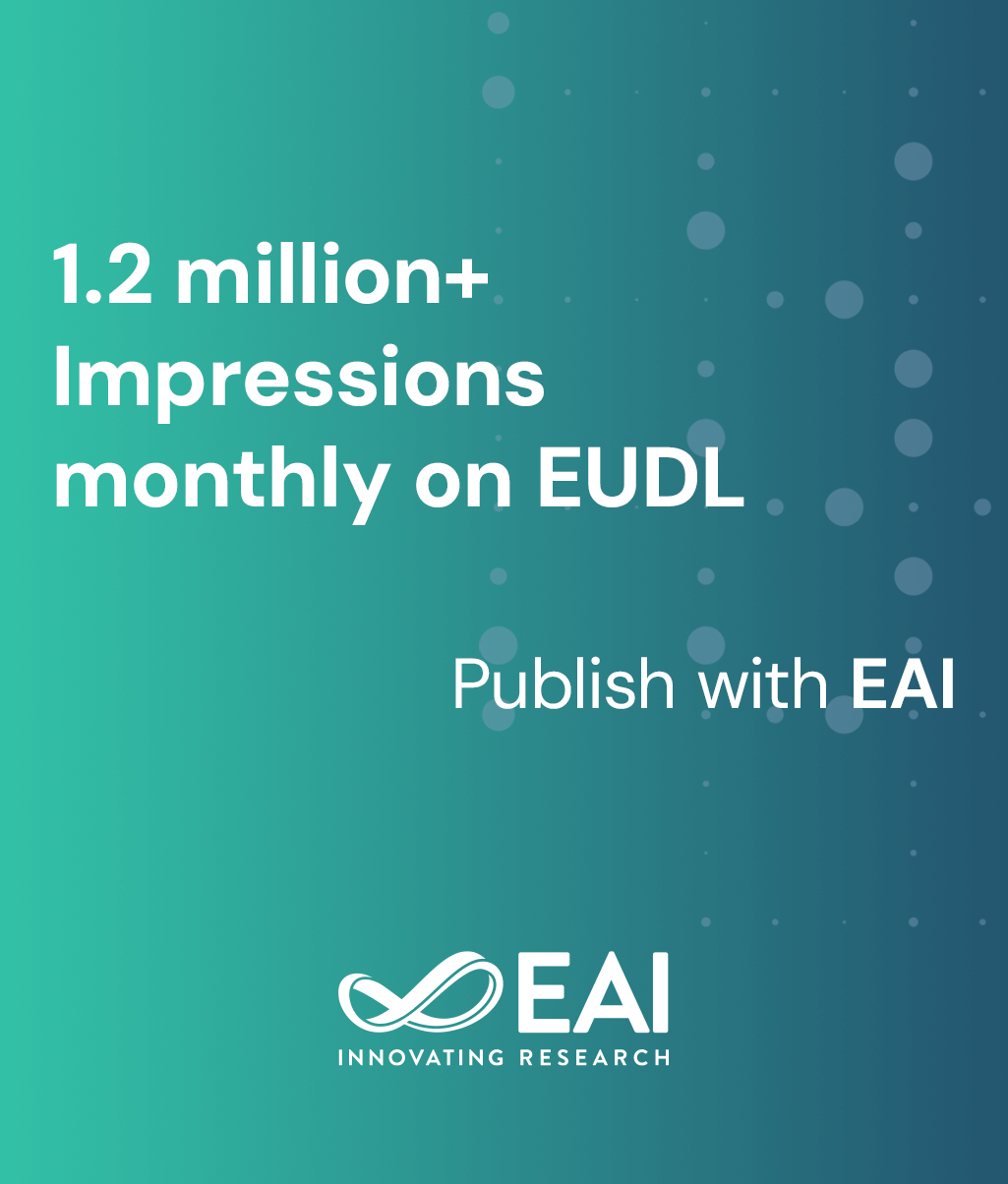
Research Article
Inferring Travel Purpose from Crowd-Augmented Human Mobility Data
@INPROCEEDINGS{10.4108/icst.urb-iot.2014.257173, author={Zack Zhu and Ulf Blanke and Gerhard Tr\o{}ster}, title={Inferring Travel Purpose from Crowd-Augmented Human Mobility Data}, proceedings={The First International Conference on IoT in Urban Space}, publisher={ACM}, proceedings_a={URB-IOT}, year={2014}, month={11}, keywords={crowd-generated urban data human mobility mining data fusion}, doi={10.4108/icst.urb-iot.2014.257173} }
- Zack Zhu
Ulf Blanke
Gerhard Tröster
Year: 2014
Inferring Travel Purpose from Crowd-Augmented Human Mobility Data
URB-IOT
ICST
DOI: 10.4108/icst.urb-iot.2014.257173
Abstract
Affordances from the urban space shape the way we interact with our environment, whether manifested as driving into the city centre for work or playing sports in designated arenas. Given today's abundance of crowd-generated digital traces on location-based social network (LBSN) platforms, an opportunity arises to grasp deeper semantic characterization of urban affordances beyond static representations found in traditional GIS systems. Complementing these perceptions of the city, travel surveys capture mobility dynamics of people with absolute trajectory recordings and explicit travel purposes. By marrying rich LBSN data with travel surveys, we ask if crowdsourced urban characteristics can be used to explain user behaviour when interacting with the city. Concretely, our objective is to model and infer the purpose of travel, or the activity at the destination of a trip, in daily life scenarios. To this end, we generate features to correspond to time, location, and demographics in order to construct a fused understanding of people's travel purposes. Using LBSN data to augment a travel survey of 87,600 trips by 10,372 people, we show that fusion of extracted features can achieve an interpersonal prediction accuracy of >75% for 9 broad classes of travel purposes covering typical aspects of life. This represents an increase of nearly 20% compared to without LBSN augmentation.