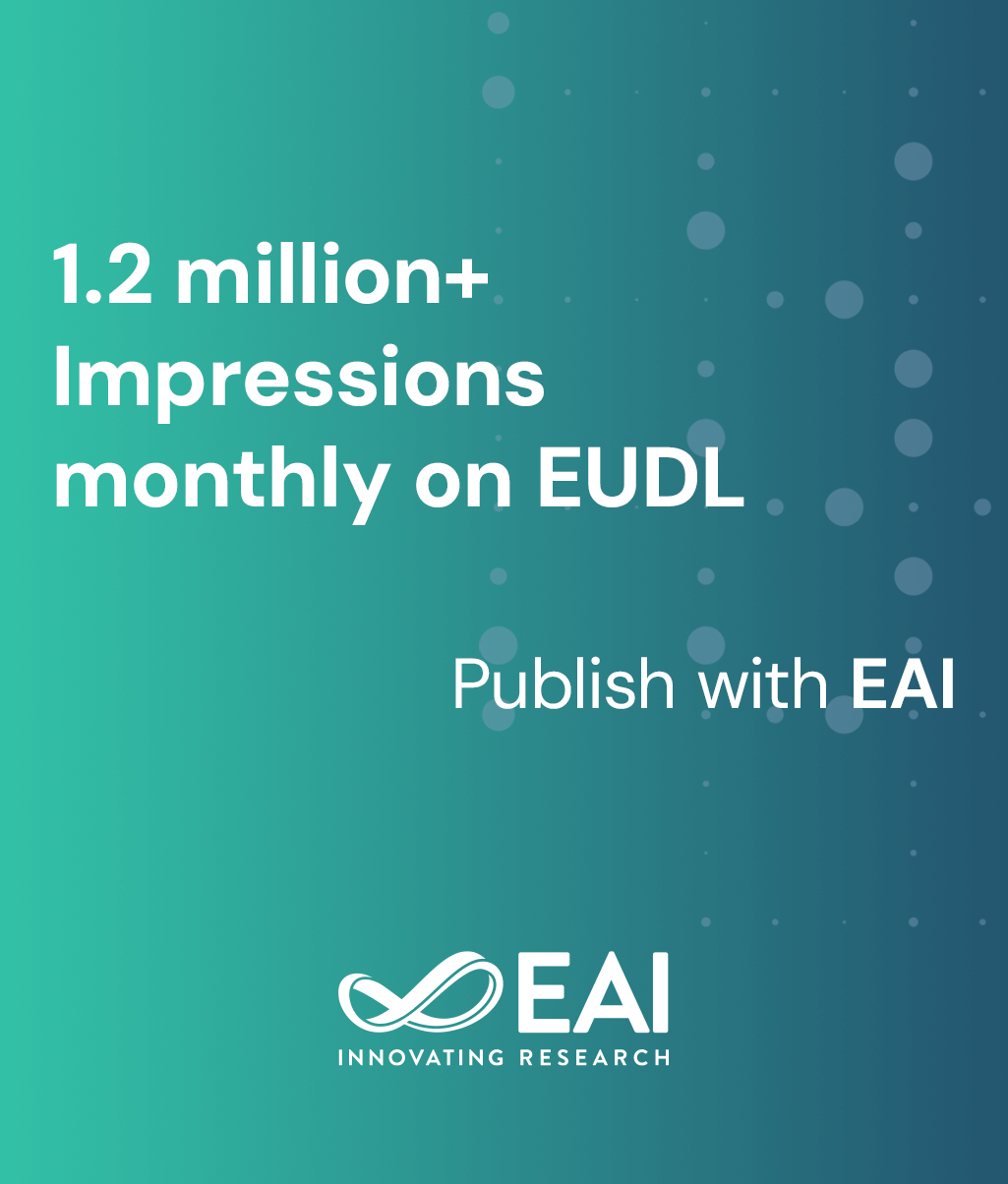
Research Article
Eigenvalue Ratio Detection Based On Exact Moments of Smallest and Largest Eigenvalues
@INPROCEEDINGS{10.4108/icst.crowncom.2011.246151, author={Muhammad Shakir and Wuchen Tang and Anlei Rao and Muhammad Imran and Mohamed Alouini}, title={Eigenvalue Ratio Detection Based On Exact Moments of Smallest and Largest Eigenvalues}, proceedings={6th International ICST Conference on Cognitive Radio Oriented Wireless Networks and Communications}, publisher={IEEE}, proceedings_a={CROWNCOM}, year={2012}, month={5}, keywords={spectrum sensing; eigenvalue ratio based detection; non-asymptotic gaussian approximation; copula}, doi={10.4108/icst.crowncom.2011.246151} }
- Muhammad Shakir
Wuchen Tang
Anlei Rao
Muhammad Imran
Mohamed Alouini
Year: 2012
Eigenvalue Ratio Detection Based On Exact Moments of Smallest and Largest Eigenvalues
CROWNCOM
IEEE
DOI: 10.4108/icst.crowncom.2011.246151
Abstract
Detection based on eigenvalues of received signal covariance matrix is currently one of the most effective solution for spectrum sensing problem in cognitive radios. However, the results of these schemes always depend on asymptotic assumptions since the close-formed expression of exact eigenvalues ratio distribution is exceptionally complex to compute in practice. In this paper, non-asymptotic spectrum sensing approach to approximate the extreme eigenvalues is introduced. In this context, the Gaussian approximation approach based on exact analytical moments of extreme eigenvalues is presented. In this approach, the extreme eigenvalues are considered as dependent Gaussian random variables such that the joint probability density function (PDF) is approximated by bivariate Gaussian distribution function for any number of cooperating secondary users and received samples. In this context, the definition of Copula is cited to analyze the extent of the dependency between the extreme eigenvalues. Later, the decision threshold based on the ratio of dependent Gaussian extreme eigenvalues is derived. The performance analysis of our newly proposed approach is compared with the already published asymptotic Tracy-Widom approximation approach.