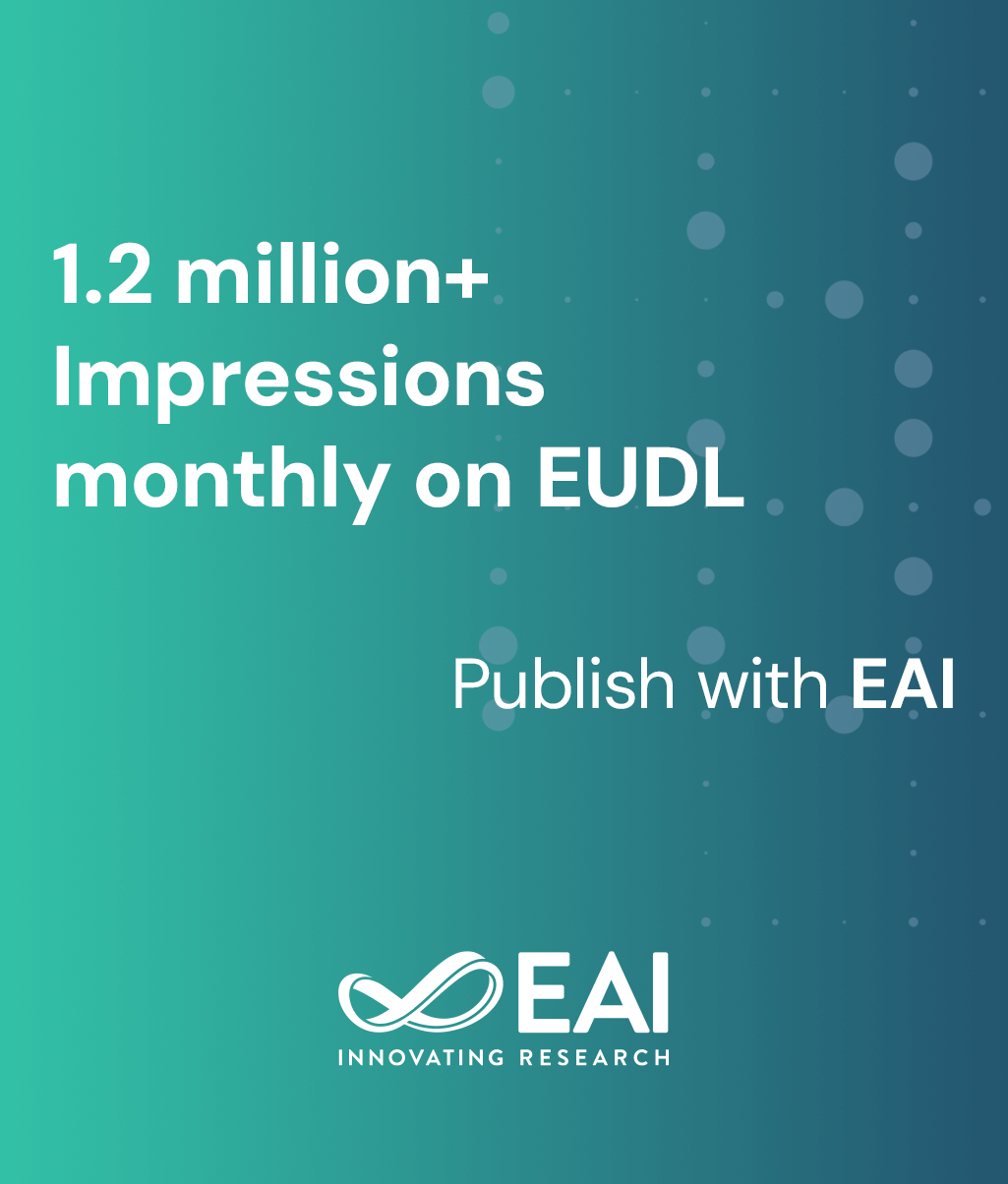
Research Article
Can Smart Plugs Predict Electric Power Consumption? A Case Study
@INPROCEEDINGS{10.4108/icst.mobiquitous.2014.257991, author={Andreas Reinhardt and Delphine Christin and Salil Kanhere}, title={Can Smart Plugs Predict Electric Power Consumption? A Case Study}, proceedings={11th International Conference on Mobile and Ubiquitous Systems: Computing, Networking and Services}, publisher={ICST}, proceedings_a={MOBIQUITOUS}, year={2014}, month={11}, keywords={distributed appliance metering power consumption prediction smart buildings smart grids}, doi={10.4108/icst.mobiquitous.2014.257991} }
- Andreas Reinhardt
Delphine Christin
Salil Kanhere
Year: 2014
Can Smart Plugs Predict Electric Power Consumption? A Case Study
MOBIQUITOUS
ICST
DOI: 10.4108/icst.mobiquitous.2014.257991
Abstract
The Internet of Things will encompass a rich variety of sensing systems including mobile phones, embedded sensor and actuator platforms, and even smart electricity meters. Through their collaborative operation, billions of such devices will realize the vision of smart homes, smart cities, and beyond. Smart electricity meters can, e.g., already help utilities monitor the stability of the power grid by periodically reporting each connected household’s energy consumption. Even more sophisticated services can be realized when data is available at higher spatio-temporal resolutions, e.g., when distributed smart power meters (sometimes referred to as smart plugs) are deployed. In this paper, we present one such novel service for the smart home, namely making projections of an appliance’s future energy demand based on previously observed power consumption data. In a first step, our system identifies and isolates unique characteristic signatures from collected power consumption traces. Subsequently, time series pattern matching is applied to detect these signatures in real-time data. Based on the occurrences of the extracted signatures in real-time data, the appliance’s future power demand is predicted. We evaluate our approach with more than 2,500 appliance activity segments collected from 15 different appliance types, and show that accurate forecasts can be made in many cases.