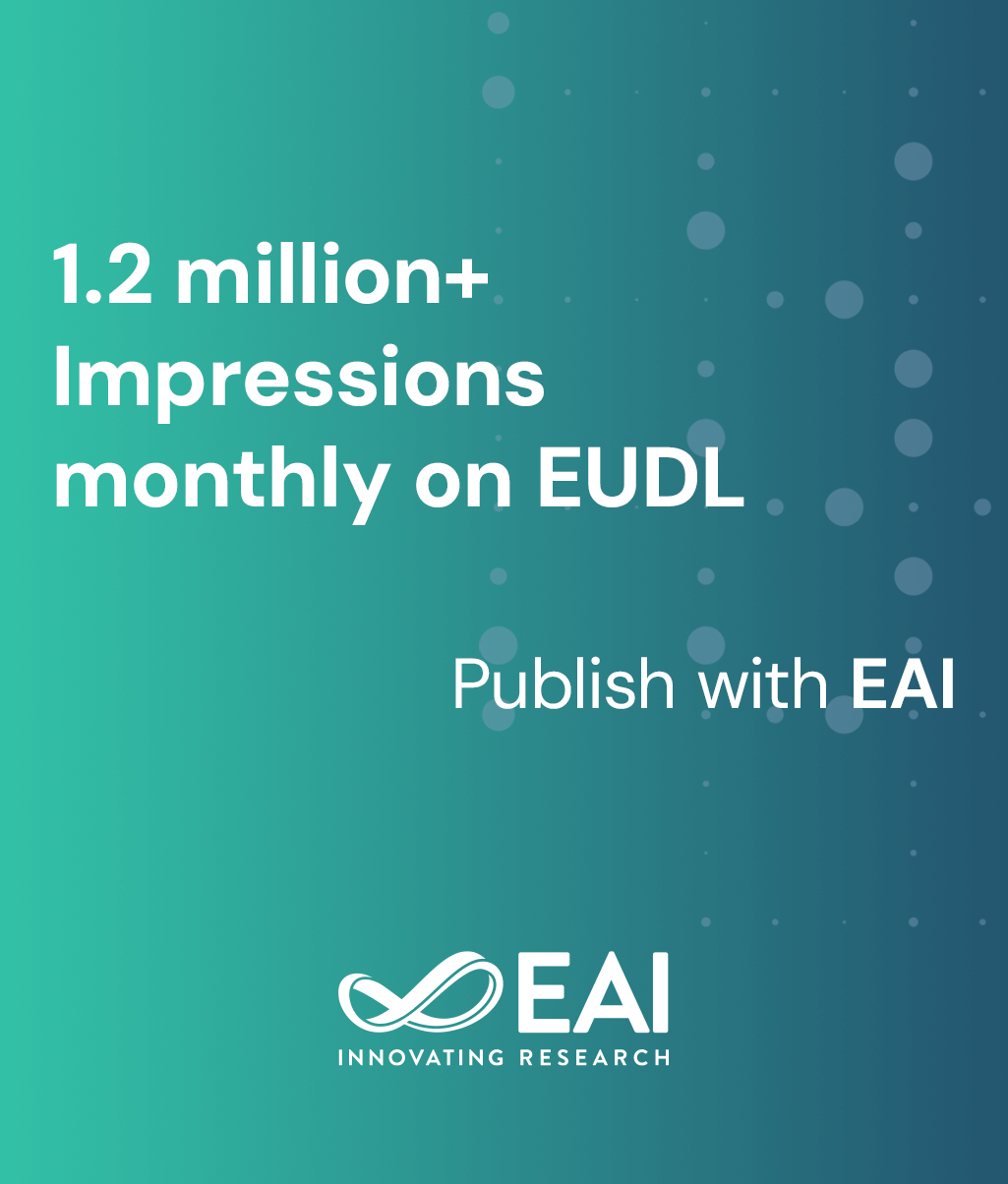
Research Article
Domain Ontology-based Feature Reduction for High Dimensional Drug Data and its Application to 30-Day Heart Failure Readmission Prediction
@INPROCEEDINGS{10.4108/icst.collaboratecom.2013.254124, author={Sisi Lu and Ye Ye and Rich Tsui and Howard Su and Ruhsary Rexit and Sahawut Wesaratchakit and Xiaochu Liu and Rebecca Hwa}, title={Domain Ontology-based Feature Reduction for High Dimensional Drug Data and its Application to 30-Day Heart Failure Readmission Prediction}, proceedings={9th IEEE International Conference on Collaborative Computing: Networking, Applications and Worksharing}, publisher={ICST}, proceedings_a={COLLABORATECOM}, year={2013}, month={11}, keywords={high dimensional data feature reduction feature selection domain ontology heart failure readmission prediction}, doi={10.4108/icst.collaboratecom.2013.254124} }
- Sisi Lu
Ye Ye
Rich Tsui
Howard Su
Ruhsary Rexit
Sahawut Wesaratchakit
Xiaochu Liu
Rebecca Hwa
Year: 2013
Domain Ontology-based Feature Reduction for High Dimensional Drug Data and its Application to 30-Day Heart Failure Readmission Prediction
COLLABORATECOM
IEEE
DOI: 10.4108/icst.collaboratecom.2013.254124
Abstract
High dimensional feature space could potentially hinder the efficiency and performance for machine learning, and high correlations between features may further increase the redundancy and diminish performance of learning algorithms. Domain ontology provides relationships and similarities between concepts in the specific area, and thus can be used to reduce redundancy by clustering concepts and revealing their functionality. In this paper, we study the problem of using high dimensional medication data to predict the probability of 30-Day heart-failure readmission. We propose a feature reduction method for high dimensional dataset using a combination of two drug ontologies. By creating a tree structure of the combination, the method uses a greedy strategy to obtain a subset of features, which may have higher correlation with the class label but lower correlation with each other. Experimental results show that our methods improve the performance of heart-failure readmission prediction (using only drug data) comparing to existing feature reduction methods without drug domain ontologies.