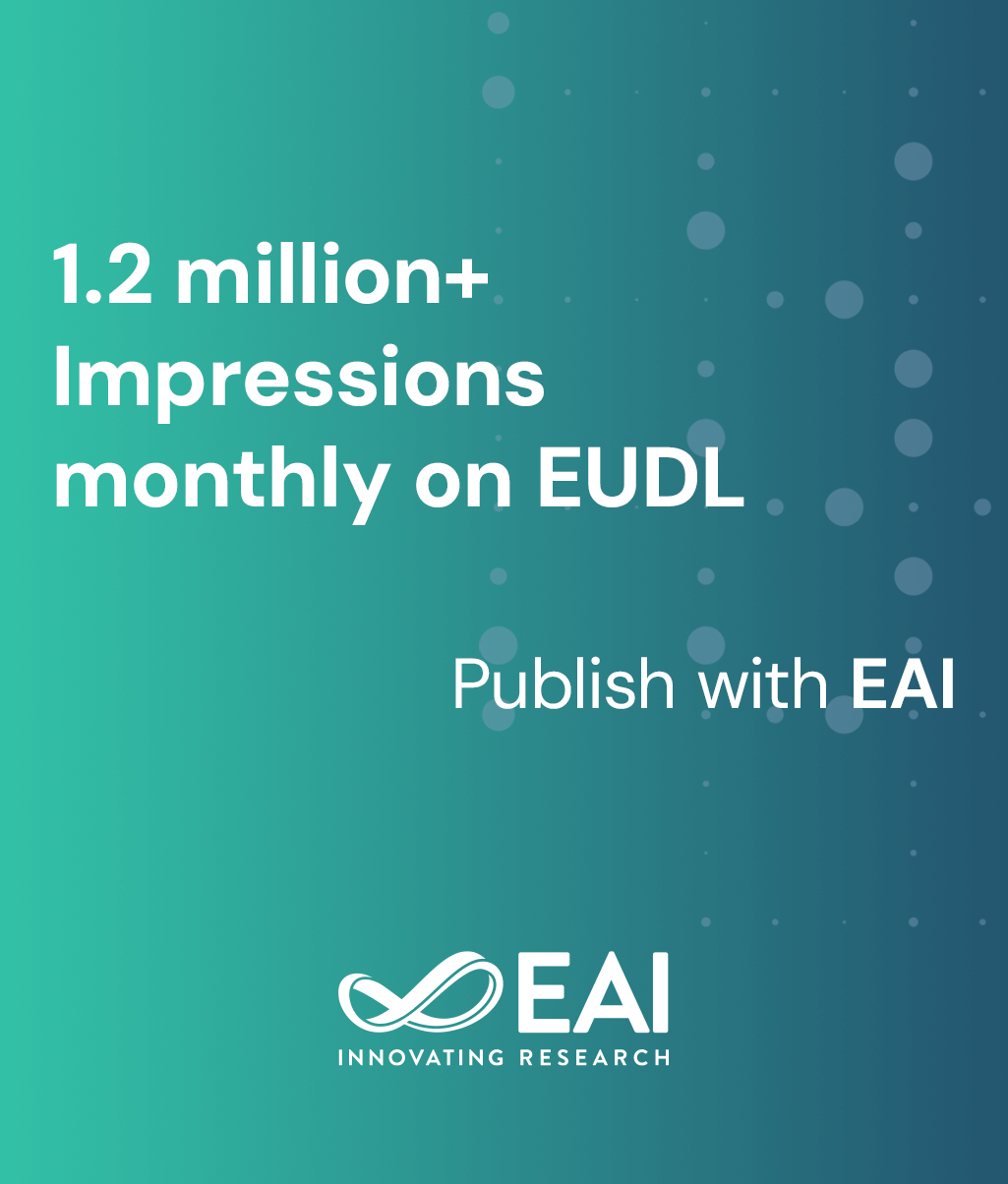
Research Article
Mining Emerging Patterns for recognizing activities of multiple users in pervasive computing
@INPROCEEDINGS{10.4108/ICST.MOBIQUITOUS2009.6818, author={Tao Gu and Zhanqing Wu and Liang Wang and Xianping Tao and Jian Lu}, title={Mining Emerging Patterns for recognizing activities of multiple users in pervasive computing}, proceedings={6th Annual International Conference on Mobile and Ubiquitous Systems: Computing, Networking and Services}, publisher={IEEE}, proceedings_a={MOBIQUITOUS}, year={2009}, month={11}, keywords={Computer science Humans Intelligent sensors Laboratories Mathematics Pattern recognition Performance analysis Pervasive computing Sensor phenomena and characterization Smart homes}, doi={10.4108/ICST.MOBIQUITOUS2009.6818} }
- Tao Gu
Zhanqing Wu
Liang Wang
Xianping Tao
Jian Lu
Year: 2009
Mining Emerging Patterns for recognizing activities of multiple users in pervasive computing
MOBIQUITOUS
IEEE
DOI: 10.4108/ICST.MOBIQUITOUS2009.6818
Abstract
Understanding and recognizing human activities from sensor readings is an important task in pervasive computing. Existing work on activity recognition mainly focuses on recognizing activities for a single user in a smart home environment. However, in real life, there are often multiple inhabitants live in such an environment. Recognizing activities of not only a single user, but also multiple users is essential to the development of practical context-aware applications in pervasive computing. In this paper, we investigate the fundamental problem of recognizing activities for multiple users from sensor readings in a home environment, and propose a novel pattern mining approach to recognize both single-user and multi-user activities in a unified solution. We exploit emerging pattern -a type of knowledge pattern that describes significant changes between classes of data - for constructing our activity models, and propose an emerging pattern based multi-user activity recognizer (epMAR) to recognize both single-user and multiuser activities. We conduct our empirical studies by collecting real-world activity traces done by two volunteers over a period of two weeks in a smart home environment, and analyze the performance in detail with respect to various activity cases in a multi-user scenario. Our experimental results demonstrate that our epMAR recognizer achieves an average accuracy of 89.72% for all the activity cases.