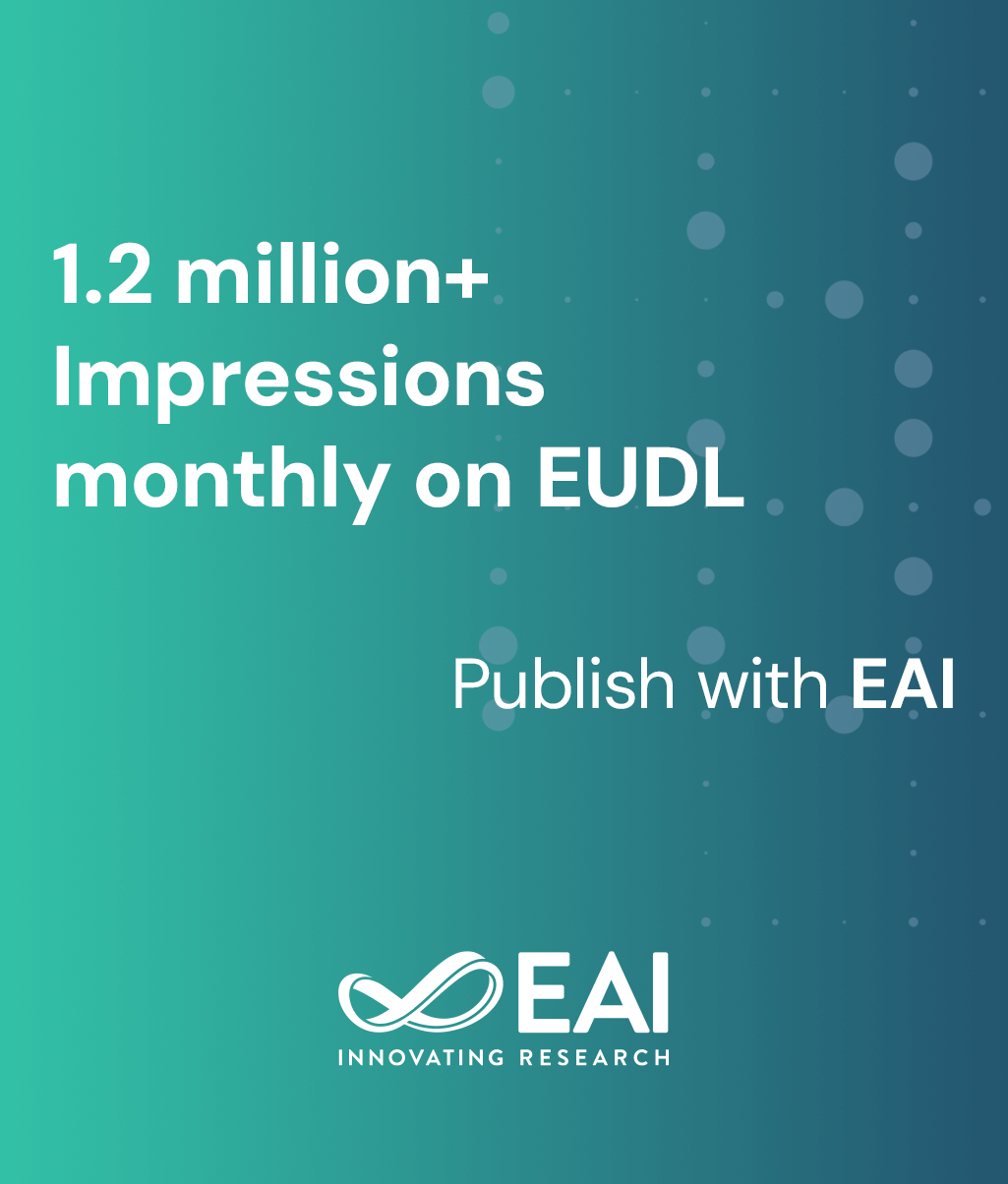
Research Article
VividhaVahana: Smartphone Based Vehicle Classification And Its Applications in Developing Regions
@INPROCEEDINGS{10.4108/icst.mobiquitous.2014.257982, author={Shilpa Garg and Pushpendra Singh and Parameswaran Ramanathan and Rijurekha Sen}, title={VividhaVahana: Smartphone Based Vehicle Classification And Its Applications in Developing Regions}, proceedings={11th International Conference on Mobile and Ubiquitous Systems: Computing, Networking and Services}, publisher={ICST}, proceedings_a={MOBIQUITOUS}, year={2014}, month={11}, keywords={mobile sensing machine learning traffic vehicle}, doi={10.4108/icst.mobiquitous.2014.257982} }
- Shilpa Garg
Pushpendra Singh
Parameswaran Ramanathan
Rijurekha Sen
Year: 2014
VividhaVahana: Smartphone Based Vehicle Classification And Its Applications in Developing Regions
MOBIQUITOUS
ICST
DOI: 10.4108/icst.mobiquitous.2014.257982
Abstract
Developing region road traffic has a unique characteristic of high heterogeneity in vehicle types. In this paper, we describe VividhaVahana, a smartphone sensor based system to categorize road vehicles into four predominant categories: two-wheeler bikes, three-wheeler auto-rickshaws, four-wheeler cars and public transport like buses. Using a variety of sensor based features, our system is able to achieve above 90% classification accuracy, evaluated over 1500+ Km of driving data, on two urban road stretches in the Indian city of Delhi. We also apply VividhaVahana to empirically examine four representative transport applications, namely travel time estimation, driving behavior detection, traffic state classification and road surface monitoring. We show how each of these applications would benefit from a vehicle class specific analysis, compared to the vehicle agnostic analysis as has been done in the past. Our work gives useful insights on how such applications can be re-designed, to better fit developing region traffic characteristics and requirements.