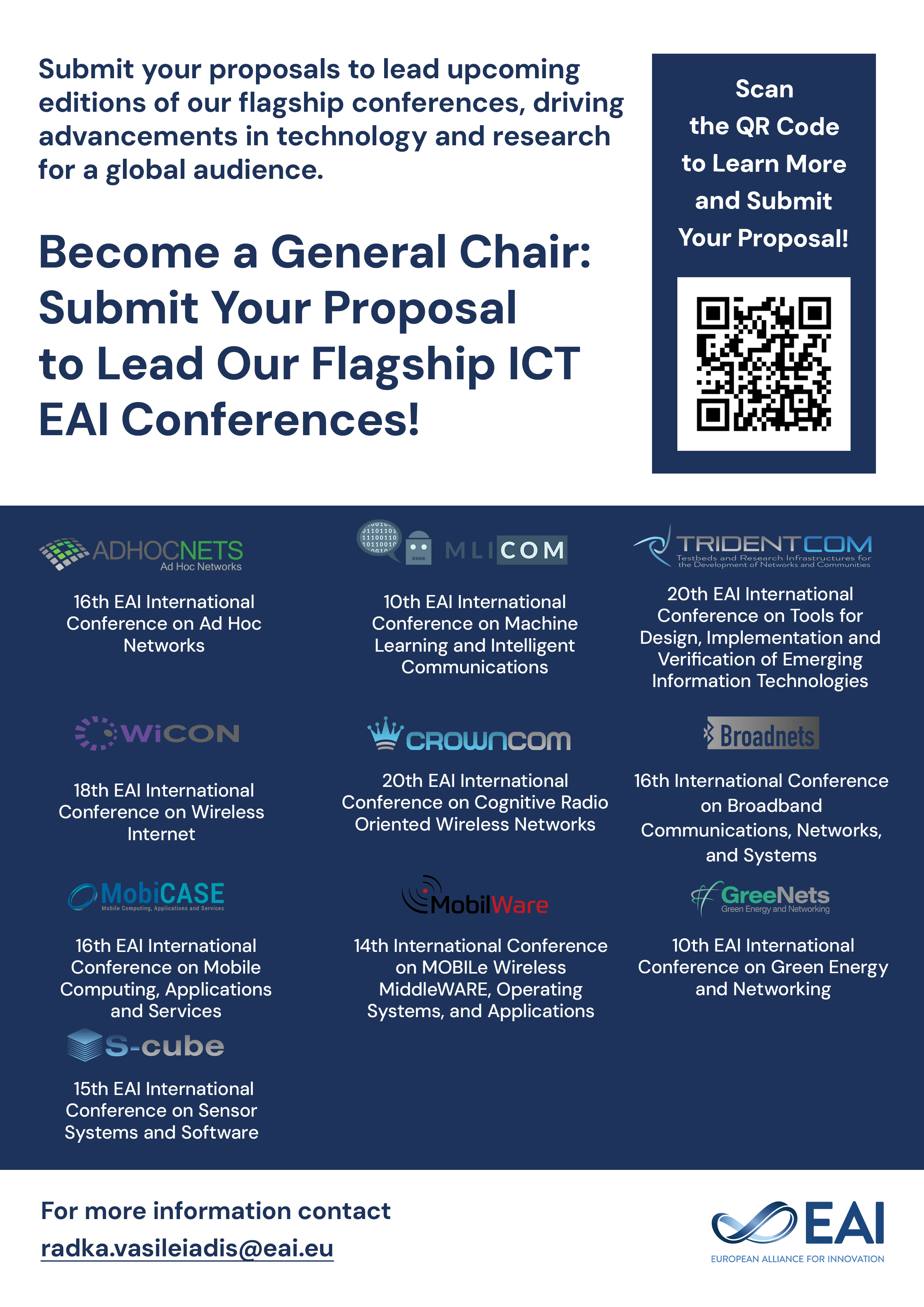
Research Article
Dual Scheme Phone: A User Assisted Mechanism to Effectively Run Sensor Analytics Applications on Smartphones
@INPROCEEDINGS{10.4108/icst.mobiquitous.2014.258081, author={Swarnava Dey and Arijit Mukherjee and Pubali Datta and Himadri S Paul and Anupam Basu}, title={Dual Scheme Phone: A User Assisted Mechanism to Effectively Run Sensor Analytics Applications on Smartphones}, proceedings={11th International Conference on Mobile and Ubiquitous Systems: Computing, Networking and Services}, publisher={ICST}, proceedings_a={MOBIQUITOUS}, year={2014}, month={11}, keywords={smartphone resource prediction machine learning usage pattern}, doi={10.4108/icst.mobiquitous.2014.258081} }
- Swarnava Dey
Arijit Mukherjee
Pubali Datta
Himadri S Paul
Anupam Basu
Year: 2014
Dual Scheme Phone: A User Assisted Mechanism to Effectively Run Sensor Analytics Applications on Smartphones
MOBIQUITOUS
ICST
DOI: 10.4108/icst.mobiquitous.2014.258081
Abstract
In recent times, many human-centric applications are being developed to leverage the diverse range of sensors present in Android smartphones. Smartphones are also being used as edge network gateways for fusing data from multiple sensors. These applications often run as background services and usually obtain and process sensor data. However, smartphones are traditionally optimized for prioritizing foreground and interactive applications and the user remains unaware about the resource-starved background applications, some of which may be important to him. In this work we present the argument in favour of optimizing the smartphones for background services too with a certain degree of user intervention. We exploit the user specific unique usage pattern of smartphones and show that machine learning techniques can be applied to predict CPU usage of applications. Based on the system-wide and app-specific predicted values of CPU usage, our system detects if any preferred background service is resource-starved and alerts the user accordingly. We show with an example sensor analytics application, that the accuracy of data collection and analysis of that application increase significantly by applying our scheme. We further show that irrespective of variations in the accuracy of prediction due to users, handsets and algorithms used, reasonable period of forecast about future resource usage is possible using historical data about the applications under execution and different context parameters including time of day, day of week, network cell, location, accelerometer data.