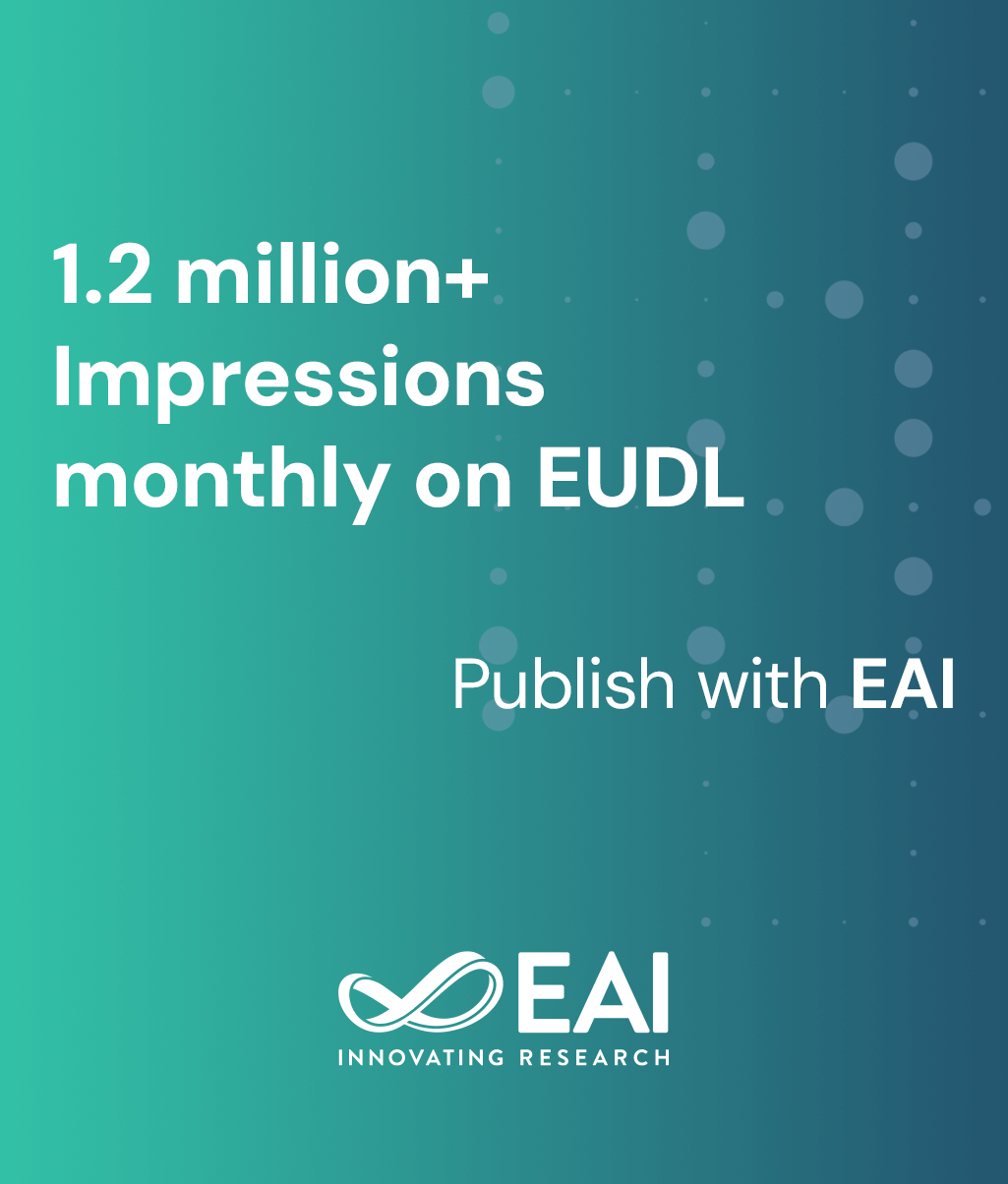
Research Article
Using Kinect for Assesing the state of Multiple Sclerosis patients
@INPROCEEDINGS{10.4108/icst.mobihealth.2014.257348, author={Dimitris Kastanitois and Gerasimos Kartsakalis and George Economou and Spiros Fotopoulos and Panagiotis Papathanasopoulos}, title={Using Kinect for Assesing the state of Multiple Sclerosis patients}, proceedings={4th International Conference on Wireless Mobile Communication and Healthcare - "Transforming healthcare through innovations in mobile and wireless technologies"}, publisher={IEEE}, proceedings_a={MOBIHEALTH}, year={2014}, month={12}, keywords={kinect multiple sclerosis gait analysis}, doi={10.4108/icst.mobihealth.2014.257348} }
- Dimitris Kastanitois
Gerasimos Kartsakalis
George Economou
Spiros Fotopoulos
Panagiotis Papathanasopoulos
Year: 2014
Using Kinect for Assesing the state of Multiple Sclerosis patients
MOBIHEALTH
IEEE
DOI: 10.4108/icst.mobihealth.2014.257348
Abstract
In this work a prototype video-based system for assessing the state of patients with Multiple sclerosis is proposed. In particular we introduce an automated system for capturing and analyzing gait sequences from patients performing the well known 2-minute walking test. The contribution of this work is twofold. First we provide a computerized approach for performing the 2-minute walk test and showing that there is a great correlation between the estimated by the system walking distance and the distance measured by the physicians (Pearson’s Rho= 0.7292, p<0.001). Second we present some preliminary results indicating that extracted gait style information is able to differentiate between healthy controls (HC) and Multiple Sclerosis patients even when the Extended Disability Status Scale (EDSS) is low. In order to exploit style information we first incorporated a view invariant representation of the skeleton. Then we represented a selected set of limbs using the Euler Angles and we mapped the sequences of features into the dissimilarity space achieving fixed length representation. Classification performed using Linear Discriminant Analysis resulted into an 88.2% correct classification rate. For our experiments we used a total of 9 MS and 8 HC matched in gender and age.