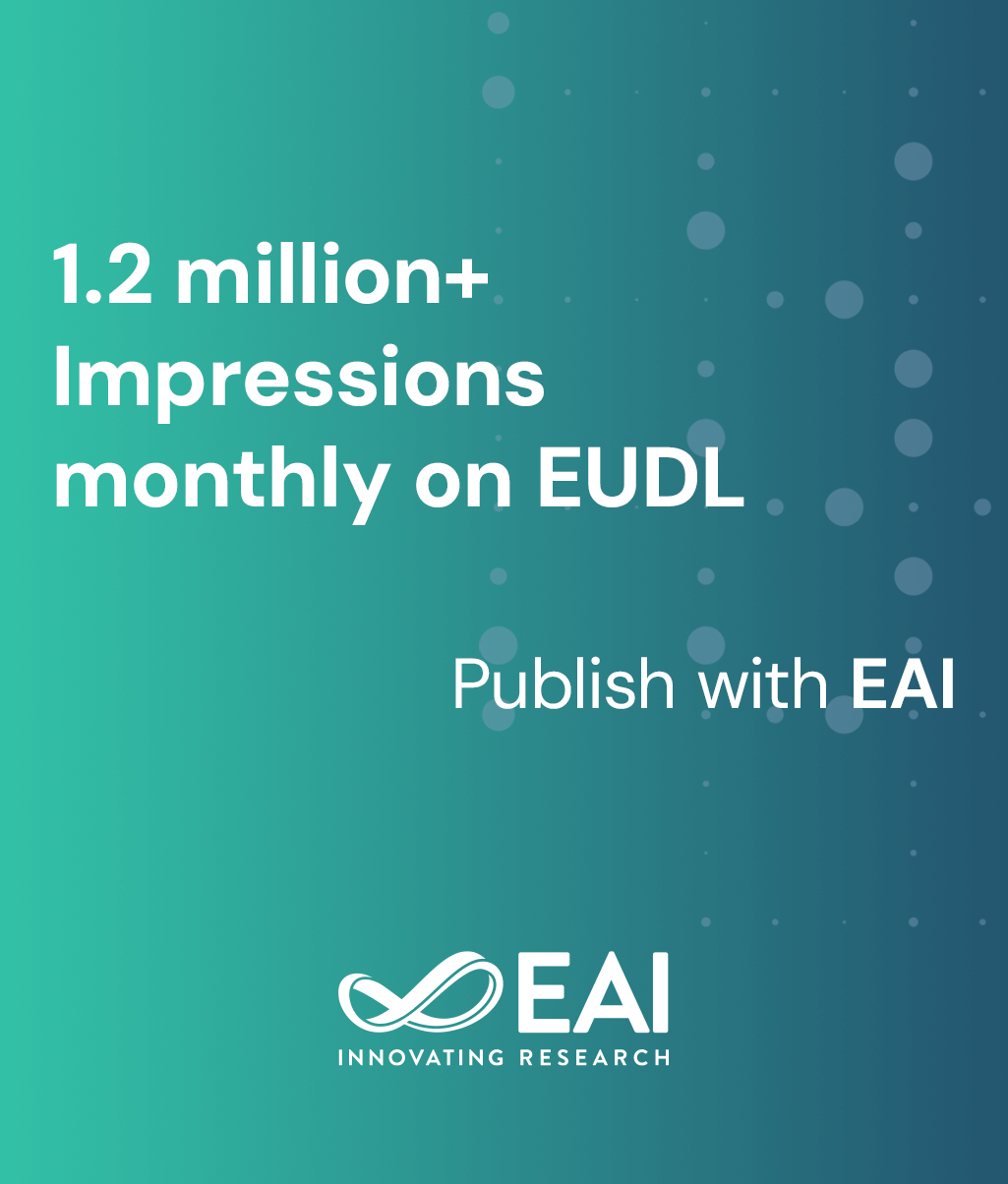
Research Article
Towards Enabling Probabilistic Databases for Participatory Sensing
@INPROCEEDINGS{10.4108/icst.collaboratecom.2014.257239, author={Quoc Viet Hung Nguyen and Saket Sathe and Thang Duong and Karl Aberer}, title={Towards Enabling Probabilistic Databases for Participatory Sensing}, proceedings={10th IEEE International Conference on Collaborative Computing: Networking, Applications and Worksharing}, publisher={IEEE}, proceedings_a={COLLABORATECOM}, year={2014}, month={11}, keywords={participatory sensing trust management probabilistic database}, doi={10.4108/icst.collaboratecom.2014.257239} }
- Quoc Viet Hung Nguyen
Saket Sathe
Thang Duong
Karl Aberer
Year: 2014
Towards Enabling Probabilistic Databases for Participatory Sensing
COLLABORATECOM
IEEE
DOI: 10.4108/icst.collaboratecom.2014.257239
Abstract
Participatory sensing has emerged as a new data collection paradigm, in which humans use their own devices (cell phone accelerometers, cameras, etc.) as sensors. This paradigm enables to collect a huge amount of data from the crowd for world-wide applications, without spending cost to buy dedicated sensors. Despite of this benefit, the data collected from human sensors are inherently uncertain due to no quality guarantee from the participants. Moreover, the participatory sensing data are time series that not only exhibit highly irregular dependencies on time, but also vary from sensor to sensor. To overcome these issues, we study in this paper the problem of creating probabilistic data from given (uncertain) time series collected by participatory sensors. We approach the problem in two steps. In the first step, we generate probabilistic times series from raw time series using a dynamical model from the time series literature. In the second step, we combine probabilistic time series from multiple sensors based on the mutual relationship between the reliability of the sensors and the quality of their data. Through extensive experimentation, we demonstrate the efficiency of our approach on both real data and synthetic data.