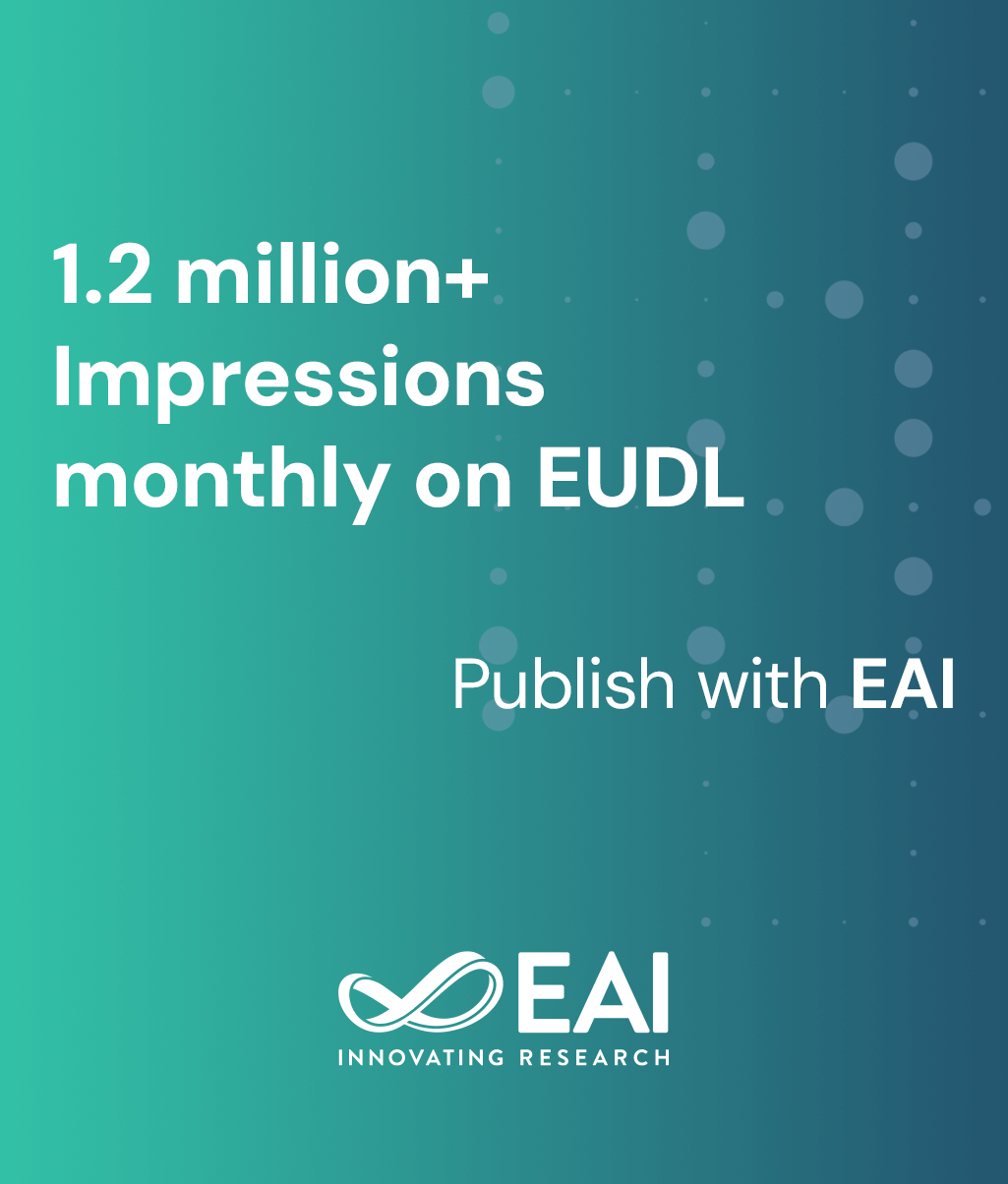
Research Article
Towards Composable Prediction of Contact Groups
@INPROCEEDINGS{10.4108/icst.collaboratecom.2014.257329, author={Andrew Ghobrial and Jacob Bartel and Prasun Dewan}, title={Towards Composable Prediction of Contact Groups}, proceedings={10th IEEE International Conference on Collaborative Computing: Networking, Applications and Worksharing}, publisher={IEEE}, proceedings_a={COLLABORATECOM}, year={2014}, month={11}, keywords={groups contact groups prediction email prediction group prediction contacts equivalence classes recommendations data mining}, doi={10.4108/icst.collaboratecom.2014.257329} }
- Andrew Ghobrial
Jacob Bartel
Prasun Dewan
Year: 2014
Towards Composable Prediction of Contact Groups
COLLABORATECOM
IEEE
DOI: 10.4108/icst.collaboratecom.2014.257329
Abstract
Users’ contacts often need to be grouped into equivalence classes for various purposes such as easily sending a message to all members of the group. Several approaches have been recently developed to make such predictions (a) for both ephemeral and persistent groups (b) in both email and social networks systems. However, no research has attempted to compare these approaches or compose them by using ideas of one in another. We have taken a step in this direction. We have developed and compared multiple approaches to predicting persistent contact groups in email. These approaches compose an algorithm that generates friend lists in Facebook from a social graph with different techniques for generating the social graph. One of these techniques is based on a scoring algorithm used by Google to predict ephemeral groups incrementally. To compare the approaches we ran a user study involving 19 participants and used two simple metrics that calculated the average percentage difference between a predicted group and the group of addresses in a future message. The evaluation showed that using the Google score was the best approach though it offered very small improvements over all but one of the simpler methods.