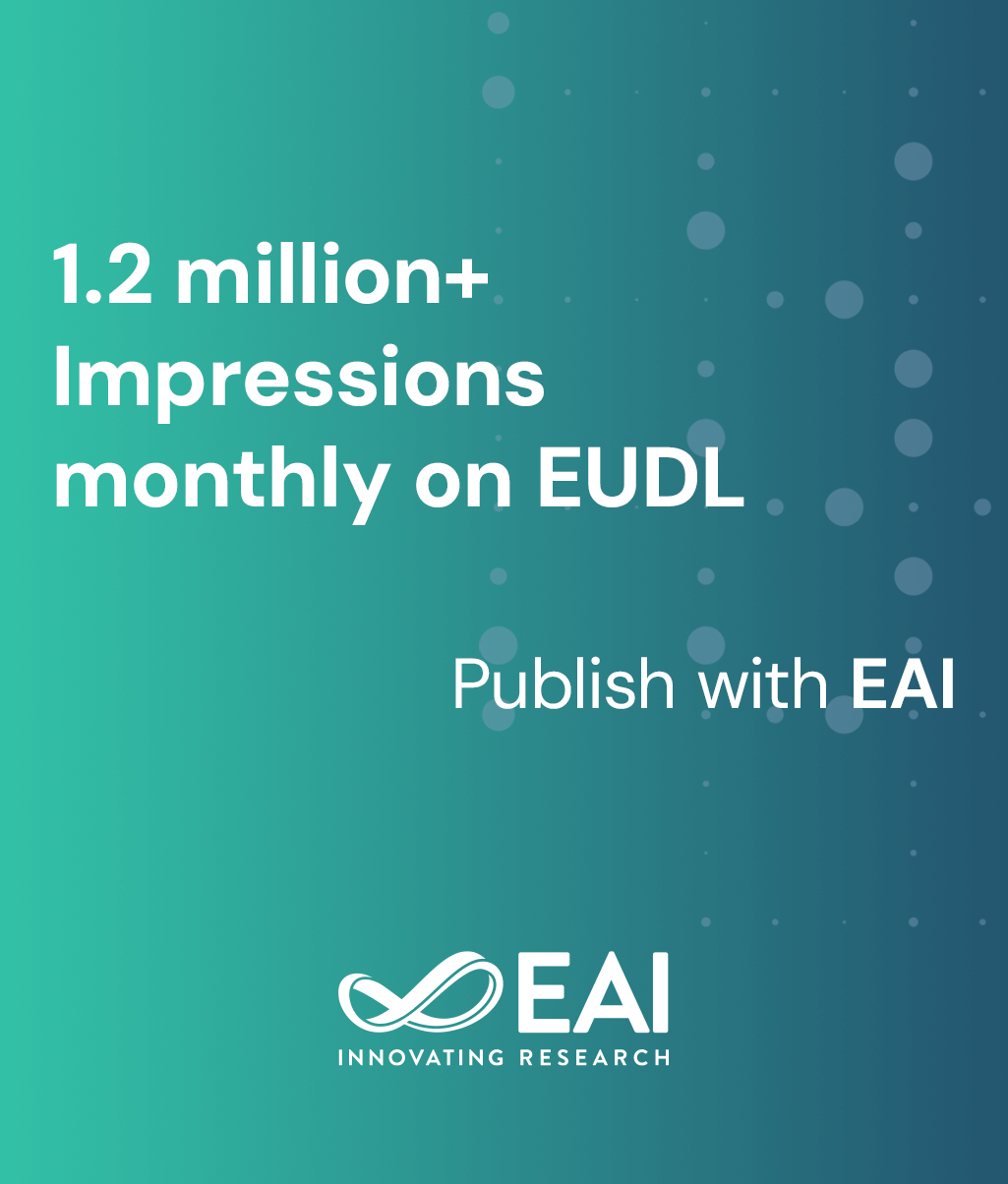
Research Article
A Method for Estimating Hunger Degree based on Meal and Exercise Logs
@INPROCEEDINGS{10.4108/icst.mobihealth.2014.257389, author={Isamu Sugita and Morihiko Tamai and Yutaka Arakawa and Keiichi Yasumoto}, title={A Method for Estimating Hunger Degree based on Meal and Exercise Logs}, proceedings={4th International Conference on Wireless Mobile Communication and Healthcare - "Transforming healthcare through innovations in mobile and wireless technologies"}, publisher={IEEE}, proceedings_a={MOBIHEALTH}, year={2014}, month={12}, keywords={hunger degree estimation blood glucose level estimation non-invasive method meal and exercise information machine learning mobile application}, doi={10.4108/icst.mobihealth.2014.257389} }
- Isamu Sugita
Morihiko Tamai
Yutaka Arakawa
Keiichi Yasumoto
Year: 2014
A Method for Estimating Hunger Degree based on Meal and Exercise Logs
MOBIHEALTH
IEEE
DOI: 10.4108/icst.mobihealth.2014.257389
Abstract
If temporal variation of a person's hunger degree could be estimated, it would be possible to adjust his/her eating habits and/or prevent obesity. It is well-known that there is a negative correlation between a hunger degree and a blood glucose level. However, it is hard to measure a person's blood glucose level anytime and anywhere, because it relies usually on an invasive method (e.g., blood sampling). This paper proposes a method for estimating a person's hunger degree in a non-invasive way. Our proposed method is composed of (1) a blood glucose level estimation model based on logs of meals and exercises, and (2) a hunger degree estimation model based on the estimated glucose level. The former model is constructed by correlating an actual blood glucose level and logs of meals and exercises with a machine learning technique. Here, the actual blood glucose level is measured by a commercial blood glucose meter invasively. The latter model is constructed by associating the measured blood glucose level with a subjective hunger degree. We also design and develop a mobile application for facilitating a user to easily record meals and exercises information. Through an experiment with a subject, we confirmed that our system can estimate a blood glucose level within about 14% mean percentage error and finally estimate hunger degree within about 1.3 levels mean error among 10 levels.