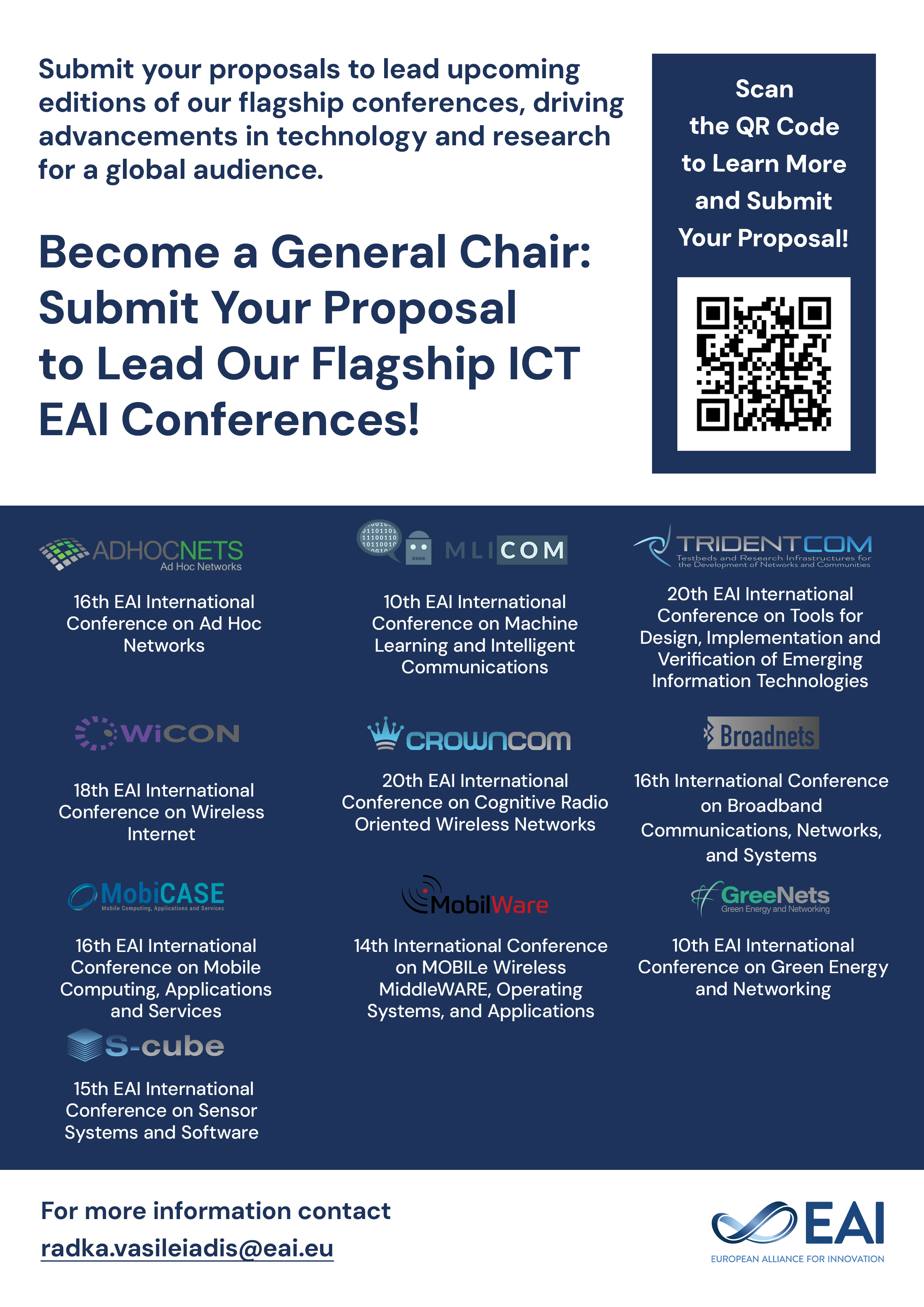
Research Article
Automatic Fall Detection Based on Doppler Radar Motion Signature
@INPROCEEDINGS{10.4108/icst.pervasivehealth.2011.245993, author={Liu Liang and Mihail Popescu and Marjorie Skubic and Marilyn Rantz and Tarik Yardibi and Paul Cuddihy}, title={Automatic Fall Detection Based on Doppler Radar Motion Signature}, proceedings={5th International ICST Conference on Pervasive Computing Technologies for Healthcare}, publisher={IEEE}, proceedings_a={PERVASIVEHEALTH}, year={2012}, month={4}, keywords={fall detection eldercare MFCC features radar classification SVM kNN}, doi={10.4108/icst.pervasivehealth.2011.245993} }
- Liu Liang
Mihail Popescu
Marjorie Skubic
Marilyn Rantz
Tarik Yardibi
Paul Cuddihy
Year: 2012
Automatic Fall Detection Based on Doppler Radar Motion Signature
PERVASIVEHEALTH
ICST
DOI: 10.4108/icst.pervasivehealth.2011.245993
Abstract
Falling is a common health problem for elderly. It is reported that more than one third of adults 65 and older fall each year in the United States. To address the problem, we are currently developing a Doppler radar-based fall detection system. Doppler radar sensors provide an inexpensive way to recognize human activity. In this paper, we employed mel-frequency cepstral coefficients (MFCC) to represent the Doppler signatures of various human activities such as walking, bending down, falling, etc. Then we used two different classifiers, SVM and kNN, to automatically detect falls based on the extracted MFCC features. We obtained encouraging classification results on a pilot dataset that contained 109 falls and 341 non-fall human activities.