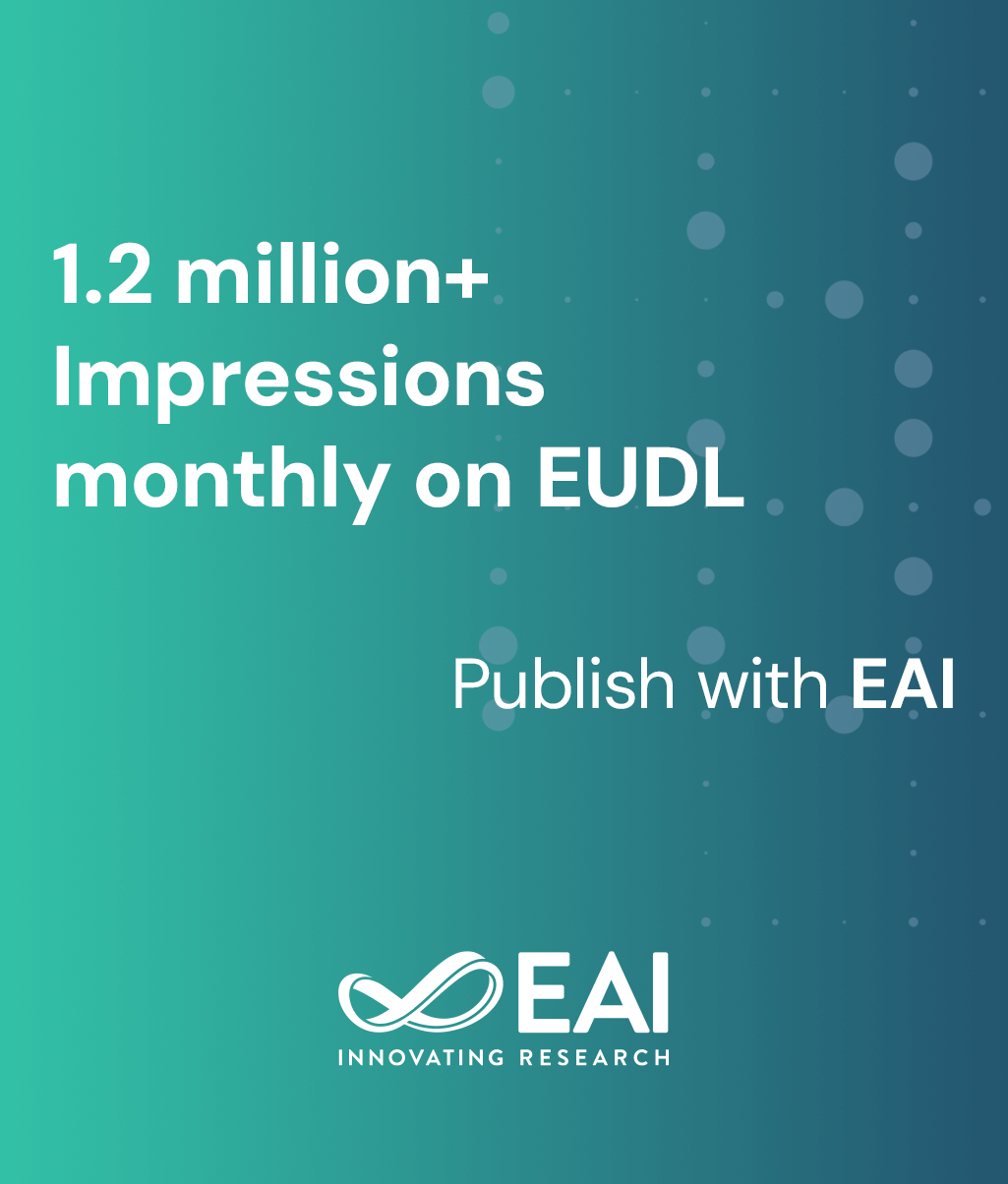
Research Article
Detecting affective states in virtual rehabilitation
@ARTICLE{10.4108/icst.pervasivehealth.2015.259250, author={Jes\^{u}s Rivas and Felipe Orihuela-Espina and L. Sucar and Lorena Palafox and Jorge Hern\^{a}ndez-Franco and Nadia Bianchi-Berthouze}, title={Detecting affective states in virtual rehabilitation}, journal={EAI Endorsed Transactions on Pervasive Health and Technology}, volume={1}, number={2}, publisher={IEEE}, journal_a={PHAT}, year={2015}, month={8}, keywords={affective computing, virtual rehabilitation, stroke, hand, motor recovery}, doi={10.4108/icst.pervasivehealth.2015.259250} }
- Jesús Rivas
Felipe Orihuela-Espina
L. Sucar
Lorena Palafox
Jorge Hernández-Franco
Nadia Bianchi-Berthouze
Year: 2015
Detecting affective states in virtual rehabilitation
PHAT
EAI
DOI: 10.4108/icst.pervasivehealth.2015.259250
Abstract
Virtual rehabilitation supports motor training following stroke by means of tailored virtual environments. To optimize therapy outcome, virtual rehabilitation systems automatically adapt to the different patients’ changing needs. Adaptation decisions should ideally be guided by both the observable performance and the hidden mind state of the user. We hypothesize that some affective aspects can be inferred from observable metrics. Here we present preliminary results of a classification exercise to decide on 4 states; tiredness, tension, pain and satisfaction. Descriptors of 3D hand movement and finger pressure were collected from 2 post-stroke participants while they practice on a virtual rehabilitation platform. Linear Support Vector Machine models were learnt to unfold a predictive relation between observation and the affective states considered. Initial results are promising (ROC Area under the curve (mean+/-std): 0.713 +/- 0.137). Confirmation of these opens the door to incorporate surrogates of mind state into the algorithm deciding on therapy adaptation.
Copyright © 2015 J. Rivas et al., licensed to EAI. This is an open access article distributed under the terms of the Creative Commons Attribution licence (http://creativecommons.org/licenses/by/3.0/), which permits unlimited use, distribution and reproduction in any medium so long as the original work is properly cited.