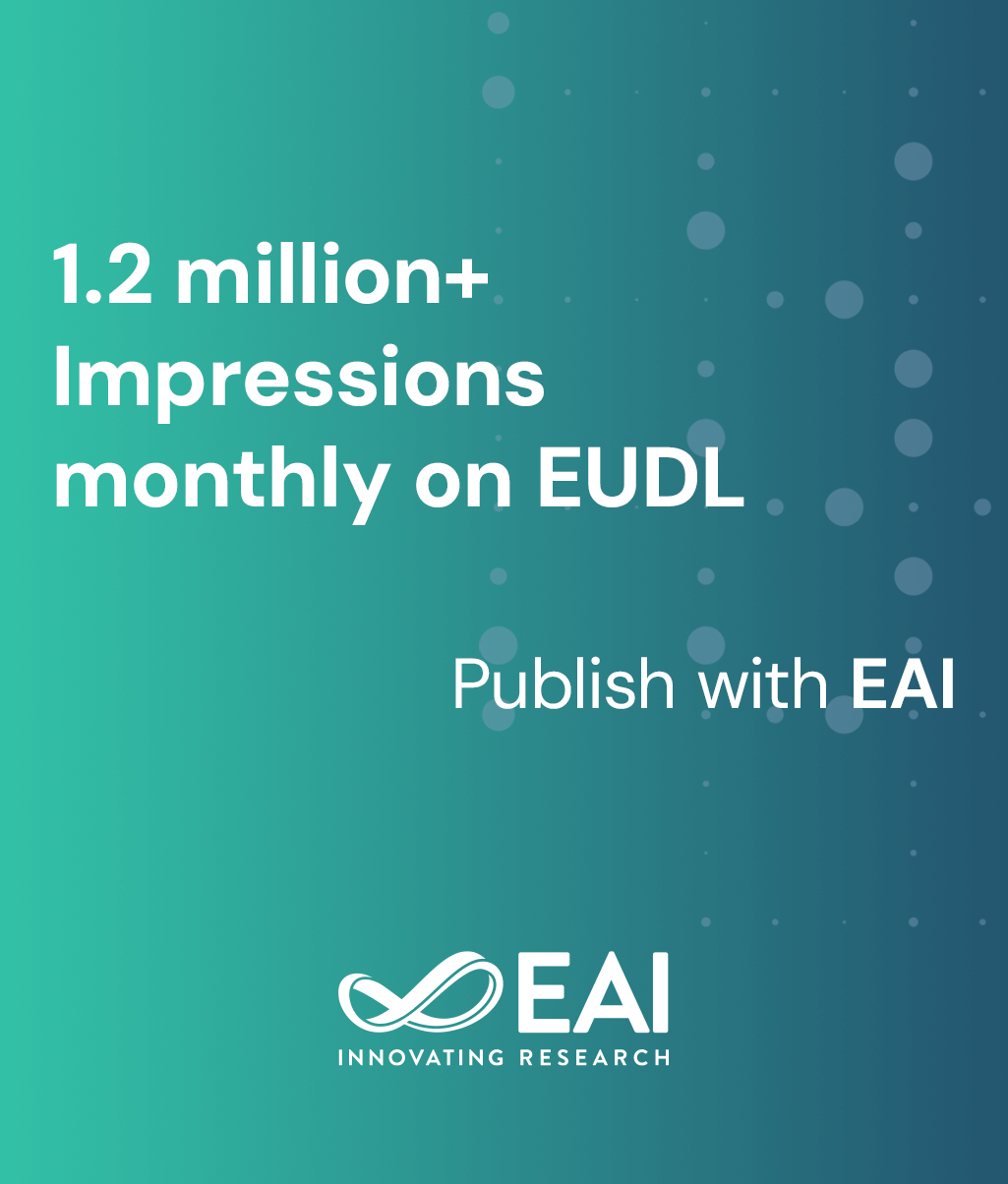
Research Article
Supporting Drug Prescription via Predictive and Personalized Query System
@INPROCEEDINGS{10.4108/icst.pervasivehealth.2015.259130, author={Samamon Khemmarat and Lixin Gao}, title={Supporting Drug Prescription via Predictive and Personalized Query System}, proceedings={9th International Conference on Pervasive Computing Technologies for Healthcare}, publisher={IEEE}, proceedings_a={PERVASIVEHEALTH}, year={2015}, month={8}, keywords={query system drug prescription personalization}, doi={10.4108/icst.pervasivehealth.2015.259130} }
- Samamon Khemmarat
Lixin Gao
Year: 2015
Supporting Drug Prescription via Predictive and Personalized Query System
PERVASIVEHEALTH
ICST
DOI: 10.4108/icst.pervasivehealth.2015.259130
Abstract
Drug prescription requires consideration of several factors, such as drug interactions and side effects. The process is further complicated by the fact that the presence of some drug properties, such as side effects, depends on patient characteristics, such as age and gender. Our goal is to provide a tool to assist medical practitioners in prescribing drugs. We develop an approach to query for drugs that satisfy a set of conditions based on drug properties. Furthermore, the approach tailors the answers to a given patient profile. We utilize drug information from multiple sources. However, data from these sources are usually noisy and incomplete as they are either manually curated or automatically extracted from text resources. To cope with incomplete and noisy data, our approach considers both the answers that exactly match and those that closely match the query. We represent drug information as a heterogeneous graph and model answering a query as a subgraph matching problem. To rank answers, our approach leverages the structure and the heterogeneity of the drug graph to quantify the likelihood of missing edges and integrates the likelihood into the scores of the answers. Our evaluation shows that for quantifying the edge likelihood, our graph-based approach can improve the AUROC (Area under Receiver Operating Characteristic) [1] by up to 40%, comparing to a baseline approach. We demonstrate the benefits of our system through several query examples.