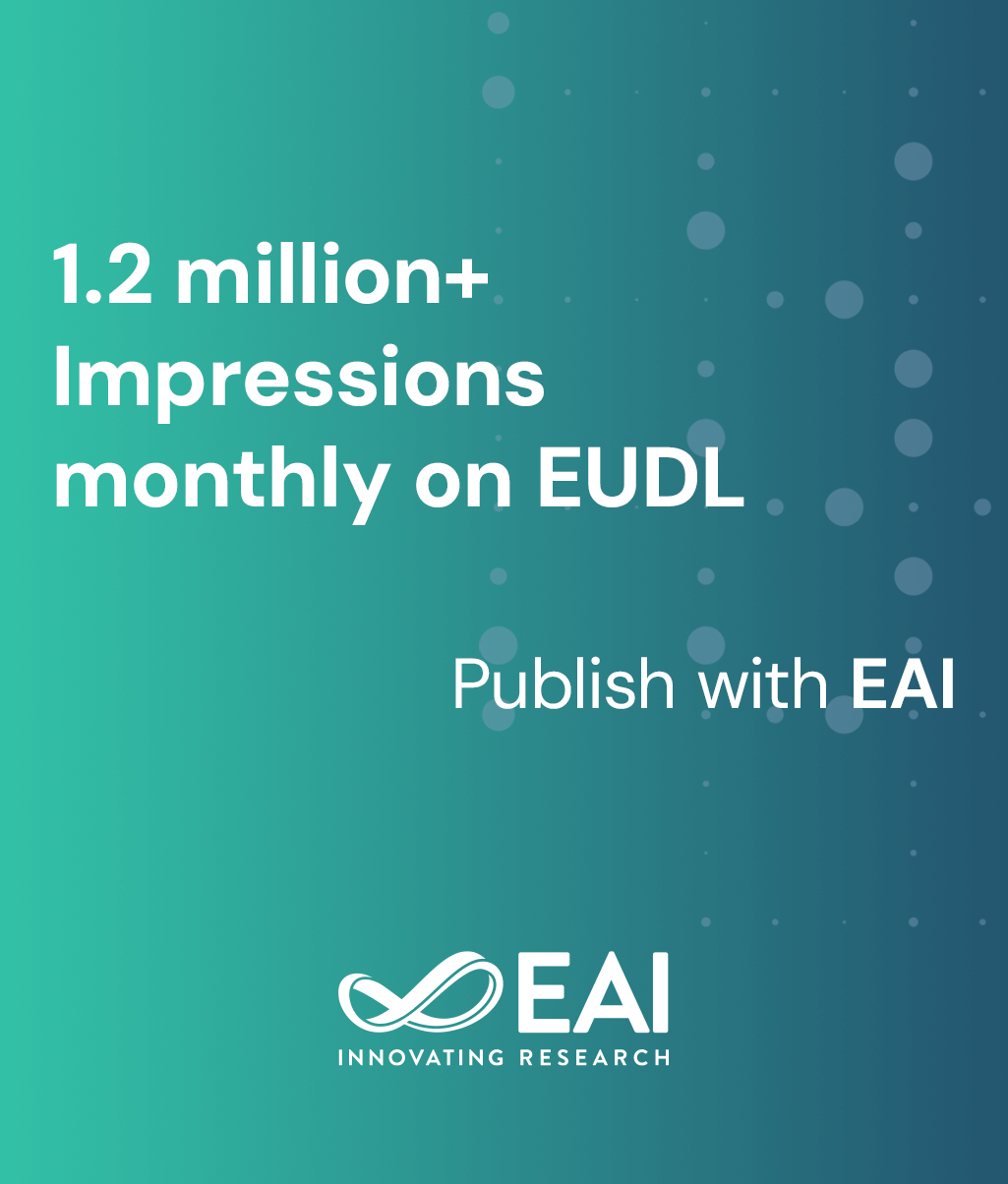
Research Article
Predicting Network Availability Using User Context
@INPROCEEDINGS{10.4108/ICST.MOBIQUITOUS2008.3563, author={Upendra Rathnayake and Max Ott}, title={Predicting Network Availability Using User Context}, proceedings={5th International ICST Conference on Mobile and Ubiquitous Systems: Computing, Networking and Services}, publisher={ICST}, proceedings_a={MOBIQUITOUS}, year={2010}, month={5}, keywords={Network Availability Prediction Context Prediction Semi Markov Model}, doi={10.4108/ICST.MOBIQUITOUS2008.3563} }
- Upendra Rathnayake
Max Ott
Year: 2010
Predicting Network Availability Using User Context
MOBIQUITOUS
ICST
DOI: 10.4108/ICST.MOBIQUITOUS2008.3563
Abstract
Most mobile devices nowadays can simultaneously connect to different access networks with different characteristics at different times. Most solutions proposed for such an environment are reactive in nature. For example, when networks are encountered, the device performs a vertical handover to the network that offers the highest bandwidth. But the cost of handover may not be justified if that network is only available for a short time. Knowledge of future network availability and its capabilities would help to proactively handle the handover process more intelligently. Network availability prediction is often addressed as user path predictions with network coverage maps. In contrast, we model it as a more robust context prediction problem that can use any of the available context variables like GSM cell ID, WLAN AP, whether the power cable plugged, number of people around etc. Specifically, we propose a Semi-Markovian context prediction model to predict WLAN availability. As collecting and processing context consumes power, we propose a method to rank each context variable according to their contributions to prediction accuracy. We also employ the same method for optimizing model parameters. Real user data collected in our experiments show that when WLAN status is static, prediction errors are nearly zero and even in changing environments, error is less than 26% on average and the obtained context variable ranking is realistic