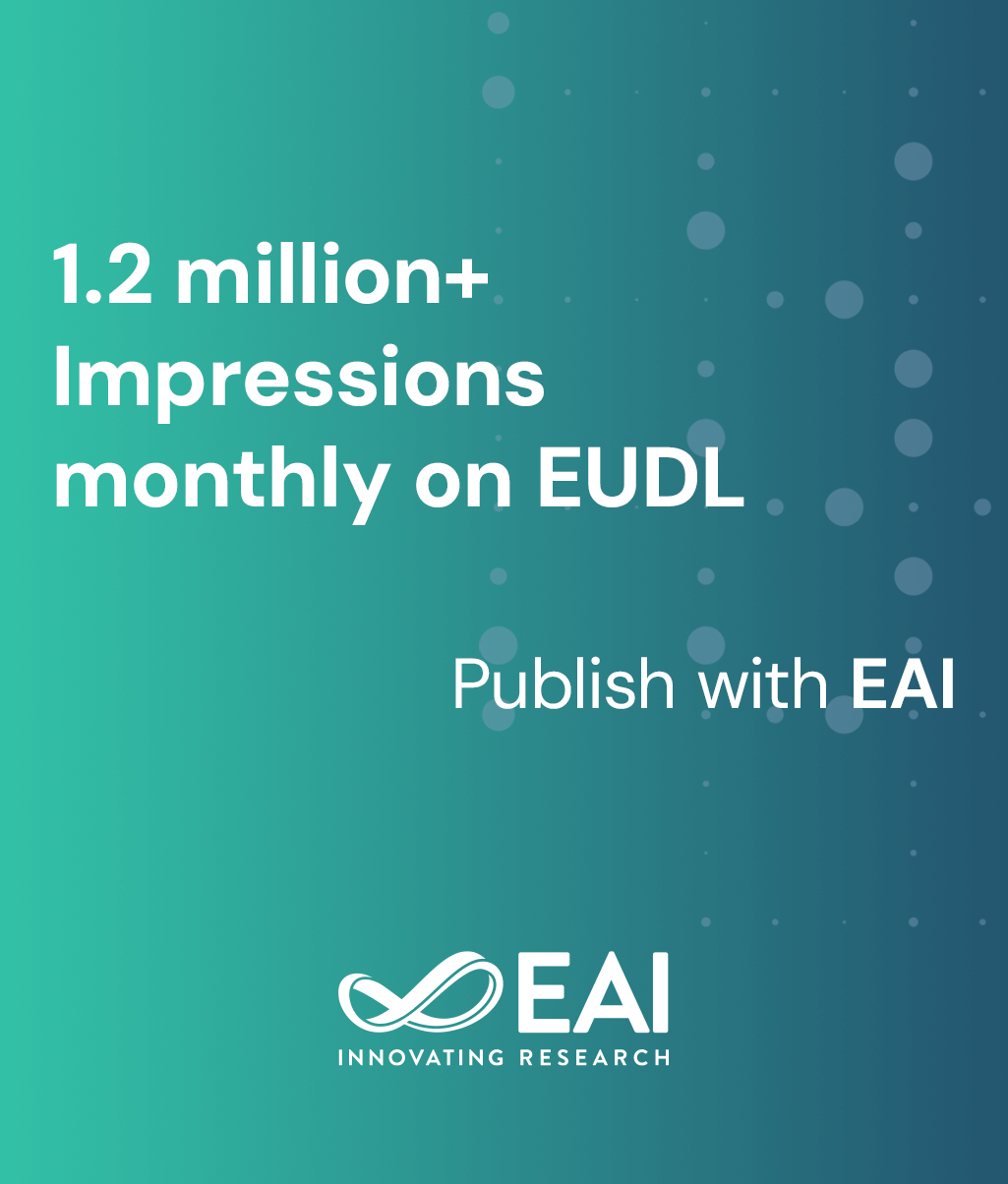
Research Article
Multiuser Automatic Modulation Classification for Cognitive Radios using Distributed Sensing in Multipath Fading Channels
@INPROCEEDINGS{10.4108/icst.crowncom.2012.248319, author={Munawwar Sohul and Barathram Ramkumar and Tamal Bose}, title={Multiuser Automatic Modulation Classification for Cognitive Radios using Distributed Sensing in Multipath Fading Channels}, proceedings={7th International Conference on Cognitive Radio Oriented Wireless Networks}, publisher={IEEE}, proceedings_a={CROWNCOM}, year={2012}, month={7}, keywords={cognitive radio multiuser amc distributed classification higher order cumulants multipath fading}, doi={10.4108/icst.crowncom.2012.248319} }
- Munawwar Sohul
Barathram Ramkumar
Tamal Bose
Year: 2012
Multiuser Automatic Modulation Classification for Cognitive Radios using Distributed Sensing in Multipath Fading Channels
CROWNCOM
IEEE
DOI: 10.4108/icst.crowncom.2012.248319
Abstract
Automatic Modulation Classifier (AMC) is an important component of a Cognitive Radio (CR) architecture that helps in better utilization of the spectrum. The successful implementation and employment of various cognitive radio services are largely dependent on the modulation classification performance of the cognitive radio terminals. AMC in literature is mostly developed for classifying the signal transmitted by a single user. Multiuser AMC, as the name suggests, simultaneously classifies signals transmitted by multiple users, while existing approaches rely on centralized processing. In this paper, we propose a fourth order cumulant based multiuser distributed AMC that can perform well even in a multipath fading environment. The proposed algorithm uses spatially distributed CRs cooperating with each other, which results in improved classification performance. Simulation results are provided to illustrate the promising results yielded by the proposed algorithm.