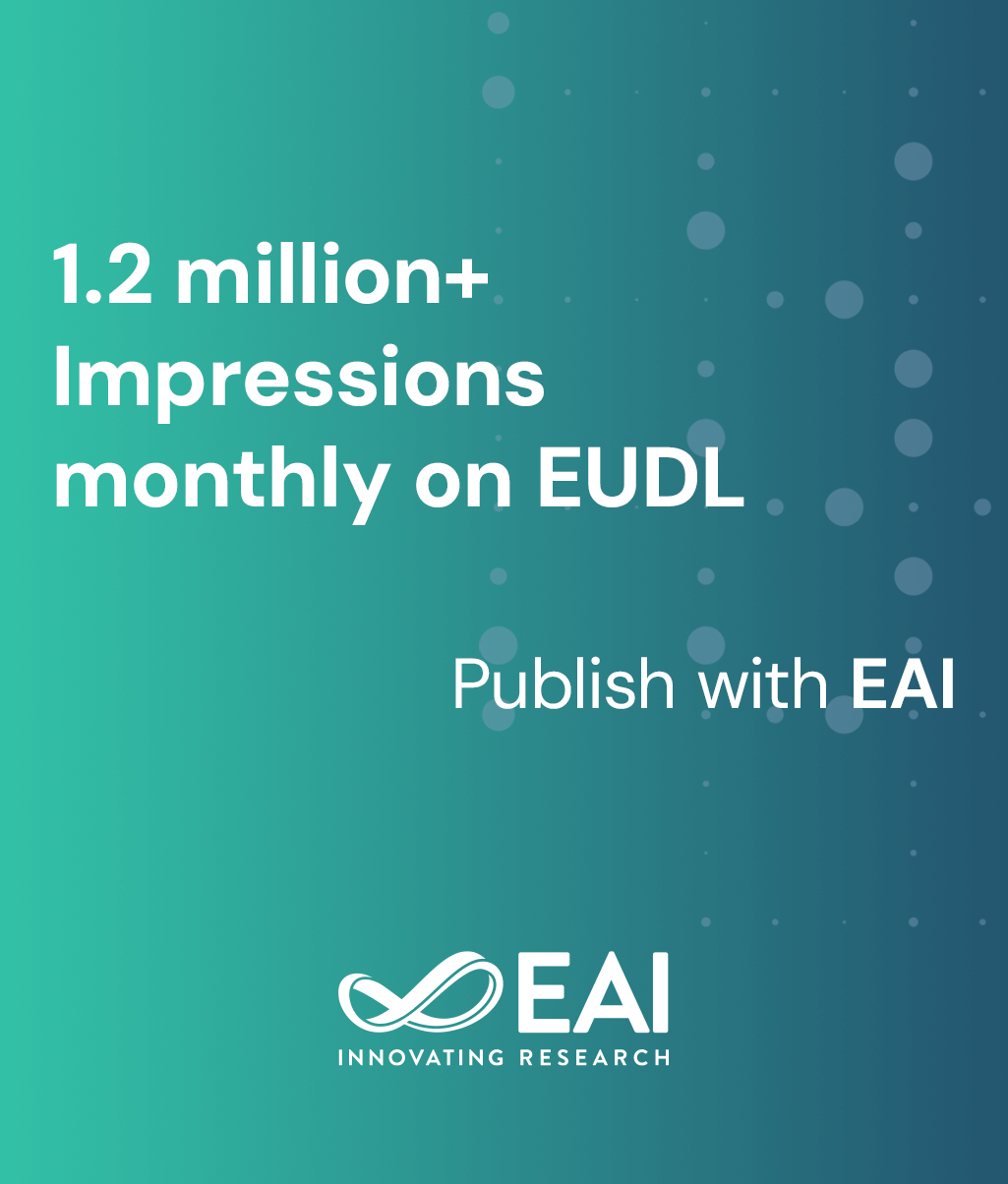
Research Article
Supporting Collaborative Work by Learning Process Models and Patterns from Cases
@INPROCEEDINGS{10.4108/icst.collaboratecom.2013.254071, author={Stefan Sch\o{}nig and Michael Zeising and Stefan Jablonski}, title={Supporting Collaborative Work by Learning Process Models and Patterns from Cases}, proceedings={9th IEEE International Conference on Collaborative Computing: Networking, Applications and Worksharing}, publisher={ICST}, proceedings_a={COLLABORATECOM}, year={2013}, month={11}, keywords={business process management process mining adaptive case management association rule mining process observation}, doi={10.4108/icst.collaboratecom.2013.254071} }
- Stefan Schönig
Michael Zeising
Stefan Jablonski
Year: 2013
Supporting Collaborative Work by Learning Process Models and Patterns from Cases
COLLABORATECOM
IEEE
DOI: 10.4108/icst.collaboratecom.2013.254071
Abstract
Recent work shows an increasing interest of the Business Process Management (BPM) community in unstructured, socalled "human-centric" processes. Case Management (CM) is a new trend that focuses on the support of collaborative human-centric processes. Although CM provides concepts that support human-centric work, processes have to be modelled beforehand in order to be supported by IT systems. Hence, a problem that arises when applying CM is that when organisations begin to formalize CM practice, it is often difficult to express rules controlling the applicability of tasks. Furthermore, fundamental complexity challenges arise when applying CM in practice. In this contribution, we provide a solution to these two issues. We propose that managing human-centric processes should start with model skeletons that serve as a lattice where initial process execution can lean against. Additionally, by tracking different process cases, substantial process knowledge is recorded. Exploring process history might reveal certain recurring patterns that serve as dynamic guidance enhancement for CM systems. In this way, process models might evolve over time, become more and more complete and better reflect operational reality.