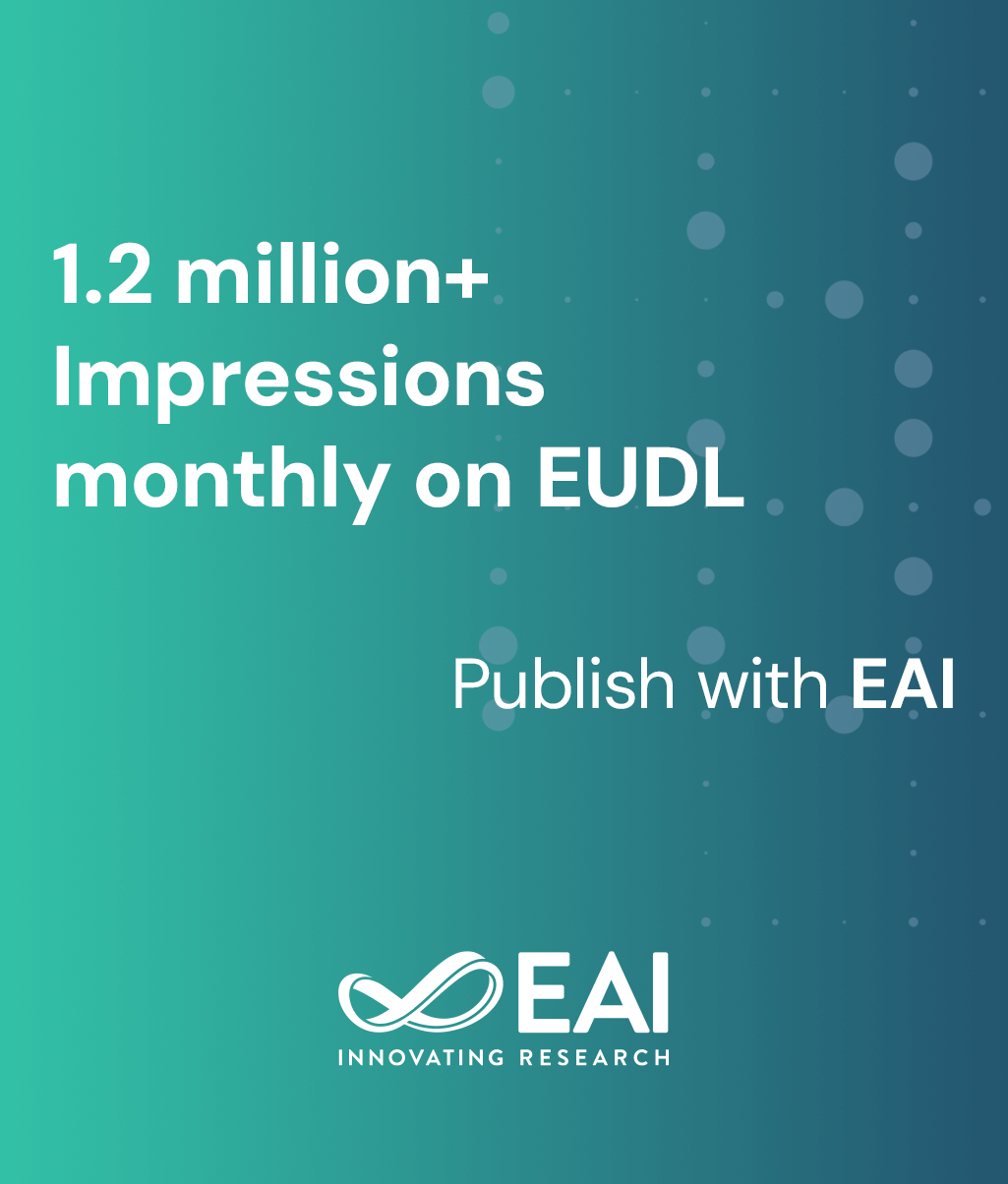
Research Article
PEAR: Power Efficiency through Activity Recognition (for ECG-based Sensing)
@INPROCEEDINGS{10.4108/icst.pervasivehealth.2011.246037, author={Feng-Tso Sun and Cynthia Kuo and Martin Griss}, title={PEAR: Power Efficiency through Activity Recognition (for ECG-based Sensing)}, proceedings={5th International ICST Conference on Pervasive Computing Technologies for Healthcare}, publisher={IEEE}, proceedings_a={PERVASIVEHEALTH}, year={2012}, month={4}, keywords={Activity Recognition Energy Efficiency Electrocardiogram Body Sensor Network}, doi={10.4108/icst.pervasivehealth.2011.246037} }
- Feng-Tso Sun
Cynthia Kuo
Martin Griss
Year: 2012
PEAR: Power Efficiency through Activity Recognition (for ECG-based Sensing)
PERVASIVEHEALTH
ICST
DOI: 10.4108/icst.pervasivehealth.2011.246037
Abstract
The PEAR framework is presented using an ECG-based body sensor network as a case study. PEAR addresses real-world challenges in continuously monitoring physiological signals. PEAR leverages a wearable sensor’s embedded processing power to conserve energy. This is accomplished by performing some data processing on the sensor and reducing the overhead of wireless data transmission. A coarse-grained decision tree-based activity classifier was implemented on a sensor node to recognize the sensor wearer’s activity level. Using the wearer’s activity level, the sensor dynamically manages its activities – sampling of the ECG sensor, processing of the data, and wireless transmission – to minimize overall power consumption. This paper describes the design and implementation of RR interval extraction and activity recognition modules on a sensor node. An activity-aware energy model is presented along with energy profiling results. The energy conservation level varies with a wearer’s level of activity, and a sensitivity analysis shows that PEAR’s advantage over standard body sensor network architectures increases with more activity. In a user study, our participants were active 18%-28% of the time. Based on this level of activity, our implementation of PEAR increases battery life up to 2.5 times when compared to conventional ECG sensing approaches. This approach is applicable to a broad range of pervasive health applications that incorporate continuous monitoring of physiological signals.