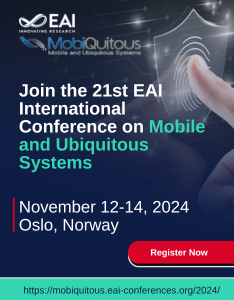
Research Article
Is "Frequency Distribution" Enough to Detect Tremor in PD Patients Using a Wrist Worn Accelerometer?
@INPROCEEDINGS{10.4108/icst.pervasivehealth.2014.254928, author={Claas Ahlrichs and Albert Sam\'{a}}, title={Is "Frequency Distribution" Enough to Detect Tremor in PD Patients Using a Wrist Worn Accelerometer?}, proceedings={8th International Conference on Pervasive Computing Technologies for Healthcare}, publisher={ICST}, proceedings_a={PERVASIVEHEALTH}, year={2014}, month={7}, keywords={pd parkinson's disease tremor at rest svm}, doi={10.4108/icst.pervasivehealth.2014.254928} }
- Claas Ahlrichs
Albert Samà
Year: 2014
Is "Frequency Distribution" Enough to Detect Tremor in PD Patients Using a Wrist Worn Accelerometer?
PERVASIVEHEALTH
ACM
DOI: 10.4108/icst.pervasivehealth.2014.254928
Abstract
This paper presents two approaches on detecting tremor in patients with Parkinson's Disease by means of a wrist-worn accelerometer. Both approaches are evaluated in terms of specificity and sensitivity as well as their applicability for a real-time implementation. One approach is solely based on the frequency distribution of a windowed time series, while the second approach utilizes commonly employed features found in the literature (e.g. FFT, entropy, peak frequency, correlation). The two algorithms detect tremor at rest in windowed time series. The effects of varying window lengths and detection thresholds are studied. The results indicate that an SVM with a linear kernel, in combination with the frequency distribution, may already be enough to accurately and reliably detect tremor in windowed time series. The approach, after being trained with a first dataset of signals obtained from 12 patients, achieved a sensitivity of 88.4% and specificity of 89.4% in a second dataset from 64 PD patients.