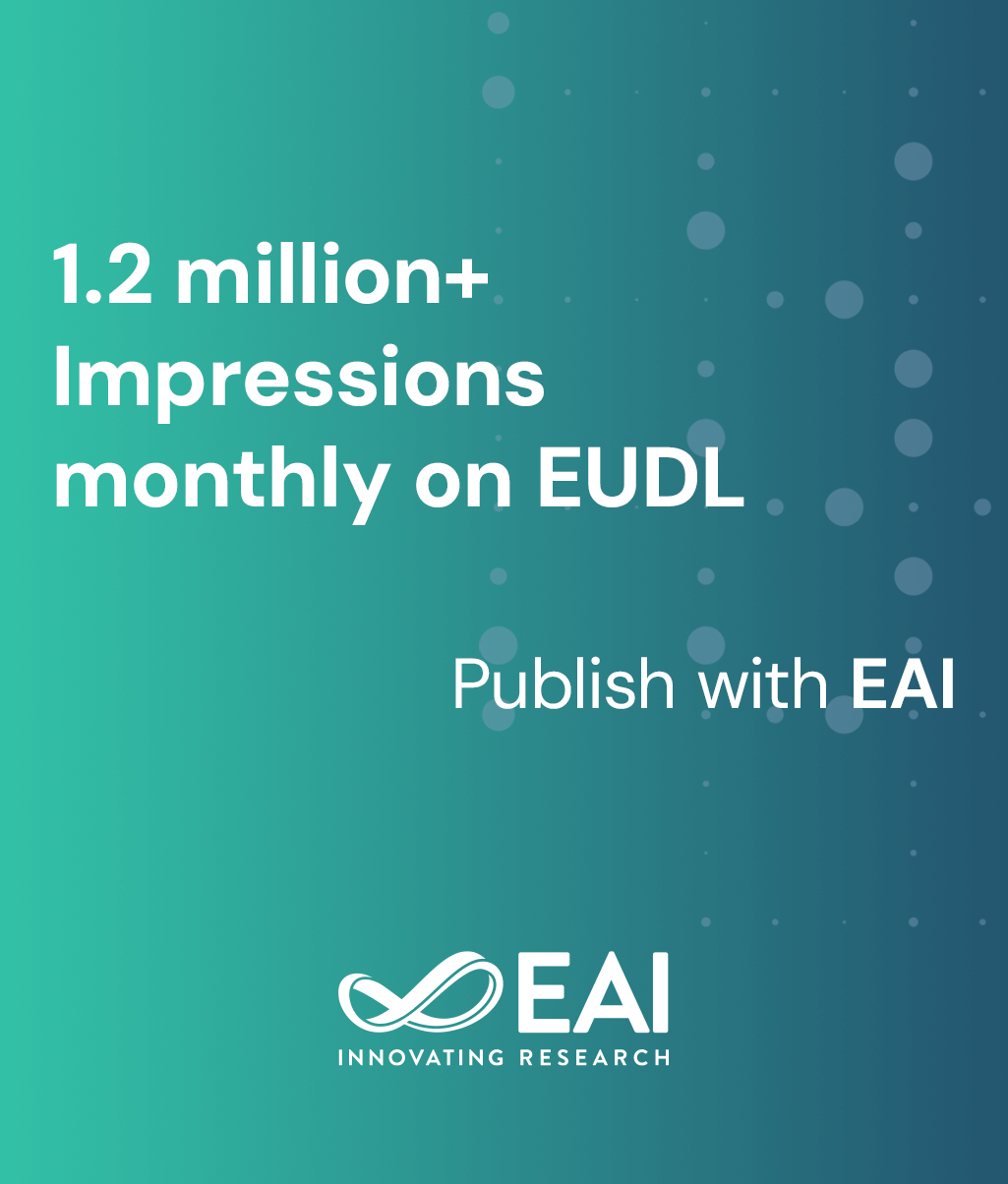
Research Article
Performance Evaluation of Decision Tree Classifiers for the Prediction of Response to treatment of Hepatitis C Patients
@INPROCEEDINGS{10.4108/icst.pervasivehealth.2014.254946, author={AbuBakr Awad and Mahasen Mabrouk and Awad Tahany and Naglaa Zayed and Sherif Mousa and Mohamed Saeed}, title={Performance Evaluation of Decision Tree Classifiers for the Prediction of Response to treatment of Hepatitis C Patients}, proceedings={8th International Conference on Pervasive Computing Technologies for Healthcare}, publisher={ICST}, proceedings_a={PERVASIVEHEALTH}, year={2014}, month={7}, keywords={afp gender decision tree c45 cart rep tree hcv pegylated interferon ribavirin}, doi={10.4108/icst.pervasivehealth.2014.254946} }
- AbuBakr Awad
Mahasen Mabrouk
Awad Tahany
Naglaa Zayed
Sherif Mousa
Mohamed Saeed
Year: 2014
Performance Evaluation of Decision Tree Classifiers for the Prediction of Response to treatment of Hepatitis C Patients
PERVASIVEHEALTH
ACM
DOI: 10.4108/icst.pervasivehealth.2014.254946
Abstract
This study included 2962 Egyptian patients with chronic hepatitis C virus (HCV) infection. Different decision-tree models were used to explore baseline predictors of response to Peginterferon plus Ribavirin therapy to discriminate HCV patients who are likely to respond. We have developed simple software that generates different possible combination of parameters for each model; using this software we were able to assort the decision-tree model according to the best performance, and to find the best classifier. The three models were comparable as regards to accuracy (about 69%); however REP Tree has shown fast and well performance for classification on medical data sets of increased size. Various pre-treatment decision tree algorithms have demonstrated that low level of Alpha-Fetal Protein (AFP) is associated with high response rate; and has the prospective to support clinical decisions regarding the proper selection of patients for therapy without imposing extra costs for additional examinations.