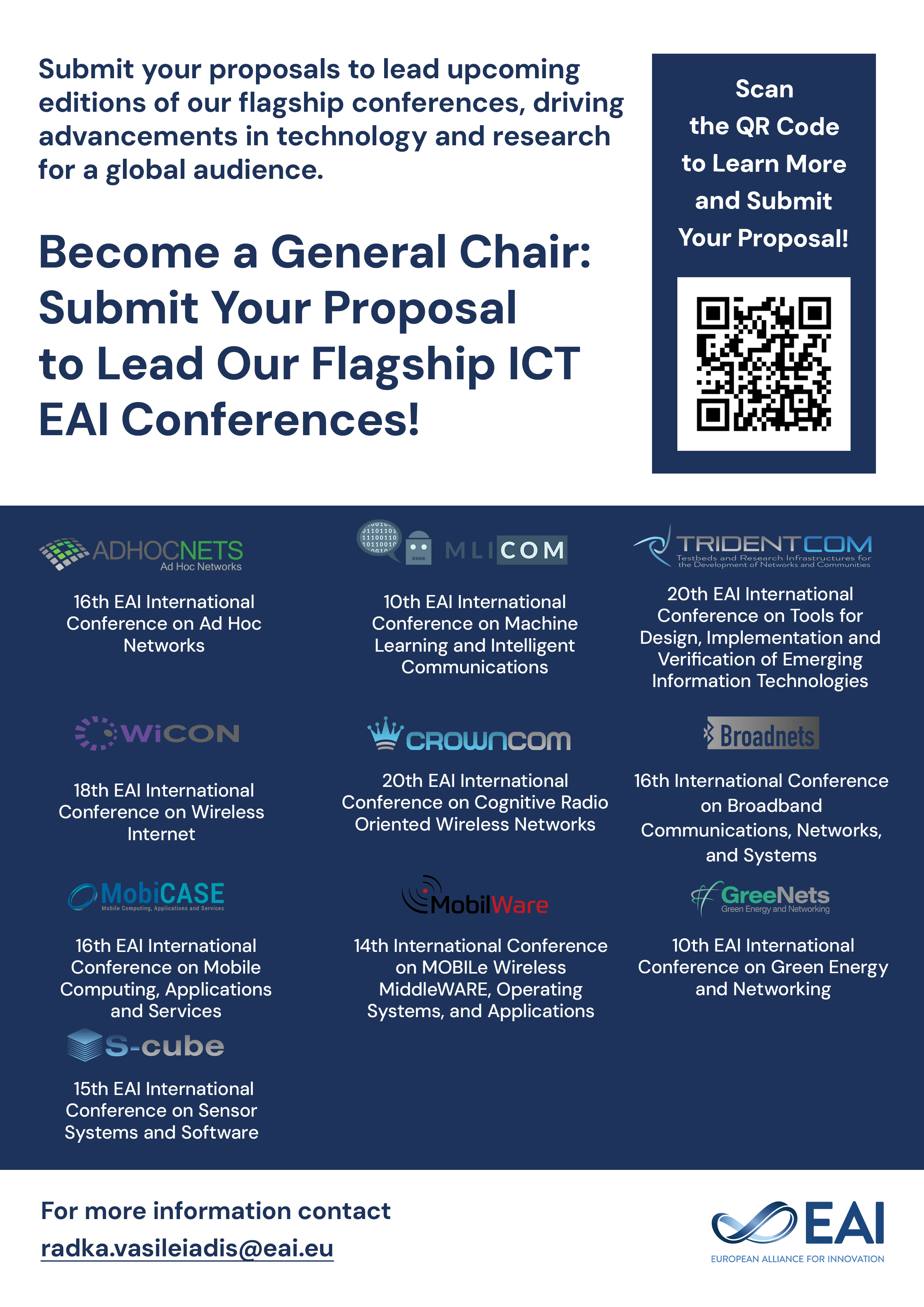
Research Article
AALO: Activity recognition in smart homes using Active Learning in the presence of Overlapped activities
@INPROCEEDINGS{10.4108/icst.pervasivehealth.2012.248600, author={Enamul Hoque and John Stankovic}, title={AALO: Activity recognition in smart homes using Active Learning in the presence of Overlapped activities}, proceedings={6th International Conference on Pervasive Computing Technologies for Healthcare}, publisher={IEEE}, proceedings_a={PERVASIVEHEALTH}, year={2012}, month={7}, keywords={activity recognition smart home data mining wireless sensors}, doi={10.4108/icst.pervasivehealth.2012.248600} }
- Enamul Hoque
John Stankovic
Year: 2012
AALO: Activity recognition in smart homes using Active Learning in the presence of Overlapped activities
PERVASIVEHEALTH
ICST
DOI: 10.4108/icst.pervasivehealth.2012.248600
Abstract
We present AALO: a novel Activity recognition system for single person smart homes using Active Learning in the presence of Overlapped activities. AALO applies data mining techniques to cluster in-home sensor firings so that each cluster represents instances of the same activity. Users only need to label each cluster as an activity as opposed to labeling all instances of all activities. Once the clusters are associated to their corresponding activities, our system can recognize future activities. To improve the activity recognition accuracy, our system preprocesses raw sensor data by identifying overlapping activities. The evaluation of activity recognition performance on a 26-day dataset shows that compared to Naive Bayesian (NB), Hidden Markov Model (HMM), and Hidden Semi Markov Model (HSMM) based activity recognition systems, our average time slice error (24.15%) is much lower than NB (53.04%), and similar to HMM (29.97%) and HSMM (26.29%). Thus, our active learning based approach performs as good as the state of the art supervised techniques (HMM and HSMM).