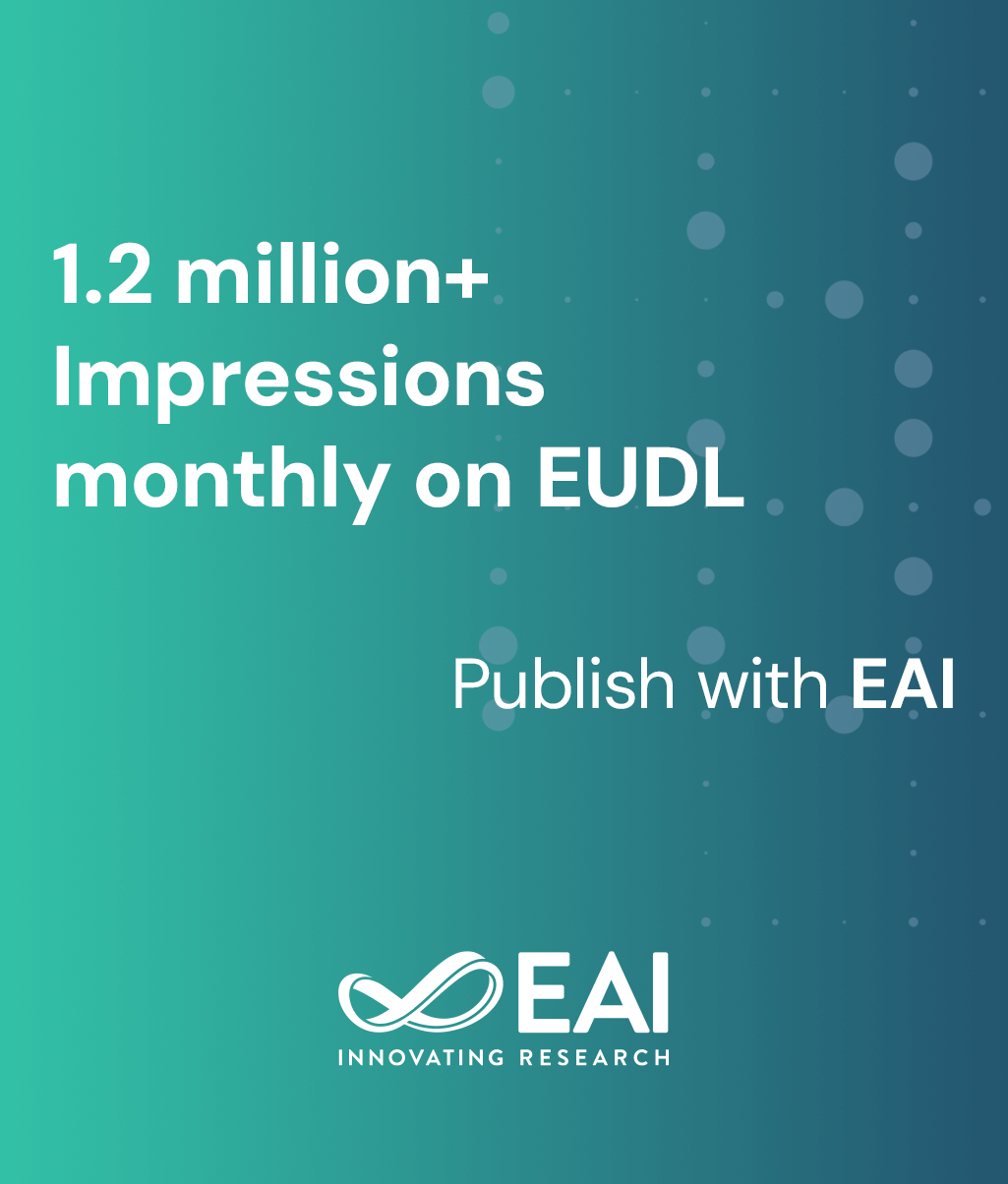
Research Article
What is this place? Inferring place categories through user patterns identification in geo-tagged tweets
@INPROCEEDINGS{10.4108/icst.mobicase.2014.257683, author={Deborah Falcone and Cecilia Mascolo and Carmela Comito and Domenico Talia and Jon Crowcroft}, title={What is this place? Inferring place categories through user patterns identification in geo-tagged tweets}, proceedings={6th International Conference on Mobile Computing, Applications and Services}, publisher={IEEE}, proceedings_a={MOBICASE}, year={2014}, month={11}, keywords={social networks semantic place labels location-based services}, doi={10.4108/icst.mobicase.2014.257683} }
- Deborah Falcone
Cecilia Mascolo
Carmela Comito
Domenico Talia
Jon Crowcroft
Year: 2014
What is this place? Inferring place categories through user patterns identification in geo-tagged tweets
MOBICASE
IEEE
DOI: 10.4108/icst.mobicase.2014.257683
Abstract
Online social networks (e.g., Facebook, Twitter) have started allowing users to tag their posts with geographical coordinates collected through the GPS interface of users smartphones. While this information is quite useful, it lacks some semantics about the type of place the user is (e.g., museum, school), which would allow a better understanding of users' patterns. While some location based online social network (e.g., Foursquare) allow users to tag the places they visit, this is not an automated process but one which requires the user help.
In this paper we exploit the dynamics of human activity to associate categories to GPS coordinates of social posts. We have collected geo-tagged tweets of London through Twitter. A supervised learning framework takes the tweets spatio-temporal features and determines human dynamics which we use to infer the place category. Our results show that the prediction framework is able to accurately identify if a place is of a certain category given its user activity patterns. The average accuracy is about 70%, reaching the highest accuracy for work (90%) and educational places (80%). Moreover the framework identifies the place category,with an accuracy up to 66%, finding out where people eat and drink, go for entertainment, or work/study.