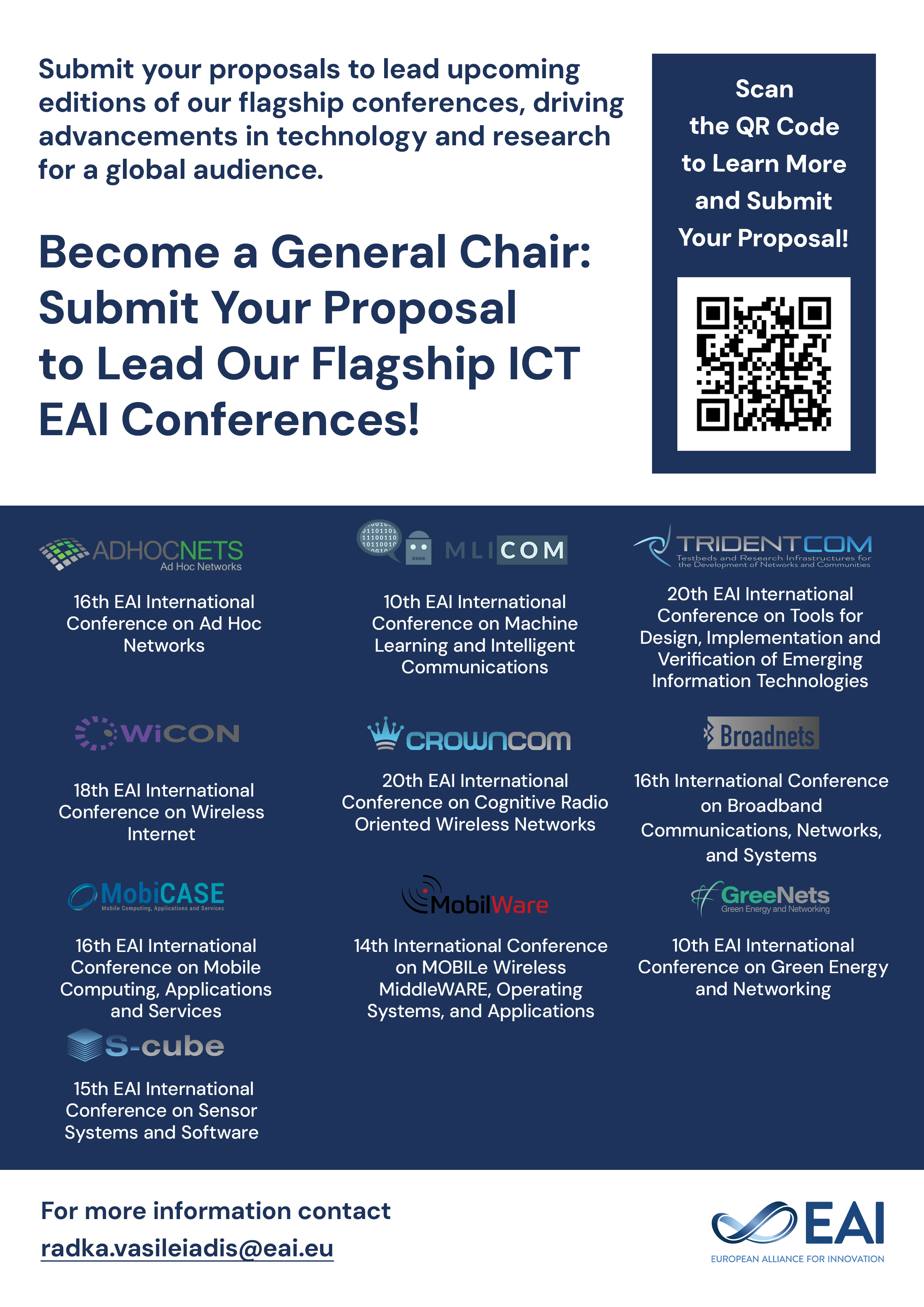
Research Article
Towards Context-aware Recommendations: Strategies for Exploiting Multi-criteria Communities
@INPROCEEDINGS{10.4108/icst.collaboratecom.2013.254105, author={Thuy Ngoc Nguyen and An Te Nguyen}, title={Towards Context-aware Recommendations: Strategies for Exploiting Multi-criteria Communities}, proceedings={9th IEEE International Conference on Collaborative Computing: Networking, Applications and Worksharing}, publisher={ICST}, proceedings_a={COLLABORATECOM}, year={2013}, month={11}, keywords={collaborative filtering context-aware recommender system matrix factorization multi-criteria communities}, doi={10.4108/icst.collaboratecom.2013.254105} }
- Thuy Ngoc Nguyen
An Te Nguyen
Year: 2013
Towards Context-aware Recommendations: Strategies for Exploiting Multi-criteria Communities
COLLABORATECOM
IEEE
DOI: 10.4108/icst.collaboratecom.2013.254105
Abstract
Nowadays, recommender systems are becoming popular since they help users alleviate the information overload problem by offering personalized recommendations. Most systems apply collaborative filtering to predict individual preferences based on opinions of like-minded people through their ratings on items. Recently, context-aware recommender systems (CARSs) exploit additional context data such as time, place and so on for providing better recommendations. However, the large majority of CARSs use only ratings as a criterion for building communities, and ignore other available data allowing users to be grouped into communities. In this paper, we present a novel approach for exploiting multi-criteria communities to generate context-aware recommendations. The underlying idea of three proposed algorithms CRMC, CRESC and CREOC is that for each context, communities from the most suitable criteria followed by the learning phase are incorporated into the recommendation process. Experimental results show that our approach can improve the quality of recommendations.